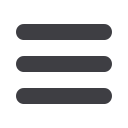

INFORMS Philadelphia – 2015
245
3 - A Low-cost Method for Multiple Disease Prediction
Mohsen Bayati, Assistant Professor, Stanford Graduate School of
Business, 655 Knight Way, Stanford, CA,
United States of America,
bayati@stanford.edu,
Andrea Montanari, Sonia Bhaskar
Recently, in response to the rising costs of healthcare, companies have been
investing in programs to improve the health of their workforce. These programs
aim to reduce the incidence of chronic illnesses and require a low-cost screening
to detect individuals with a high risk of developing such diseases. We offer a
multiple disease prediction procedure that maximizes the predictive power while
minimizes the screening cost. Our method is based on multi-task learning from
machine learning.
MD42
42-Room 102B, CC
Joint Session MSOM-Health/HAS: Operations
Research/Management for Public Health:
Data-Driven and Dynamic Decision-Making
Sponsor: Manufacturing & Service Oper
Mgmt/Healthcare Operations
Sponsored Session
Chair: Soroush Saghafian, Harvard University, 79 JFK Street,
Cambridge, MA, 02138, United States of America,
Soroush.Saghafian@asu.edu1 - New Data-driven Approach to Safety and Risk
Management in ICUs
Retsef Levi, J. Spencer Standish (1945) Professor of Operations
Management, Sloan School of Management, MIT, 100 Main
Street, BDG E62-562, Cambridge, MA, 02142, United States of
America,
retsef@mit.edu,Patricia Folcarelli, Yiqun Hu,
Jeffrey Adam Traina, Daniel Talmor
We develop an innovative system approach to safety in ICUs. The approach is
based on the innovative concept of risk drivers, which are states of the ICU, its
environment and its staff that affect the likelihood of harms, as well as an
innovative aggregated measure of the ‘burden of harm’. Using real data we
develop statistical models that identify risky states in the ICUs of a major
academic medical center.
2 - Developing Optimal Biomarker-Based Prostate Cancer
Screening Policies
Christine Barnett, University of Michigan, 1205 Beal Ave.,
Ann Arbor, MI, United States of America,
clbarnet@umich.edu,Brian Denton, James Montie
Recent advances in the development of new biomarker tests, which physicians
use for the early detection of cancer, have the potential to improve patient
survival by catching cancer at an early stage. We describe a partially observable
Markov decision process (POMDP) to compute near optimal prostate cancer
screening strategies. We present results based on Monte Carlo simulation to
compare the policies developed using our approximated POMDP methods with
those recommended in the medical literature.
3 - Optimizing Hepatitis C Screening and Treatment
Allocation Strategy
Yuankun Li, University of Washington, Seattle, WA,
United States of America,
yuankunl@uw.edu,Zelda Zabinsky,
Hao Huang, Shan Liu
Chronic hepatitis C (HCV) is a significant public health problem affecting 2.7-3.9
million Americans. The U.S. healthcare systems are ramping up combined HCV
screening and treatment efforts, but screening and treatment programs are very
costly. We design the optimal HCV screening and treatment allocation strategies in
the next 10 years under yearly budget constraint from a national perspective. The
method includes simulation optimization using adaptive probabilistic branch and
bound.
4 - A Robust POMDP Framework for the Management of
Post-transplant Medications
Alireza Boloori, PhD Student Of Industrial Engineering, Arizona
State University, 699 S Mill Avenue, Office # 313, Tempe, AZ,
85282, United States of America,
aboloori@asu.edu,
Curtiss B. Cook, Soroush Saghafian, Harini A. Chakkera
Patients after organ transplantations receive high dosages of immunosuppressive
drugs (e.g., tacrolimus) to reduce the risk of organ rejection. However, this
practice has been shown to increase the risk of New-Onset Diabetes After
Transplantation (NODAT). We propose a robust POMDP framework to generate
effective medication management strategies for tacrolimus and insulin. Our
approach increases the patient’s quality of life while reducing the effect of
transition probability estimation errors.
MD43
43-Room 103A, CC
Empirical Revenue Management
Sponsor: Revenue Management and Pricing
Sponsored Session
Chair: Dan Zhang, University of Colorado at Boulder, 995 Regent Dr,
Boulder, United States of America,
Dan.Zhang@colorado.edu1 - Would You Like to Upgrade to a Premium Room? An Empirical
Analysis on Standby Upgrades
Ovunc Yilmaz, PhD Student, University of South Carolina,
1014 Greene St, Columbia, SC, 29208, United States of America,
oyilmaz@email.sc.edu, Mark Ferguson, Pelin Pekgun
Standby upgrades, where the guest is only charged if the upgrade is available at
the time of arrival, is one technique that has become increasingly popular in the
hotel industry. Working on a data set from a major hotel chain, we analyze the
linkage between guest attributes, hotel characteristics and guest decision-making
for standby upgrades through an empirical study.
2 - Analytics for an Online Retailer – Demand Forecasting and Price
Optimization at Rue La La
Kris Johnson Ferreira, Harvard Business School, Morgan Hall
492, Boston, MA, 02163, United States of America,
kferreira@hbs.edu,David Simchi-levi, Bin Hong Alex Lee
We present our work with Rue La La, an online retailer who offers limited-time
discounts on designer apparel. One of their main challenges is revenue
management for new products. We use machine learning to build a demand
prediction model, the structure of which poses challenges on creating a pricing
policy. We develop theory around multi-product price optimization and use this
to create and implement a pricing decision support tool. Field experiment results
show significant increases in revenue.
3 - A Model to Estimate Individual Preferences using Panel Data
Gustavo Vulcano, NYU, 44 West Fourth St, Suite 8-76, New York,
NY, 10012, United States of America,
gvulcano@stern.nyu.edu,Srikanth Jagabathula
In a retail operation, customer choices may be affected by stockout and
promotion events. Given panel data with the transaction history of each
customer, we use a general nonparametric framework in which we represent
customers by partial orders of preferences. Numerical experiments on real-world
panel data show that our approach allows more accurate, fine-grained predictions
for individual purchase behavior compared to state-of-the-art existing methods.
4 - Estimation of Arrival Rates and Choice Model with Censored Data
Anton Kleywegt, Georgia Tech, 755 Ferst Drive NW, Atlanta, GA,
30332, United States of America,
anton@isye.gatech.eduRevenue management models with customer choice behavior include two types
of parameters: (1) customer arrival rates and (2) choice parameters. Revenue
managers usually have censored arrival data only, because no-purchase data are
not included. For both homogenous and nonhomogeneous Poisson arrivals we
give necessary and sufficient conditions for the arrival rates and choice
parameters to be identifiable with such censored data, and we give algorithms for
parameter estimation, with numerical results with airline data
MD44
44-Room 103B, CC
Pricing and Information in Innovative
Business Models
Sponsor: Revenue Management and Pricing
Sponsored Session
Chair: Jose Guajardo, University of California Berkeley,
545 Student Services Bldg #1900, Berkeley, CA, 94720-1900,
United States of America,
jguajardo@berkeley.edu1 - Information Provision Policies in Developing Countries:
Heterogeneous Farmers and Market Selection
Chen-Nan Liao, National Taiwan University, No.1,Sec. 4,
Roosevelt Rd., Taipei City, Taiwan - ROC,
chennan@berkeley.edu,Ying-ju Chen, Chris Tang
We examine the impact of information provision policies on farmer welfare in
developing countries where heterogeneous farmers lack relevant information for
making market (or crop) selection. We show that the optimal information
provision policy may call for limited dissemination, and the government can
implement it while overcoming perceived unfairness by providing information to
all farmers at a nominal fee. We also examine issues including information
dissemination via a for-profit company.
MD44