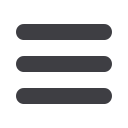

INFORMS Philadelphia – 2015
384
2 - Marketing-driven Content Management in Large Organizations
Roman Vaculin, IBM, 1101 Kitchawan Rd, Yorktown Heights, NY,
United States of America,
vaculin@us.ibm.com, Yi-min Chee,
Ashish Jagmohan, Elham Khabiri, Richard Segal, Noi Sukaviriya
Content management in large organizations is a complex process involving range
of approaches such as text analysis or classification with taxonomies. We focus on
facilitating management of legacy and creation of new content to improve
marketing content effectiveness. We discuss techniques for (1) content
characterization based on semantic concept models, (2) mapping textual content
to legacy taxonomies, and (3) taxonomy enhancement for data-driven taxonomy
rationalization, extension and mapping.
3 - Product Recommendations with Discovered Hidden Topics in
Online Reviews
Julie Zhang, Assistant Professor, University of Massachusetts
Lowell, One University Ave., Lowell, MA, 01854,
United States of America,
juheng_zhang@uml.eduWe use LDA (latent Dirichlet Allocation) to discover hidden information
embedded in online product reviews for consumers to make informed purchase
decisions. The discovered topics are used to make product recommendations to
offer consumers better utility.
4 - Towards Automatic Identification of B2B Marketing Prospects
Elham Khabiri, IBM, 1101 Kitchawan RD, Yorktown Heights, NY,
United States of America,
ekhabiri@us.ibm.com,Roman Vaculin,
Richard Hull, Matthew D. Riemer
In B2B marketing, it is critical to identify marketing leads effectively. We
investigate into what extent the B2B leads identification process can be
automated using online and historical data, and if algorithmic leads mining can
achieve better performance than leads identified by human experts. We present a
solution that combines deep learning to identify look-a-like companies with
respect to historical leads, and mining of online data for identification of signals
indicating potential leads.
WA33
33-Room 410, Marriott
Data-driven Healthcare Operations
Sponsor: Health Applications
Sponsored Session
Chair: Muge Capan, Value Institue, Christiana Care Health System,
4755 Ogletown-Stanton Road, John H. Ammon Medical Education
Center, Newark, DE, 19718, United States of America,
Muge.Capan@ChristianaCare.org1 - Determining an Optimal Schedule for Pre-mixing
Chemotherapy Drugs
Donald Richardson, University of Michigan, Ann Arbor, MI,
donalric@umich.edu, Amy Cohn
In collaboration with the University of Michigan Comprehensive Cancer Center,
we have developed a data-driven, optimization-based approach to improving the
timeliness of drug preparation for chemotherapy infusion patients while reducing
staff workload and improving resource utilization. We compare the results from
both static and dynamic decision policies to determine the optimal schedule for
pre-mixing chemotherapy drugs at the cancer center.
2 - Using Text Analytics to Identify Labor and Delivery Sentiment
from the Internet and Social Media
Karen Hicklin, PhD Student, North Carolina State University, 111
Lampe Drive, Campus Box 7906, Raleigh, NC, 27695, United
States of America,
kthickli@ncsu.edu, Julie Ivy, Fay Cobb Payton,
Evan Myers, Meera Viswanathan, Michael Wallis,
Vidyadhar Kulkarni
Pregnant women often seek counsel online from doctors, midwives, experienced
and other expectant mothers to understand if their experience lines up with that
of others and to seek opinions, suggestions and recommendations. We use text
analytics to identify key attributes and preferences for women from internet data
to identify important attributes that influence patient perceptions in regards to
the birth experience that can be used as input parameters to inform delivery
mode decision models.
3 - Data-driven and Analytical Approaches to Falls Injury Prediction
and Rescue Resources Allocation
Tze Chiam, Associate Director, Research Informatics, Christiana
Care Health Systems, 4755 Ogletown-Stanton Road,
Newark, DE, 19718, United States of America,
Tze.C.Chiam@ChristianaCare.org,Kristen Miller,
Bailey Ingraham Lopresto
As an effort to improve patient safety, Christiana Care Health Systems embarked
on work to evaluate current rescue strategies for patient fall events. This study
investigates the use of age, bone density, coagulation, surgery and fall type to
identify patients at high risk for injury due to falls and the appropriate responses
based on these criteria. A discrete-event simulation is used to evaluate rescue
strategies that yield fastest response time while minimizing interruptions to the
system.
4 - Nurse Scheduling Optimization in the Neonatal Intensive
Care Unit
Muge Capan, Value Institue, Christiana Care Health System, 4755
Ogletown-Stanton Road, John H. Ammon Medical Education
Center, Newark, DE, 19718, United States of America,
Muge.Capan@ChristianaCare.org,Eric V. Jackson, Robert Locke
Nurse scheduling is the process of assigning nurses to work shifts. A suboptimal
schedule can impact the staffing ratios, nurses’ well-being, job satisfaction, and
quality of care. Nurse scheduling in the Neonatal Intensive Care Unit is
particularly challenging due to the complexity of care environment and required
nursing skillset. We present a multi-objective optimization approach to allocate
nurses to shifts while considering institutional requirements, workload fairness
and nurses’ health.
WA34
34-Room 411, Marriott
Real World Problems, Innovative Approaches and
Implementations at Geisinger Health System
Sponsor: Health Applications
Sponsored Session
Chair: Christopher Stromblad, Senior Modeler - Operations Research,
Geisinger Health System, 100 N. Academy Ave, Danville, PA, 17822,
United States of America,
ctstromblad@geisinger.edu1 - Implementing Predictive Models in Five Operating Suites
Improved Surgical Case Duration Accuracy
Ronald Dravenstott, Geisinger Center for Healthcare Systems
Engineering, 100 N Academy Ave, Danville, PA, 17822, United
States of America,
rwdravenstott@geisinger.edu, Eric Reich,
Cheng-bang Chen, Priyantha Devapriya
The length of a surgical procedure is generally predicted using a moving average
of previous procedures performed by a given surgeon, a method which is often
inaccurate and complicates Operating Room scheduling. This research developed
and implemented predictive models for high-volume surgeries to seamlessly
improve surgical scheduling by leveraging patient and provider data. The models
now in place overcame real-time data inconsistencies and have improved
scheduling.
2 - Anticipating Provider Orders in Outpatient Clinics
Yi-shan Sung, Research Assistant, Penn State, 425 Waupelani Dr.,
Apt. 509, State College, PA, 16801, United States of America,
yqs5097@psu.edu, Ronald Dravenstott, Priyantha Devapriya,
Soundar Kumara
Understanding provider ordering patterns for patients can enhance resource
utilization in hospitals. By using patient and provider retrospective data, this
research aims to develop statistical and network-based techniques to predict
orders for upcoming appointments. These predictions can lead to a
recommendation system supporting providers to increase order effectiveness and
ease order documentation. The models will be validated with outpatient data
from pulmonary clinics.
3 - RTLS Data Applications in Healthcare Analytics and Modeling
Seth Hostetler, Lead Process Engineer, Geisinger Health System,
100 N Academy Ave, Danville, PA, 17822-2550,
United States of America,
sthostetler@geisinger.eduOften, when developing models in healthcare, the lack of accurate and complete
data is cited as a limitation. A real-time location system is able provide additional
information concerning patient, staff, and asset locations and flows. This
presentation will present results from applications in three application areas
(ambulatory, inpatient, and emergency department) where RTLS data has been
utilized to provide additional insight via analytic methods and improved modeling
capabilities.
4 - Outpatient Clinic Provider Scheduling under Uncertainty
Deepak Agrawal, PhD Candidate, Pennsylvania State University,
801 Southgate Drive, A4, State College, PA, 16801, United States
of America,
dua143@psu.edu, Christopher Stromblad,
Soundar Kumara, Mort Webster
Current clinic provider scheduling systems are inefficient, because scheduling is
done manually and months in advance, most of the demand, capacity, and other
constraints and their uncertainties are not considered. There is no objective way
to evaluate the quality of a schedule; until after the fact. Therefore, the original
schedule becomes suboptimal at the time of realization. A stochastic program to
provider scheduling is proposed considering demand and capacity uncertainties
using real data.
WA33