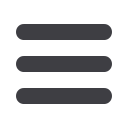

INFORMS Nashville – 2016
221
3 - Price Competition Based On Relative Prices Or Subsidies
Awi Federgruen, Columbia University, New York, NY,
United States,
af7@gsb.columbia.edu,Lijian Lu
We consider price competition models for oligopolistic markets in which the
consumer reacts to relative rather than absolute prices. The relative price is
defined as the difference between the absolute price and a reference value., either
a third party subsidy or a prospect theoretical reference price. Application areas
We review five different application areas. We then characterize the equilibrium
behavior under general reference value scheme , assuming the consumer choice
model is of the general MultiNomial Logit type. We also derive comparison results
for the price equilibria that arise under alternative Subsidy schemes. These have
important applications for the design of subsidy schemes.
4 - Risk-aware Demand Management Of Aggregators Participating In
Energy Programs With Utilities
Ana Radovanovic, Google,
anaradovanovic@google.comWe present a methodology for modeling and optimally managing the demand of
an aggregator with deferrable (flexible) loads (e.g., electric vehicles and HVACs)
under uncertainty. We propose a unified framework for treating different types of
flexible loads, that captures uncertainties in their parameters, and environmental
conditions they are exposed to. Our optimization formulation minimizes the total
expected cost, whose goal is to optimally balance two terms: user discomfort cost
(regret), and cost paid to the utility. We propose a cost-efficient procedure for risk
estimation, and provide guidelines for its consideration in cost-effective program
selection.
MD38
206A-MCC
Reliability
Contributed Session
Chair: Zhimin Xi, University of Michigan-Dearborn, 4901 Evergreen
Road, 2240 HPEC, Dearborn, MI, 48128, United States,
zxi@umich.edu1 - Estimation Of Field Reliability Based On Aggregate Lifetime Data
Piao Chen, National University of Singapore, Singapore,
Singapore,
cp@u.nus.eduBecause of the exponential distribution assumption, many reliability databases
recorded data in an aggregate way. The data format is different from traditional
lifetime data and the statistical inference is challenging. In this study, we model
the aggregate data by gamma distribution and inverse Gaussian (IG) distribution.
Statistical inference methods are proposed.
2 - ALT With Exponentially Changing Stress Durations Under
Cost Constraint
David Han, University of Texas at San Antonio, Management
Science & Statistics, College of Business, San Antonio, TX,
78249-0632, United States,
david.han@utsa.eduWhen designing ALT, several variables such as the allocation proportions and
stress durations must be determined carefully because of constrained resources.
This talk discusses the optimal decision variables based on the popular optimality
criteria under the constraint that the total cost does not exceed a pre-specified
budget. A general scale family of distributions is considered to accommodate
different lifetime models for flexible modeling with exponentially decreasing
stress durations.
3 - Fault Localization Of A Series System When Tests Are Imperfect
Tonguc Unluyurt, Sabanci University, Orhanli Tuzla, Istanbul,
34956, Turkey,
tonguc@sabanciuniv.edu, Zahed Shahmoradi
We consider a failed series system. The goal is to find the component that caused
the failure with the minimum expected cost. In order to do this, we conduct
costly tests and we know the probability that a certain component is the reason of
the failure. The complicating factor is the fact that tests are imperfect and this is
described by type I and type II error probabilities. In addition to the testing costs,
we also consider misclassification costs. In order to decrease the total cost we
develop a model that allows repetition of the tests in a certain way. We compute
the expected cost of such a solution and we demonstrate the potential savings
resulting from repetition of tests.
4 - Model Uncertainty Approximation Using A Copula-based
Approach For Reliability Based Design Optimization
Zhimin Xi, University of Michigan-Dearborn, 4901 Evergreen
Road, 2240 HPEC, Dearborn, MI, 48128, United States,
zxi@umich.eduReliability-based design optimization (RBDO) has been widely used to design
engineering products with minimum cost function while meeting reliability
constraints. Model uncertainty, i.e., the uncertainty of model bias indicating the
inherent model inadequacy for representing the real physical system, is typically
overlooked in RBDO. This paper addresses model uncertainty approximation in a
product design space and further integrates the model uncertainty into RBDO. In
particular, a copula-based bias modeling approach is proposed and results are
demonstrated by two vehicle design problems.
MD39
207A-MCC
Markov Lecture
Sponsored: Applied Probability
Sponsored Session
Chair: David Goldberg, GA Institute of Technology, Atlanta, GA
30332-0205,
dgoldberg9@isye.gatech.eduCo-Chair: Rouba Ibrahim, University College London,
rouba.ibrahim@ucl.ac.uk1 - Piecewise Deterministic Markov Processes For Monte Carlo
Gareth Roberts, University of Warwick, Coventry,
United Kingdom,
Gareth.O.Roberts@warwick.ac.ukTraditional MCMC approaches for sampling complex distributions are almost all
based on the Metropolis-Hastings framework, which, although versatile, restrict
algorithms to be reversible, discrete time, and to require target density evaluation
at each iteration. All of these features can sometimes be significant disadvantages.
To overcome these difficulties, new algorithms are being developed which are
non-reversible and continuous in time, for example the Zigzag, Bouncy Particle
sampler, and the SCALE and CIS algorithms. The first two of these are pure
MCMC algorithms whereas the latter two involve combination with sequential
Monte Carlo methods. They all share the property that they can be couched in
terms of Piecewise Deterministic Markov processes. The presentation will also
touch on the impressive theoretical and empirical properties of these methods,
including the super-efficiency properties of the zigzag algorithm, the unbiased
subsampling properties of the SCALE approach and the stability of the CIS
importance sampling approach, and is based on joint work with Joris Bierkens,
Paul Fearnhead, Adam Johansen, and Krys Latuszynski.
2 - Adaptive MCMC For Everyone
Jeffrey Rosenthal, University of Toronto, Toronto, ON, Canada,
rosenthal,
jeff@math.toronto.eduIn this discussion, we shall briefly discuss the theory behind “adaptive” Markov
chain Monte Carlo (MCMC), which automatically modify the algorithm while it
runs in an effort to improve its performance “on the fly”. Adaptation can greatly
improve convergence, but it can also destroy the ergodicity properties necessary
for the algorithm to be valid. We shall present some examples and theorems
concerning the ergodicity and efficiency of adaptive MCMC, with an aim towards
making it more widely applicable in broader contexts
3 - Optimal Scaling Of MCMC Algorithms
Natesh Pillai, Harvard University, Cambridge, MA, United States,
nateshspillai@gmail.comIn this discussion, we will give a brief overview of optimal scaling of MCMC
algorithms. Optimal scaling offers a new perspective for studying the efficiency of
MCMC algorithms in high dimensions. The key idea is to study the properties of
the proposal distribution as a function of the dimension. This point of view gives
us new insights on the behavior of the algorithm, such as precise estimates of the
number of steps required to explore the target measure as a function of the
dimension of the state space. After reviewing the original results, we will mention
some recent progress as well.
MD40
207B-MCC
Theoretical Development in Estimation
Invited: Data Envelopment Analysis
Invited Session
Chair: Ole Olesen, Southern Denmark University, Campusvej 55,
Odense, 5230, Denmark,
ole@sam.sdu.dk1 - An Improved Afriat-Diewert-Parkan Nonparametric Production
Function Estimator
Ole Olesen, Southern Denmark University,
ole@sam.sdu.dk,
John Ruggiero
Nonparametric regression estimators with shape constraints have recently been
extended based on the Afriat inequalities. Overfitting of the ADP estimator
suggests that estimators based on a weighted average of restricted estimators may
provide an equally unbiased estimator but an estimator with lower variance. Both
an Average Random k-Hinge estimator, a Jackknife Model Average (JMA) or a
slightly modified JMA are considered. Small sample properties of the estimators
are presented
MD40