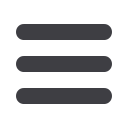

INFORMS Nashville – 2016
214
MD18
106A-MCC
Statistical Learning under a Modern
Optimization Lens
Sponsored: Optimization, Integer and Discrete Optimization
Sponsored Session
Chair: Dimitris Bertsimas, Operations Research Center, MIT, MIT,
E40-111, Cambridge, MA, 02139, United States,
dbertsim@mit.eduCo-Chair: Dimitris Bertsimas, Operations Research Center, MIT, MIT,
E40-111, Cambridge, MA, 02139, United States,
dbertsim@mit.edu1 - Missing Data Imputation Via A Modern Optimization Lens
Colin Pawlowski, Massachusetts Institute of Technology,
Cambridge, MA, United States,
cpawlows@mit.edu,
Dimitris Bertsimas, Ying Zhuo
We propose an optimization framework for missing data imputation. The
formulation imputes missing values to minimize the total distance to the K-
nearest neighbors of each data point. Because the problem is non-convex, we
derive a fast first-order method opt.impute to find high-quality solutions. On a
sample of 64 data sets with 25% hidden values, opt.impute produces the best
overall imputation in 40 data sets benchmarked against state-of-the-art methods.
Further, the relative performance of opt.impute improves as the percentage of
missing data increases, and for problems with the highest percentage of missing
data, the mean absolute deviation from true values is lowered by 2.4%.
2 - Multi-target Tracking Via Mixed Integer Optimization
Shimrit Shtern, Operations Research Center, MIT,
sshtern@mit.edu,Zachary C. Saunders
The Multi-target tracking problem combines the problems of assigning sensor
detections to targets and estimating the target trajectory. Inaccuracies in the
detection process make this a difficult problem. The literature is abundant with
probability based models, but few optimization based models have been
suggested, which mostly focus on model accuracy rather than interoperability or
solvability. In our work we develop a simple and interpretable mixed integer
optimization model, which is warm started by an appropriate heuristic, and yields
high quality solutions in a reasonable time.
3 - Optimal Sparse Inverse Covariance Estimation
Jourdain Bernard Lamperski, Operations Research Center, MIT,
jourdain@mit.eduWe consider the maximum likelihood estimation of sparse inverse covariance
matrices. We formulate the estimation problem as a nonlinear MIP and develop a
novel decomposition approach that solves the formulation to optimality. Using a
variety of datasets, we demonstrate that the approach scales to problem sizes of
interest and yields sparser solutions than existing methods, while maintaining
desirable statistical properties.
4 - Optimal Low Rank Factor Analysis
Martin S. Copenhaver, Operations Research Center, MIT,
Cambridge, MA, United States,
mcopen@mit.edu,Dimitris Bertsimas, Rahul Mazumder
Factor Analysis (FA) is a technique that is widely used to obtain a parsimonious
representation of correlation structure among a set of variables in terms of a
smaller number of common hidden factors. In this talk we revisit the classical
rank-constrained FA problem and propose a flexible family of exact, smooth
reformulations for this task. By coupling state-of-the-art techniques from
nonlinear optimization with methods from discrete and global optimization, we
show that our approach often finds certifiably optimal solutions and significantly
outperforms existing publicly available methods across a variety of real and
synthetic examples.
MD19
106B-MCC
Networks and Geography in Optimization
Sponsored: Computing
Sponsored Session
Chair: John Gunnar Carlsson, University of Southern California,
University of Southern California, Los Angeles, CA
United States,
jcarlsso@usc.edu1 - A Decomposition Heuristic For The One-warehouse Multi-retailer
Problem With Batch Costs
Alejandro Toriello, Georgia Institute of Technology, Atlanta, GA,
United States,
atoriello@isye.gatech.edu,Weihong Hu
We consider a one-warehouse, multi-retailer system with deterministic dynamic
demand over a discrete finite horizon, where orders placed by any facility incur
batched fixed costs. We propose a decomposition heuristic that solves the
warehouse’s and retailers’ problems separately and then judiciously converts the
separate solutions into a globally feasible solution with worst-case approximation
guarantee of 2; this improves the previously best-known ratio of 3.6. Time
permitting, we will discuss the extension to concave batch costs.
2 - Bounds For The Euclidean Generalized Travelling
Salesman Problem
John Gunnar Carlsson, University of Southern California,
jcarlsso@usc.eduWe study the Euclidean generalized travelling salesman problem (GTSP), in
which the goal is to find a tour that visits one element from each of a collection of
point sets. Our results characterize the long-term, asymptotic behavior of the tour
length when a large number of points are sampled, and give rise to a number of
counterintuitive managerial insights.
3 - Distributionally Robust Travelling Salesman Problem With
Wasserstein Distance
Mehdi Behroozi, Visiting Scholar, University of Southern
California, Los Angeles, CA, United States,
mehdi.behroozi@usc.edu,John Gunnar Carlsson
Recent research on the robust and stochastic Euclidean travelling salesman
problem has seen many different approaches for describing the region of
uncertainty, such as taking convex combinations of observed demand vectors or
imposing constraints on the moments of the spatial demand distribution. In this
paper, we consider a distributionally robust version of the Euclidean travelling
salesman problem in which we compute the worst-case spatial distribution of
demand against all distributions whose Wasserstein distance to an observed
demand distribution is bounded from above. This constraint allows us to
circumvent common overestimation that arises when other procedures are used.
MD20
106C-MCC
Recent Developments in Multistage
Stochastic Programming
Invited: Tutorial
Invited Session
Chair: Alan King, IBM Research, 1, Yorktown Heights, NY, 15,
United States,
kingaj@us.ibm.com1 - Recent Developments In Multistage Stochastic Programming
Alan King, IBM Research, Yorktown Heights, NY, 10,
United States,
kingaj@us.ibm.comMultistage stochastic programming is a framework for applying large scale
optimization technologies to multiperiod decision making under uncertainty. This
talk will review the past decade and a half’s developments in multistage stochastic
programming, including risk functionals, Stochastic Dual Dynamic Programming,
time-consistent risk measures, and quantization of scenario trees.
MD21
107A-MCC
Healthcare Information Systems
Sponsored: Health Applications
Sponsored Session
Chair: Yi-Chin Lin, Hofstra University, Hempstead, Weller Hall 032,
Hempstead, NY, 11549-1000, United States,
yichin.kl@gmail.comCo-Chair: Rema Padman, Carnegie Mellon University, 5000 Forbes
Avenue, Pittsburgh, PA, 15213, United States,
rpadman@cmu.edu1 - Physician Quality And Online Reviews
Danish Saifee, PhD Student, Naveen Jindal School of
Management/The University of Texas at Dallas,
800 West Campbell Road, Richardson, TX, 75080, United States,
dxs121230@utdallas.edu, Indranil Bardhan, Eric Zheng
We investigate how online reviews and physician competence differ in terms of
their impact on clinical outcomes. Using a panel of COPD patients, we find that
physicians with higher competence exhibit better patient outcomes.
MD18