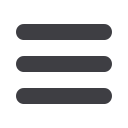

INFORMS Philadelphia – 2015
176
2 - A Novel Strategy for General Polynomial Partitions in
Multiparametric Programming
Stratos Pistikopoulos, Texas A&M University, Artie McFerrin Dept.
of Chem. Eng., College Station, TX, 77843,
United States of America,
stratos@tamu.edu,Richard Oberdieck
Currently, the solution to multiparametric mixed-integer programming problems
is presented as a polyhedral partitioning of the considered parameter space,
because no strategy exists to explicitly handle any nonconvex partitions. In this
work, we demonstrate a novel approach which allows for the handling of general,
polynomial partitions in multiparametric programming using a combination of
suitable linearizations and global optimization strategies.
3 - Polyhedral Cut Generation for Global Optimization of Problems
with Edge-concave Intermediates
Yash Puranik, Carnegie Mellon University, Pittsburgh, PA,
ypp@andrew.cmu.edu,Nikolaos Sahinidis
We describe a branch-and-cut implementation for obtaining facet defining cuts
that utilizes a highly efficient solution strategy for linear separation problems.
These cuts are valid for edge-concave functional forms which admit polyhedral
convex envelopes. Computational performance results of this implementation are
presented on standard test libraries.
4 - Recent Developments in BARON for Global Optimization of
NIPS and MINLPS
Mustafa Kilinc, Carnegie Mellon University, 5000 Forbes Ave,
Pittsburgh, PA, United States of America,
mkilinc@andrew.cmu.edu, Nikolaos Sahinidis
We report recent developments in the integer arsenal of branch-and-reduce and
their implementation in the global optimization software BARON. Extensive
computational results will be presented on problems from a collection of test sets.
MB13
13-Franklin 3, Marriott
Distributed Stochastic Optimization for Large-Scale
Machine Learning
Sponsor: Optimization/Optimization Under Uncertainty
Sponsored Session
Chair: Qihang Lin, The University of Iowa, 21 East Market Street, Iowa
City, IA, 52245, United States of America,
qihang-lin@uiowa.edu1 - Adding vs. Averaging in Distributed Primal-dual Optimization
Chenxin Ma, PhD Student, Lehigh University, 200 West Packer
Avenue, Bethlehem, PA, 18015, United States of America,
machenxin622@gmail.com, Peter Richtárik, Virginia Smith,
Martin Jaggi, Michael Jordan, Martin Takac
Reducing communication makes the efficient aggregation of partial work from
different machines more challenging. We present a novel generalization of the
recent communication efficient primal-dual coordinate ascent framework
(CoCoA). Our framework, CoCoA+, allows for additive combination of local
updates to the global parameters at each iteration, whereas previous schemes only
allowed conservative averaging.
2 - DSCOVR: A Randomized Asynchronous Algorithm for Distributed
Learning with Parameter Server
Adams Wei Yu, PhD Student, Carnegie Mellon University,
Pittsburgh, PA, United States of America,
adamsyuwei@gmail.com,Qihang Lin, Lin Xiao, Weizhu Chen
Machine learning with big data often involves big models, where the number of
variables in a model can be too large for frequent communication and
synchronization. In this case, we can set up a parameter server to maintain the
overall model and coordinate updates of subsets of the parameters at different
machines. We propose an algorithm DSCOVR, which exploits the double
partitions in both data and model to gain parallelism, and applies periodic
variance reduction to achieve linear convergence.
3 - Massively Distributed Optimization: Beyond the
Traditional Setting
Jakub Konecn, University of Edinburgh, James Clerk Maxwell
Building, 5406, Peter Guthrie Tait Road, Edinburgh, EH9 3DF,
United Kingdom,
J.Konecny@sms.ed.ac.uk,Brendan Mcmahan
The purpose of this work is to present a new, increasingly important setting for
distributed optimization in machine learning. Main assumption is that we have a
very large number of computers available, each of which has access to relatively
small number of training examples. This is arising if the data are not stored on
datacenters owned by companies, but instead kept at users’ devices, a arising
trend driven primarily by privacy concerns. We demonstrate that encouraging
results are achievable.
MB14
14-Franklin 4, Marriott
Statistical Optimization
Sponsor: Optimization/Optimization Under Uncertainty
Sponsored Session
Chair: Mengdi Wang, Assistant Professor, Princeton University,
302 Trinity Ct #2, Princeton, NJ, 08540, United States of America,
mengdiw@princeton.edu1 - Minimax-optimal Private-preserving Sparse PCA in
Distributed Systems
Jian Ge, Princeton University, Operations Research and Financial
Engine, Sherrerd Hall, Charlton Street, Princeton, NJ, 08544,
United States of America,
jiange@exchange.Princeton.EDUWe propose a distributed private-preserving sparse PCA (DPS-PCA) algorithm that
generates a minimax-optimal sparse PCA estimator in polynomial time under
differential privacy constraints. Data providers can use this algorithm to
collaboratively analyze the union of their data sets in a distributed system while
limiting the disclosure of their private information.
2 - Upper Bounds for the Correlated Bayesian Information
Filtering Problem
Bangrui Chen, Cornell University, 55 Lois Ln, Ithaca, NY, 14850,
United States of America,
bc496@cornell.edu, Peter Frazier
We present a Bayesian sequential decision-making formulation of the information
filtering problem, in which an algorithm presents items (blog posts, scientific
papers, emails) arriving in a stream, learning relevance from user feedback on
presented items. Our formulation uses a linear model, and is similar to a Bayesian
linear bandit. We compute an upper bound on the value of the optimal policy,
which allows computing an optimality gap for heuristic policies, and motivates an
index policy.
3 - Statistical Limits of Convex Relaxations
Zhaoran Wang, Graduate Student, Princeton University, Sherrerd
Hall, Charlton Street, Princeton, NJ, United States of America,
zhaoran@exchange.Princeton.EDU, Quanquan Gu, Han Liu
In this paper, we study the statistical limits of convex relaxations. Particularly, we
consider two problems: Mean estimation for sparse principal submatrix and edge
probability estimation for stochastic block model. We exploit the sum-of-squares
relaxation hierarchy to sharply characterize the limits of a broad class of convex
relaxations. Our result shows statistical optimality needs to be compromised for
achieving computational tractability using convex relaxations.
4 - Post-regularization Confidence Bands for High Dimensional
Nonparametric Models with Local Sparsity
Junwei Lu, Princeton University, Sherrerd Hall, Charlton St.,
Princeton, NJ, 08540, United States of America,
junweil@princeton.edu, Mladen Kolar, Han Liu
We propose a novel high dimensional nonparametric model named ATLAS which
is a generalization of the sparse additive model. We aim to estimate high
dimensional function using a novel kernel-sieve hybrid regression estimator that
combines the local kernel regression with the B-spline basis approximation. We
show the estimation rate of true function in the supremum norm. We also
propose two types of confidence bands for true function.
MB15
15-Franklin 5, Marriott
Unconstrained and Bound-Constrained Optimization
Sponsor: Optimization/Nonlinear Programming
Sponsored Session
Chair: Daniel Robinson, Assistant Professor, Johns Hopkins University,
3400 N. Charles Street, Baltimore, MD, 21218, United States of
America,
daniel.p.robinson@gmail.com1 - A Solver for Nonconvex Bound-constrained
Quadratic Optimization
Daniel Robinson, Assistant Professor, Johns Hopkins University,
3400 N. Charles Street, Baltimore, MD, 21218, United States of
America,
daniel.p.robinson@gmail.com,Hassan Mohy-ud-din
I present a new method for optimizing quadratic functions subject to simple
bound constraints. If the problem happens to be strictly convex, the algorithm
reduces to an efficient method by Dostal and Schoberl. Our algorithm, however, is
also able to efficiently solve nonconvex problems. During this talk I will present
the algorithm, a sketch of the convergence theory, and numerical results for
convex and nonconvex problems.
MB13