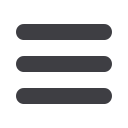

INFORMS Philadelphia – 2015
181
MB28
28-Room 405, Marriott
Economic Models of Auctions
Cluster: Auctions
Invited Session
Chair: Brian Baisa, Assistant Professor, Amherst College,
100 Boltwood Ave, Amherst, MA, 01002, United States of America,
bbaisa@amherst.edu1 - A Detail-free and Efficient Auction for Budget
Constrained Bidders
Brian Baisa, Assistant Professor, Amherst College, 100 Boltwood
Ave, Amherst, MA, 01002, United States of America,
bbaisa@amherst.eduI study auctions for divisible goods where bidders have private values and private
budgets. My main result shows that when bidders have full-support beliefs over
their rivals’ types, a clinching auction played by proxy-bidders implements a
Pareto efficient outcome. Bid behavior is derived using two rounds of iterative
deletion of weakly dominated strategies. This contrasts with recent work that
shows efficient auction design is incompatible with dominant strategy incentive
compatibility.
2 - Weak Cartels and Collusion-proof Auctions
Jinwoo Kim, Associate Professor, Seoul National University,
1 Gwanak-ro Gwanak-gu, Seoul, Korea, Republic of,
jikim72@gmail.com,Yeon-koo Che, Daniele Condorelli
We study collusion in auctions by cartels whose members cannot exchange side-
payments (i.e., weak cartels). We provide a complete characterization of
outcomes that are implementable in the presence of weak cartels, identifying the
set of circumstances under which standard auctions are susceptible to them. We
then solve for optimal collusion-proof auctions and show that they can be made
robust to the specific details of how cartels are formed and operated.
3 - Multi-unit Auctions with a Large Bidder
Justin Burkett, Wake Forest University, Box 7505,
Winston-Salem, NC, 27104, United States of America,
burketje@wfu.edu, Brian Baisa
Recent work in IPV settings shows that the uniform-price and discriminatory
auctions are approximately efficient if there are many bidders with relatively
small demands bidding for a homogenous good. We study a setting where a large
bidder competes against a continuum of small bidders, and show that the small
bidders prefer the uniform-price over the discriminatory auction, the large bidder
has the reverse ranking, and there is no clear efficiency or revenue ranking
between the two formats.
4 - A Truthful-in-expectation Mechanism for the Generalized
Assignment Problem
Salman Fadaei, Technische Universität Mönchen, Munich,
Germany,
salman.fadaei@tum.de, Martin Bichler
We propose a truthful-in-expectation, 1-1/e-approximation mechanism for the
generalized assignment auction. In such an auction, each bidder has a knapsack
valuation function. We present a novel convex optimization program for the
problem which yields an MIDR allocation rule. We show how to implement the
convex program in polynomial time using a fractional greedy algorithm which
approximates the optimal solution within an arbitrarily small error.
MB29
29-Room 406, Marriott
Joint Session Analytics/MIF/HAS:
Healthcare Analytics
Sponsor: Analytics
Sponsored Session
Chair: Shannon Harris, Katz Graduate School of Business,
241 Mervis Hall, Pittsburgh, PA, 15213, United States of America,
sharris@katz.pitt.edu1 - Optimal Staffing of Revenue Centers in Healthcare Delivery
Organizations
Jerome Niyirora, SUNY Polytechnic Institute,
100 Seymour Rd, Utica, NY, 13502, United States of America,
jerome.niyirora@gmail.com, Jamol Pender
In the operations management literature, little attention is paid to profitability in
healthcare delivery organizations. But such an important issue cannot be
overlooked since an unprofitable organization is unlikely to meet the quality of
service demands. To address this issue, we introduce a nonstationary queueing
model and apply optimal control theory to derive a new closed form square root
staffing formula to allow for optimal staffing based on the cost-to-revenue ratio.
2 - Managing Customer Arrivals in Service Systems with
Multiple Servers
Christos Zacharias, Visiting Assistant Professor, University of
Miami, School of Business Administration, Miami, FL, United
States of America,
czacharias@miami.edu, Michael Pinedo
We analyze a discrete multi-server queueing model for scheduling customer
arrivals in service systems with parallel servers. Theoretical and heuristic
guidelines are provided for the effective practice of appointment overbooking to
offset no-shows. The benefits of resource-pooling are demonstrated in containing
operational costs and increasing customer throughput.
3 - A Hierarchical Bayes Model of No-show Rates
Joseph Johnson, Associate Professor, University of Miami,
5250 University Drive, 501 Kosar Epstein Building,
Coral Gables, FL, 33146, United States of America,
jjohnson@bus.miami.edu, Yu Tang, Yutian Li
Patient no-shows in US clinics can sometimes shoot up to 80%. Accurate
predictions of no-shows help clinics optimally schedule appointments. We
develop a Hierarchical Bayes logit model which improves prediction accuracy
over the widely-used simple logit model. The accuracy gain arises from the
individual patient-level coefficients provided by the Bayesian method.
Comparison of model fit on 12-months of appointment data shows that the
Bayesian model vastly outperforms the simple logit model.
4 - Appointment Scheduling with No-shows and Cancellations
Shannon Harris, Katz Graduate School of Business, 241 Mervis
Hall, Pittsburgh, PA, 15213, United States of America,
sharris@katz.pitt.edu,Jerrold H. May, Luis Vargas
Appointment no-shows and cancellations can be disruptive to clinic operations.
Scheduling strategies such as overbooking or overtime slot assignments can assist
with mitigating these disruptions. We propose a scheduling model that accounts
for both no-show and cancellation rates, and show properties of optimal
scheduling models under specific conditions.
MB30
30-Room 407, Marriott
Practice Presentations by INFORMS Roundtable
Companies I
Sponsor: INFORMS Practice
Sponsored Session
Chair: Stefan Karisch, Digital Aviation Optimization & Value Strategy,
Boeing Commercial Aviation Services, 55 Inverness Drive East,
Englewood, CO, 80112, United States of America,
stefan.karisch@jeppesen.com1 - Optimization Models for Planning and Dispatch in Large-scale
Freight Operations
Ted Gifford, Distinguished Member Of Technical Staff,
Schneider National, Inc., P.O. Box 2545, Green Bay, WI, 54306,
United States of America,
GiffordT@schneider.comSchneider National operates a fleet of 13,000 tractors and 48,000 trailers in a
random network and accepts 10,000 customer orders daily. In order to maximize
revenue and asset utilization, Schneider current employs math programming
models providing real-time decision support for load acceptance, asset re-
positioning and dispatch assignment. We will describe a number of these models
and the operational challenges that accompany them, as well as enhancements
and new models in development.
2 - Management Science at Bank of America Merrill Lynch
Russ Labe, Director, Analytics & Modeling, Bank of America,
1500 Merrill Lynch Drive, First Floor, Pennington, NJ, 08534,
United States of America,
russ.labe@bankofamerica.comThis paper will discuss the impact of analytics at Bank of America Merrill Lynch.
Russ Labe, Director of Analytics & Modeling, will provide an overview of his
group and discuss a few examples of business issues, analytic approaches, and
results. He will also discuss how OR/MS drives business benefits, lessons learned,
and best practices.
3 - Predictive Modeling at Scale
Kathy Lange, Sr. Director, Global Analytics Practice, SAS,
SAS Campus Drive, Cary, NC, 27518, United States of America,
Kathy.Lange@sas.comMost organizations realize that analytics can help them become more competitive,
more profitable, save money, or improve lives. Now they need to expand their
analytical impact. This presentation will discuss new capabilities that address how
organizations can scale up the modeling process, how to generate many hundreds
or thousands of predictive models simultaneously, automating the creation and
management of models.
MB30