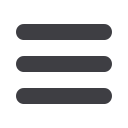

INFORMS Philadelphia – 2015
194
MB65
65-Room 113B, CC
Risk Attitudes in Decision Analysis
Sponsor: Decision Analysis
Sponsored Session
Chair: Andrea Hupman, University of Missouri-St. Louis, 1 University
Blvd, St. Louis, MO, United States of America,
hupman1@illinois.edu1 - Individualized Predictions of Normative Decision Making
Andrea Hupman, University of Missouri-St. Louis,
1 University Blvd, St. Louis, MO, United States of America,
hupman1@illinois.edu, Ali Abbas
Understanding how individuals make decisions in practice and predicting
behavior is important in many practical applications, giving rise to numerous
descriptive models of decision making behavior. In this talk, a behavioral decision
making experiment is described. The results are consistent with a bounded
rationality explanation of decision making behavior in which making accurate
predictions of decisions hinges on individualized information about the decision
maker’s risk attitude.
2 - Marriage and Managers’ Attitudes to Risk
Pavel Savor, Fox School of Business, Temple University,
Philadelphiaa, PA,
pavel.savor@temple.eduWe explore the impact of marriage on corporate CEOs and find that firms run by
single CEOs exhibit higher stock return volatility, pursue more aggressive
investment policies, and do not respond to changes in idiosyncratic risk. These
effects are weaker for older CEOs. Our results continue to hold when we use
variation in divorce laws across states to instrument for marital status, supporting
the hypothesis that marriage itself drives choices rather than it just reflecting
innate heterogeneity.
3 - Using Means Objectives to Present Risk Information
Candice Huynh, Cal Poly - Pomona, CA, United States of
America,
candicehuynh@cpp.edu,Jay Simon
When making decisions involving alternatives with risk, individuals are not
always able to express or view the possible outcomes in terms of a fundamental
objective. To apply information about a means objective correctly, a decision
maker must first translate it into information about a fundamental objective. This
paper presents the results of a study regarding decision makers’ preferences when
information is presented either in terms of a means objective or a fundamental
objective.
4 - Evolving Risk Perceptions of Cybersecurity Events
Heather Rosoff, Research Assistant Professor, University of
Southern California, Price School of Public Policy & CREATE,
3710 McClintock Avenue, Los Angeles, CA, 90089-2902, United
States of America,
rosoff@usc.edu,Robin Dillon-Merrill
Mitigating cyber risks requires understanding how people evaluate risks in this
challenging context and in particular, how they respond to repeated warnings
over time. In the case of the Target credit card breach in 2013, evidence now
shows that Target had failed to follow through on security alerts triggered by the
hacker’s activities. We speculate on why Target’s security team, and why people in
general, might not respond to such alarms in the cyber-security context.
MB66
66-Room 113C, CC
Air Traffic Management Decision Support:
Learning from History
Sponsor: Aviation Applications
Sponsored Session
Chair: Yi Liu, UC Berkeley, 107 McLaughlin Hall, Berkeley, CA, 94720,
United States of America,
liuyi.feier@gmail.com1 - The Identification of Similar Days in the New York Area for Air
Traffic Flow Management Initiatives
Kenneth Kuhn, RAND Corporation, 1776 Main Street, Santa
Monica, CA, 90407, United States of America,
kkuhn@rand.org,
Akhil Shah
Analysis of air traffic flow management initiatives can show the relative success of
decisions, but must account for conditions during planning and operations. We
apply cluster analysis to identify similar days, using features detailing aviation
weather and air traffic around New York. An example is the degree to which jet
route J75 is blocked by convective weather at 9am according to a 7am weather
forecast. Some features are based on automated approaches such as Principal
Component Analysis.
2 - Similar Days? A Story Based on User-defined Similarity
Yi Liu, UC Berkeley, 107 McLaughlin Hall, Berkeley, CA, 94720,
United States of America,
liuyi.feier@gmail.com, Mark Hansen,
Alexey Pozdnukhov
In this work, we propose a supervised data-mining algorithm for measuring
similarity between two days. First, the algorithm trains the distance matrix
between hours according to user-defined similarity and dissimilarity. Then it
calculates the daily distance as a weighted sum of hourly distances. The approach
can be applied to measure similarity between two days post-operation or identify
similar days in the past for a given day.
3 - Representative Traffic Management Initiative Decisions
Alex Estes, University of Maryland-College Park, 3117 AV
Williams, College Park, MD, 20742, United States of America,
aestes@math.umd.edu, Michael Ball, David Lovell
We provide a method for presenting data on traffic management initiatives so that
it may more easily be interpreted by researchers or by TMI decision makers. This
method involves solving a dominating set problem to produce a set of TMIs which
are representative of the range of TMI decisions that have been taken in the past.
MB67
67-Room 201A, CC
The Role of Information in Transportation Models
Sponsor: TSL/Freight Transportation & Logistics
Sponsored Session
Chair: Dirk Mattfeld, Germany,
d.mattfeld@tu-braunschweig.de1 - Approximate Dynamic Programming for Temporal-spatial
Anticipation and Routing of Service Requests
Justin Goodson, Saint Louis University, St. Louis, MO, United
States of America,
goodson@slu.edu, Marlin Ulmer, Dirk Mattfeld
We consider the problem of dynamically routing a vehicle to respond to service
requests arriving randomly over a given time horizon. Building on a value-
function approximation (VFA) that estimates rewards-to-go via the temporal
components of the state variable, we use rollout algorithms to explicitly consider
spatial dimensions. Our method improves upon the VFA scheme in isolation and
points to the potential benefit of using two different approximate dynamic
programming techniques in tandem.
2 - A Look-ahead Solution Framework for the Dynamic Vehicle
Routing Problem
Han Zou, University of Southern California, Los Angeles, CA
United States of America,
hanzou@usc.edu,Maged Dessouky
The problem concerns routing a fleet of capacitated vehicles in real time to fulfill
orders placed by a known set of customers. Some of the orders are known a
priori, while the rest are placed in real time. We develop a look-ahead solution
framework that periodically re-optimizes current vehicle routes by using both
realized and forecasted information. With fine-tuned parameter settings, our
approach has the potential to outperform both a priori routing and total dynamic
dispatching schemes.
3 - Optimal Information Collection in the Vehicle Allocation Problem
in Mega-Cities
Yixiao Huang, Tsinghua University, China,
huangyx12@mails.tsinghua.edu.cn, Warren Powell, Ilya Ryzhov,
Lei Zhao
In mega-cities, urban freight delivery companies serve customers spread in a large
area. These companies typically divide the city into several regions and allocate
the delivery vehicles to serve these regions. The precise cost evaluation of a
vehicle allocation decision can be very expensive, due to the volume and
uncertainty of the delivery demand. We study on, given a fixed information
collection budget, how to collect the cost evaluation information (possibly
through real-life practice) to gain the best knowledge to allocate the vehicles
optimally.
4 - Data-Driven Vehicle Routing with Profits
Dauwe Vercamer, Ghent University, Ghent, Belgium,
Dauwe.Vercamer@ugent.be,, Dirk Van Den Poel,
Michel Gendreau, Philippe Baecke
In sales teams, making optimal visits is crucial. Customer Profitability models help
in identifying top customers, but do not consider the associated visit costs. Vehicle
Routing models make efficient schedules, but use naive estimates rather than
good forecasts. Our prescriptive analytics approach uses auxiliary data and
statistical learning to approximate full-information vehicle routes. The results
show this approach improves customer selection in vehicle routes and maximizes
profitability.
MB65