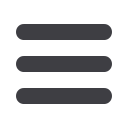

INFORMS Nashville – 2016
109
3 - Path-dependent And Randomized Strategies In Barberis’ Casino
Gambling Model
Xunyu Zhou, Columbia University, Manhattan, New York City, NY,
United States,
xz2574@columbia.eduWe consider the dynamic casino gambling model initially proposed by Barberis
(2012) and study the optimal stopping strategy of a pre-committing gambler with
cumulative prospect theory (CPT) preferences. We illustrate how the strategies
computed in Barberis (2012) can be strictly improved by reviewing the betting
history or by tossing an independent coin, and we explain that the improvement
generated by using randomized strategies results from the lack of quasi-convexity
of CPT preferences. Moreover, we show that any path-dependent strategy is
equivalent to a randomization of path-independent strategies.
4 - Optimal Exit Time From Casino Gambling: Strategies Of
Pre-committed And Naive Gamblers
Xuedong He, Chinese University of Hong Kong,
xdhe@se.cuhk.edu.hkWe study the strategies of a pre-committed gambler, who commits her future
selves to the strategy she sets up today, and of a naive gambler, who fails to do so
and thus keeps changing plans at every time. We identify conditions under which
the pre-committed gambler, asymptotically, adopts a stop-loss strategy, exhibits
the behavior of disposition effect, or does not exit. When the utility function is
piece-wise power and the probability weighting functions are concave power, we
derive the optimal strategy of the pre-committed gambler in closed-form
whenever it exists. Finally, we study the actual behavior of the naive gambler and
highlight its marked differences from that of the pre-committed gambler.
SD46
209B-MCC
Revenue Management: From Theory to Practice
Sponsored: Revenue Management & Pricing
Sponsored Session
Chair: Georgia Perakis, Massachusetts Institute of Technology,
Cambridge, MA, United States,
georgiap@mit.edu1 - The Role Of Vendor Funds In Promotion Planning
Lennart Baardman, MIT, Cambridge, MA, United States,
Georgia Perakis, Kiran Venkata Panchamgam
Vendor funds are integral to promotion planning. Vendor funds are trade deals in
which manufacturers offer discounts to retailers, encouraging them to promote
their products, while demanding pass-through to the customers. We model the
problem of selecting vendor funds to maximize profit while taking into account
the impact on promotional pricing as a QIP. First, we use a strategic model to
analyze the optimal strategies of both the manufacturer and the retailer.
Additionally, to solve the tactical problem, we use Lagrangian relaxation to
propose an approximation algorithm with analytical performance guarantees.
Finally, computational results show near-optimal practical performance.
2 - Revenue Management For The Shipping Industry Under
Limited Foresight
Max Biggs, Massachusetts Institute of Technology, Cambridge, MA,
United States,
maxbiggs@mit.edu, Georgia Perakis
We study a scenario where a shipping company has limited foresight into cargo
availability in future periods. It is possible that a high valued cargo may discharge
in unpromising region with scarce or low valued cargoes, thus jeopardizing profit
in subsequent time periods. We model this situation using a finite horizon
Markov Decision Process and present a ranking algorithm which solves optimally
in polynomial time. We also explore fast heuristics, and test these algorithms on a
simulation that uses real shipping data to evaluate their performance in a practical
setting. We also show extensions for uncertain shipping rates.
3 - Submodular Batch Scheduling
Daniel Chen, MIT, Cambridge, MA, United States,
dcchen@mit.edu, Retsef Levi, Georgia Perakis
Consider an online retailer shipping out orders from a warehouse. Multi-item
orders take up capacity in a holding area until all items are picked. This holding
area is often the cause of bottlenecks, so we formulate the submodular batch
scheduling problem to maximize throughput. We wish to schedule jobs in batches
to minimize the sum of job completion times. The processing time of each batch is
given by a submodular cost function, and the completion time of each job is given
by the completion time of the batch. We show this problem is strongly NP-hard,
but propose several practical methods, including a 4-approximation algorithm.
4 - The Periodic Joint Replenishment Problem Is Strongly NP-hard
Tamar Cohen, MIT,
tcohen@mit.edu,Liron Yedidsion
The Joint Replenishment Problem (JRP) deals with the prospect of saving
resources through coordinated replenishments in order to achieve substantial cost
savings. In the JRP it is required to schedule the replenishment times of
numerous commodities in order to supply a constant demand per commodity. In
this research we answer a long-standing open question regarding the
computational complexity the periodic JRP. This problem received a lot of
attention over the years and many heuristic and approximation algorithms were
suggested. However, in spite of the vast effort, the complexity of the problem
remained unresolved. In this research, we provide a proof that the problem is
indeed strongly NP-hard.
SD47
209C-MCC
Online Learning and Revenue Management
Sponsored: Revenue Management & Pricing
Sponsored Session
Chair: Maxime Cohen, Google Research, Google, New York, NY, 10012,
United States,
maxccohen@google.comCo-Chair: Ilan Lobel, New York University, New York, NY, United
States,
ilobel@stern.nyu.edu1 - Dynamic Pricing And Learning With Online Retail Rankings
N. Bora Keskin, Duke University, Durham, NC, United States,
bora.keskin@duke.edu,Arnoud V. den Boer
In online market environments such as Amazon or Google Shopping, firms
receive advertisement space if they satisfy certain conditions. Beforehand, it is not
clear if the benefits of this increased exposure outweigh the potential costs. We
investigate this question in a dynamic pricing-and-learning setting.
2 - Dynamic Pricing With Demand Covariates
Sheng Qiang, Stanford University,
sqiang@stanford.edu,Mohsen Bayati
We consider a generic problem that a firm sells products over T periods without
knowing the demand function. The firm sets prices to earn revenue and learn the
demand function. In each period before setting the prices, the firm observes some
demand covariates, which may be predictive of the demand. Demand covariates
can include marketing expenditure, consumer’s attributes, weather, etc. We prove
that in this setting the greedy policy achieves asymptotically optimal performance.
We also show that inclusion of any set of demand covariates as potential variables
for the demand estimation (even though they could be independent of the
demand) would make greedy policy asymptotically optimal.
3 - Optimistic Gittins Indices
Vivek Farias, MIT,
vivekf@mit.edu,Eli Gutin
We consider the multi-armed bandit problem in the Bayesian setting wherein one
seeks to minimize expected regret. Gittins indices provide an optimal algorithm
for the maximization of discounted, infinite horizon rewards (as opposed to regret
minimization) in this very setting. So motivated, we propose an index rule we
dub ‘optimistic’ Gittins indices. We show that this rule achieves logarithmic regret
with an optimal constant, matching the Lai-Robbins lower bound for the problem
— the strongest possible guarantee possible. The algorithm also offers excellent
performance relative to recently studied approaches such as Thomspon and
Information Directed Sampling.
4 - Feature-based Dynamic Pricing
Ilan Lobel, NYU Stern, New York, NY, 10012, United States,
ilobel@stern.nyu.edu,Maxime Cohen, Renato Paes Leme
We consider the problem faced by a firm that receives highly differentiated
products in an online fashion and needs to price them in order to sell them to its
customer base. Products are described by vectors of features and the market value
of each product is linear in the values of the features. The firm does not initially
know the values of the different features, but it can learn the values of the
features based on whether products were sold at the posted prices in the past. We
propose an algorithm that combines ideas from contextual bandits and the
ellipsoid method, and show that it has a worst-case regret that is quadratic in the
dimensionality of the feature space and logarithmic in the time horizon.
SD47