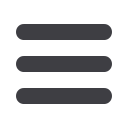

INFORMS Nashville – 2016
220
MD35
205A-MCC
Empirical Service Operations
Sponsored: Manufacturing & Service Oper Mgmt,
Service Operations
Sponsored Session
Chair: Robert Louis Bray, Kellogg School of Management,
830 Hinman Ave, Apt 2S, Evanston, IL, 60202, United States,
robertlbray@gmail.com1 - Modeling Growth In Service Operations: Evidence From
The App Economy
Ken Moon, The Wharton School, Philadelphia, PA, United States,
kenmoon@wharton.upenn.edu, Haim Mendelson
The best service firms expand and sustain their customer bases and profits
organically through word of mouth and customer retention. We propose a
customer-flow model fashioned after classical service operations models that
focuses on the effects of customer retention, usage frequency, and growth (RFG).
Using daily, weekly, and monthly usership data for services in the app economy,
our results empirically demonstrate the importance of RFG, including that new
apps score increasingly higher in growth by improving in service quality. Finally,
we present evidence of an experience curve, analogous to that in manufacturing,
whereby service events drive advances in service quality and RFG.
2 - Managing Product Quality In The Face Of Field Failures
Ahmet Colak, Northwestern University, Evanston, IL,
United States,
a-colak@kellogg.northwestern.eduWe model a manufacturer’s and regulator’s joint recall decisions as a dynamic
discrete choice game. We estimate our model with 14,124 U.S. auto recalls and
976,062 defect reports over the period 1994—2015. We find that (i) automakers
initiate recalls mainly to avoid field failure costs, and (ii) automakers don’t
preempt the regulator’s interventions in 86% of our sample.
3 - Impact Of Callers’ History On Abandonment:
Model And Implications
Seyed Emadi, UNC - Kenan Flagler Business School, Chapel Hill,
NC, 27599, United States,
Seyed_Emadi@kenan-flagler.unc.edu,Jayashankar M Swaminathan
Caller abandonment could depend on their past waiting experiences. To tease out
the impact of callers’ waiting experiences on their abandonment behavior from
the impact of their heterogeneity, we use a structural estimation approach in a
Bayesian learning setting. Our framework has managerial implications at both
tactical and operational levels such as managing customer expectation about their
delays in the system, and implementation of patience-based priority policies such
as Least-Patience-First and Most-Patience-First scheduling.
4 - Multitasking, Multi-armed Bandits, And The Italian Judiciary
Robert Louis Bray, Kellogg School of Management,
robertlbray@gmail.comWe model how a judge schedules cases as a multi-armed bandit problem. The
model indicates that a first-in-first-out (FIFO) scheduling policy is optimal when
the case completion hazard rate function is monotonic. But there are two ways to
implement FIFO in this context: at the hearing level or at the case level. Our
model indicates the latter policy, prioritizing the oldest case, is optimal when the
case completion hazard rate function increases. This result convinced six judges of
the Roman Labor Court of Appeals—-a court that exhibits increasing hazard
rates—-to switch from hearing-level FIFO to case-level FIFO. We estimate that
our intervention decreased the average case duration by 12%.
MD36
205B-MCC
Control, Learning, and Strategic Behavior in
Queueing Models
Sponsored: Manufacturing & Service Oper Mgmt,
Service Operations
Sponsored Session
Chair: Philipp Afeche, University of Toronto, 105 St. George Street,
Toronto, ON, M5S 3E6, Canada,
afeche@rotman.utoronto.ca1 - Dynamic Control Of A Call Center With The Callback Option
Xiaoshan Peng, University of Chicago Booth School of Business,
x-peng@chicagobooth.edu, Baris Ata
We investigate a call center with the callback option. An incoming customer is
routed to an online queue or to an offline queue where she needs to hang up the
phone and waits for the system to call her back. We characterize the optimal
routing policy and service policy when the forecast of the arrival rate is available.
2 - Learning And Impatience In Queues
Senthil Veeraraghavan, Wharton School of the University of
Pennsylvania,
senthilv@upenn.edu,Li Xiao, Hanqin Zhang
We study the abandonment behavior of customers in M/M/1+G by the Bayesian
learning approach. An arriving impatient customer knows the average arrival rate
but does not know the average service rate. To have a rational abandonment, the
arriving impatient customer has to learn the service rate. Two Bayesian learning
ways are discussed in accordance with what kind information of the queueing
system is available to the arriving impatient customer. Based on the learned
service rate, the abandonment behavior is quantitatively characterized by the
utility of the arriving impatient customer.
3 - Jumping The Line, Charitably: Analysis And Remedy Of The
Donor Priority Rule
Tinglong Dai, Assistant Professor, Johns Hopkins University,
100 International Drive, Baltimore, MD, 21202, United States,
dai@jhu.edu, Ronghuo Zheng, Katia P. Sycara
In the United States, the growth of the organ transplantation waiting list
significantly outpaces the supply of donated cadaveric organs. Among a myriad of
initiatives aiming to boost the supply, the donor priority rule, under which a
registered organ donor, in case of needing a transplant, is given priority to receive
a donated organ, has been weighed by U.S. policy makers. In this paper, we
model the U.S. organ donation and allocation system using the strategic queueing
theoretic framework. We propose a simple freeze-period mechanism, and prove
that in conjunction with the donor priority rule, it can increase the supply of
donated organs without compromising the average quality of the donor pool.
4 - Learning And Earning For Congestion-prone Service Systems
N. Bora Keskin, Duke University, Durham, NC, United States,
bora.keskin@duke.edu,Philipp Afeche
Consider a firm selling a service in a congestion-prone system to price- and delay-
sensitive customers. The firm faces Bayesian uncertainty about the consumer
demand for its service and can dynamically make noisy observations on the
demand. We characterize the structure and performance of the myopic Bayesian
policy and well-performing variants.
MD37
205C-MCC
Sustainability in Supply Chains
Sponsored: Manufacturing & Service Oper Mgmt,
Sustainable Operations
Sponsored Session
Chair: Georgia Perakis, Massachusetts Institute of Technology,
Cambridge, MA, United States,
georgiap@mit.eduCo-Chair: Maxime Cohen, Google NYC, New York, NY, United States,
maxccohen@gmail.com1 - Optimal Stopping Of Subsidies To Products With
Network Externality
Ningyuan Chen, HKUST, Hong Kong, Hong Kong,
nychen@ust.hk,
Saed Alizamir, Vahideh Manshadi
Many products exhibit network externality: a customer who has purchased the
product makes his/her neighbors or friends more likely to buy the same product.
This includes eco-friendly products such as electronic cars and solar panels. The
government subsidizes customers to promote such products. We find that it is
optimal for the government to stop the subsidy when the total externality of the
owners reaches a threshold, which depends on the spectrum of the externality
matrix. The optimal stopping time is not monotone in the strength of the
externality between customers. We investigate how the structure of the network
affects the stopping time and the optimal reward of the government.
2 - A Unifying Framework For Consumer Surplus Under
Demand Uncertainty
Charles Thraves, MIT,
cthraves@mit.edu, Maxime Cohen,
Georgia Perakis
We present a general framework for consumer surplus when demand is stochastic
and there are multiple items. We take a utility maximization approach in order to
study the impact of demand uncertainty on consumers in several interesting
settings. We show how the impact of uncertainty on consumers depends on the
demand shape (convexity) and the allocation rule. In many settings we show that
it can in fact hurt consumers.
MD35