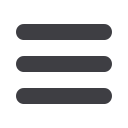

INFORMS Philadelphia – 2015
170
2 - Real-time Monitoring for Additive Manufacturing using Online
Sparse Estimation Based Classification
Kaveh Bastani, Research Assistant, Virginia Tech University,
106 Durham Hall (MC 0118) 1145 Perry Str, Blacksburg,VA,
United States of America,
kaveh@vt.edu, Zhenyu Kong
The objective of this work is to realize real-time monitoring of additive
manufacturing processes using multiple sensor signals. To achieve this objective,
an approach invoking the concept of sparse estimation called online sparse
estimation-based classification (OSEC) is proposed. The OSEC approach is
equipped with a novel computationally fast sparse estimation algorithm to
facilitate real-time monitoring applications.
3 - Diagnostic Monitoring of Multivariate Process via a LASSO-BN
Formulation
Yan Jin, University of Washington, 530 NE 103rd St, Seattle, WA,
98125, United States of America,
yanjin@uw.edu,
Guan Wang, Shuai Huang, Houtao Deng
Fault detection and root-cause diagnosis are usually considered as two separate
tasks in most existing process monitoring methods. While they could reinforce
each other, we propose a diagnostic monitoring approach that unifies monitoring
and root-cause diagnosis by integrating process monitoring, Bayesian network,
and sparse learning.
4 - Multi-stage Nanocrystal Growth Identifying and Modeling via
In-situ TEM Video
Yanjun Qian, PhD Candidate, TAMU, 1501 Harvey Rd,
Apt 806, College Station, TX, 77840, United States of America,
qianyanjun09@gmail.comWhile in-situ transmission electron microscopy technique has caught a lot of
recent attention, one of the bottlenecks appears to be the lack of automated and
quantitative analytic tools. We introduce an automated tool suitable for analyzing
the in-situ TEM videos. It learns and tracks the normalized particle size
distribution and identifies the phase change points delineating the stages in
nanocrystal growth. We furthermore produce a quantitative physical-based
model.
5 - Rul Prediction Based on Noisy Condition Monitoring Signals using
Constrained Kalman Filter
Junbo Son, PhD Candidate, University of Wisconsin-Madison,
1513 University Avenue, Madison, WI, 53706, United States of
America,
json5@wisc.edu, Shiyu Zhou, Chaitanya Sankavaram,
Yilu Zhang, Xinyu Du
In this paper, a robust statistical prognostic method is proposed to predict the
remaining useful life of individual units based on condition monitoring signals
that are contaminated by severe noises. The proposed method defines a set of
inequality constraints so that satisfactory prediction accuracy can be achieved
regardless of the noise level. The advantageous features of the proposed method is
demonstrated by both numerical studies and a case study with real world
automotive battery data.
MA73
73-Room 203B, CC
IEEE T-ASE Invited Session: Healthcare and Service
Systems Automation
Sponsor: Quality, Statistics and Reliability
Sponsored Session
Chair: Jingshan Li, Professor, 1513 University Ave, Madison, WI,
53706, United States of America,
jli252@wisc.edu1 - N-k Power Problem in the Power Systems: A Budget
Allocation Perspective
Loo Hay Lee, National University of Singapore,
Department of Industrial & Systems, Engineering, Singapore,
iseleelh@nus.edu.sg,Chun-hung Chen, Ek Peng Chew,
Giulia Pedrielli, Haobin Li, John Shortle, Yue Liu
In the stochastic N-k power network problem, N choose k failures result in a
probability of the entire system to fail (unsafe system). We want to determine if
the system is unsafe, when failures are estimated through noisy simulations, by
optimal computing budget allocation. The solution method is based on the
likelihood of the system to be safe (unsafe), whose estimation is sequentially
improved to be given as input to a tailored budget allocation that also considers
the observed system state.
2 - Integrating OCBA and GA to Find the Approximate Pareto Patient
Flow Distribution
Jie Song, Peking University, Room 512,Fangzheng Building,
Beijing, China,
songjie@coe.pku.edu.cn, Zekun Liu, Yunzhe Qiu
We develop a methodology to find the optimal macro-level patient flow
distribution in terms of multi-dimension inputs and outputs for the hierarchical
healthcare system. The proposed method integrates the discrete event simulation,
the multi-objective optimization, and the simulation budget allocation to
comprehensively improve the overall system performances. A case study based on
the real data is carried out to validate and implement the proposed method.
3 - Improving Response-Time Performance in Acute Care Delivery:
A Systems Approach
Xiaolei Xie, Department of Industrial Engineering, Tsinghua
University, 614 Shunde Building, Tsinghua University, Beijing,
China,
xxie@tsinghua.edu.cn,Colleen Swartz, Paul Depriest,
Jingshan Li
In response to a patient with acute physiological deterioration, we study the
probability that an appropriate decision is made within a desired time period,
referred to as response time performance (RTP). First, a closed formula to
evaluate RTP is derived by assuming exponential response time, which is followed
by bottleneck analysis. Then, under general case, an approximation approach is
proposed to evaluate RTP. Finally, a case study is introduced to illustrate the
applicability of the method.
4 - Spatiotemporal Differentiation of Myocardial Infarctions
Chen Kan, University of South Florida, 4202 E. Fowler Ave.
ENB118, Tampa, FL, United States of America,
chenkan@mail.usf.edu,Hui Yang
This paper presents a novel warping approach to quantify the dissimilarity of
disease-altered patterns in 3-lead VCGs. The hypothesis testing shows that there
are significant space-time differences between healthy and diseased subjects.
Further, we optimize the embedding of each VCG as a feature vector in the high-
dimensional space that preserves the dissimilarity distance matrix. Experimental
results demonstrated that this novel approach improves the performance of
predictive models.
MA74
74-Room 204A, CC
Data Analytics for Quality Control and Improvement I
Sponsor: Quality, Statistics and Reliability
Sponsored Session
Chair: Kaibo Liu, Assitant Professor, UW-Madison, 1513 University
Avenue, Madison, WI, 53706, United States of America,
kliu8@wisc.edu1 - A Distribution Free Procedure for Fault Identification in
High-Dimensional Processes
Mehmet Turkoz, Rutgers University, 16 Rachel Terrace,
Piscataway, NJ, 08854, United States of America,
turkoz@scarletmail.rutgers.edu,Sangahn Kim, Young-seon
Jeong, Myong K (MK) Jeong, Elsayed Elsayed, K.N. Al-khalifa,
Abdel Magid Hamouda
In a process with high-dimension, identifying which variables cause an out-of-
control signal is a challenging issue for quality problems. Even though there are
many procedures for fault identification, most of them assume the normal
distribution. However, many real life problems come from multivariate non-
normal distribution. We present a new fault identification method that does not
assume any specific probability distribution.
2 - Broaching Process Modeling Based on Non-repeating
Cyclic Signals
Meg Tian, Graduate Student, Virginia Tech, 250 Durham Hall,
Blacksburg, VA, 24060, United States of America,
tian0414@vt.edu, Jaime Camelio, Ran Jin, Lee Wells
Broaching is often used to produce complex contours by sequentially removing
material via multiple cutting teeth. The broaching force signal exhibits a non-
repeating cyclic pattern. A new approach is proposed to model the non-repeating
cyclic signals and thus detect changes in a broaching process.
3 - Heterogeneous Recurrence T^2 Charts for Monitoring and
Control of Nonlinear Dynamic Processes
Yun Chen, University of South Florida, 4202 E. Fowler Ave.
ENB118, Tampa, FL, United States of America,
yunchen@mail.usf.edu, Hui Yang
This paper presents a new approach of heterogeneous recurrence T^2 control
chart for online monitoring and anomaly detection in nonlinear dynamic
processes. An effective partition scheme is firstly developed to delineate local
recurrence regions in the multi-dimensional continuous state space. Further, we
designed a new fractal representation of state transitions among recurrence
regions, and then develop new measures for on-line monitoring and predictive
control of process recurrences.
MA73