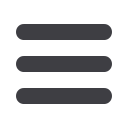

INFORMS Nashville – 2016
213
2 - Aggregate Variation Under Network Perturbation
Azarakhsh Malekian, Rotman School of management,
azarakhsh.malekian@rotman.utoronto.caWe consider a game among agents represented by nodes of a graph in which the
utility of each agent depends on the externalities from his neighbors. We study
the sensitivity of equilibrium actions of agents with respect to the strengths of the
network structure and its connections in both random and deterministic settings.
3 - Diffusion Disparities In Competitive Settings
Yotam Shmargad, School of Information, University of Arizona,
yotam@email.arizona.eduIn many settings, competing groups have different resources at their disposal,
resulting in imbalances in their abilities to seed messages. We conduct an
experimental study to investigate the effect of network structure on diffusion in a
setting with asymmetric seeding. We manipulate network structure by randomly
assigning participants to social networks that vary in clustering (i.e. the extent to
which individuals’ ties are connected to each other). We then seed competing
messages in these networks and model their diffusion over the course of eight
days. We find that clustering perpetuates the disparities in exposure to
asymmetrically-seeded messages.
MD16
105A-MCC
Distributionally Robust Optimization
Sponsored: Optimization, Optimization Under Uncertainty
Sponsored Session
Chair: Angelos Georghiou, Swiss Federal Institute of Technology,
Zurich, Zurich, 00000, Switzerland,
angelosg@control.ee.ethz.chCo-Chair: Grani Adiwena Hanasusanto, University of Texas at Austin,
Austin, TX, 1015, United States,
grani.hanasusanto@utexas.edu1 - Robust Control With Adjustable Uncertainty Sets: Providing
Frequency Reserves To The Power Grid Via Demand Response
Angelos Georghiou, Dr, Swiss Federal Institute of Technology,
Zurich, Switzerland,
angelosg@control.ee.ethz.ch, Xiaojing Zhang,
Maryam Kamgarpour, John Lygeros
Given a fixed uncertainty set, robust control finds a policy that minimizes a given
cost while satisfying the system’s constraints for all uncertainty realizations. In
this work, we extend the robust control setup by allowing both the policies and
the uncertainty sets to be decision-dependent, which we refer to as adjustable
uncertainty sets. By restricting the set of admissible policies, we can cast the
problem as a tractable convex optimization problem. We showcase the
effectiveness of our approach on a demand response problem, providing
frequency reserves to the power grid.
2 - Two-stage Distributionally Robust Linear Programming Over
Wasserstein Balls
Grani Adiwena Hanasusanto, Assistant Professor,
The University of Texas at Austin, Austin, TX, United States,
grani.hanasusanto@utexas.edu,Daniel Kuhn
We study two-stage stochastic linear programming problems where the
distribution of the uncertain parameters is ambiguous and is only known to
belong to a family of all distributions that are close to the empirical distribution
with respect to the Wasserstein metric. We derive an exact copositive program for
the generic problems and formulate a tractable linear program for instances with
only right-hand side uncertainty. We illustrate the effectiveness of our
reformulations in numerical experiments and demonstrate their superiority over
the classical sample average approximation scheme and the state-of-the-art
moment-based model.
3 - Bounds On Random Binary Quadratic Programs
Karthik Natarajan, Singapore University of Technology and Design,
karthik_natarajan@sutd.edu.sgIn this paper, we consider a binary quadratic program (BQP) with random
objective coefficients. Given information on the marginal distributions of the
objective coefficients, we propose new bounds on the expected optimal value of
the random BQP. Preliminary computational results on random quadratic
unconstrained binary optimization problems and random quadratic knapsack
problems provides evidence of the quality of the bounds.
4 - Multi-objective Robust Optimization
Aurelie Thiele, Associate Professor, Southern Methodist University,
Dallas, TX, United States,
aurelie@alum.mit.eduWe investigate robust optimization frameworks for decision-making under high
uncertainty when the decision maker has multiple, possibly conflicting objectives.
Of particular interest is a situation with competitors seeking to either maintain or
gain the lead in some of the markets the decision maker operates in. The decision
maker must decide whether to use his budget defending his current positions or
trying to take the lead in others. We investigate distributionally robust paradigms
in those cases.
MD17
105B-MCC
Robust Optimization and Learning
Sponsored: Optimization, Optimization Under Uncertainty
Sponsored Session
Chair: Xi Chen, Assistant Professor, New York University, 44 West 4th
Street, New York, NY, 10012, United States,
xchen3@stern.nyu.eduCo-Chair: Qihang Lin, The University of Iowa, 101 Jessup Hall,
Iowa City, IA, 52242, United States,
qihang-lin@uiowa.edu1 - Piecewise Affine Policies For Two-stage Optimization Under
Demand Uncertainty
Vineet Goyal, Columbia University,
vgoyal@ieor.columbia.edu,
Aharon Ben-Tal, Omar El Housni
We consider the problem of designing piecewise affine policies for two-stage
robust linear optimization under right hand side uncertainty. Such problems arise
in many settings with demand uncertainty. One of the main challenges for
piecewise affine policy is designing the pieces of the uncertainty set. We introduce
a new framework where we approximate the set by a simplex and construct a
piecewise affine policy from a map between the uncertainty set and simplex. Our
policy can be computed efficiently and performs significantly better than affine
policy for many interesting uncertainty sets.
2 - Distributional Robust Analysis For Log-concave And
IFR Distributions
Simai He, Shanghai University of Finance and Economics,
simaihe@mail.shufe.edu.cnDistributional free robust optimization have raised attention for a long time,
however it has been criticized as too over-conservative. Especially, the bounds
yield from the so-called moment problems corresponds to few points discrete
distributions, which are usually considered too extreme in practice. Increasing
failure rate (IFR) and log-concave distribution assumptions are too widely
accepted and applied distribution assumptions in many area. We develop general
distributional free robust optimization theory with such distribution assumptions,
based on optimum solution structure of a particular class of non-convex
optimization problems.
3 - Recovering Statistical Guarantees Via The Empirical
Robust Optimization
Henry Lam, University of Michigan,
khlam@umich.eduWe investigate the use of distributionally robust optimization (DRO) in recovering
the statistical guarantees provided by the best benchmark that is in line with the
central limit theorem, for the feasibility of expected value constraints. We show
that the divergence ball, suitably empirically defined, and with its size calibrated
by the quantile of a chi-square process excursion, amounts to such guarantees.
The construction of this ball deviates from the standard mechanism of DRO in
that the ball can have low, or even zero probability of covering the true
distribution. Rather its performance is explained by connecting the dual of the
DRO with a generalization of the empirical likelihood method.
4 - Optimal Regret In Multi Armed Bandits Under Heavy Tailed And
Correlated Reward Processes
Alexej Proskynitopoulos, Northwestern University, Evanston, IL,
United States,
alexej.proskynitopoulos@kellogg.northwestern.edu,Chaithanya Bandi
It is well known that Thomson Sampling and UCB based algorithms achieve the
optimal regret for Bandits with light tailed reward processes. In this talk, we
present a new framework for deriving these optimal algorithms which achieves
optimal regret for heavy tailed reward processes as well as a bound for correlated
arms. We also derive the order of the optimal regret as a function of the heavy tail
coefficient and correlation.
MD17