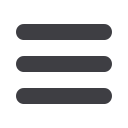

INFORMS Philadelphia – 2015
177
2 - Handling Negative Curvature in Gradient Methods for
Unconstrained and Bound Constrained Optimization
Wei Guo, Lehigh University, 200 W Packer Ave, Bethlehem, PA,
18015, United States of America,
weg411@lehigh.edu,
Frank E. Curtis
A gradient-descent method is proposed for unconstrained and bound constrained
optimization. Emphasis is placed on techniques for computing appropriate step
sizes when negative curvature is present. It extends Barzilai-Borwein two-point
step size method, its variants and gradient projection method for unconstrained
and bound constrained optimization. Global convergence is guaranteed under
mild assumptions. Numerical results illustrate the benefits of the method in the
presence of non-convexity.
3 - A Trust Region Algorithm with a Worst-case Iteration Complexity
of O(eps^(-3/2))
Mohammadreza Samadi, Lehigh University, 200 West Packer,
Bethlehem, PA, 18015, United States of America,
mos213@lehigh.edu, Daniel Robinson, Frank E. Curtis
We present a trust region method for unconstrained nonconvex optimization that
is able to drive the norm of the gradient of the objective below a prescribed
threshold eps > 0 after at most O(eps^(-3/2)) iterations, while maintaining
standard global and fast local convergence guarantees through employing
modified step acceptance criteria and a novel trust region updating mechanism.
We also present ideas for the constrained case and show numerical results.
4 - A Modified DC Algorithm for Solving Linear Programs with
Equilibrium Constraints
Francisco Jara-Moroni, Northwestern University, 2145 Sheridan
Road, Room C210, Evanston, Il, 60208, United States of America,
franciscojaramoroni2013@u.northwestern.edu,Jong-Shi Pang,
Andreas Waechter
We propose a method for finding local optima of linear programs with
equilibrium constraints. The complementarity restriction is handled by a penalty
term that can be expressed as the difference of convex functions. The
reformulated problem is solved to optimality by the difference-of-convex
functions algorithm with some variations exploiting the specific structure of the
penalization.
MB16
16-Franklin 6, Marriott
Trends in Optimization
Sponsor: Optimization/Linear and Conic Optimization
Sponsored Session
Chair: Angelia Nedich, University of Illinois, Urbana, IL,
United States of America,
angelia@illinois.edu1 - Convex Optimization for Low Rank Models
Madeleine Udell, Postdoctoral Fellow, Caltech, CMS,
Mail Code 9-94, Pasadena, CA, 91125, United States of America,
madeleine.udell@gmail.comMany of the most popular methods for unsupervised learning can be formulated
as a kind of bi-convex optimization problem which we call a low rank model,
including nonnegative matrix factorization, matrix completion, sparse and robust
PCA, and k-means. In this talk, we discuss three approaches to fitting low rank
models: alternating minimization, stochastic gradient methods, and methods
based on convex relaxations.
2 - Resolution of Misspecified Constrained Optimization Problems
via Augmented Lagrangian Schemes
Hesam Ahmadi, Student, Pennsylvania State University, 107
Holerman Hall, University Park, PA, 16802,
United States of America,
ahmadi.hesam@gmail.com,Necdet Serhat Aybat, Uday Shanbhag
We consider a misspecified optimization problem that requires minimizing of a
convex function f(x; y) in x over constraint set represented by h(x; y)=0 where y
is an unknown (or misspecified) vector but can be learnt by a distinct learning
problem. We develop joint first-order augmented Lagrangian scheme for
computing x while learning y. Iteration complexity analysis is provided when
penalty parameters are constant.
3 - Convex and Stochastic Optimization under Indirect Observations
Niao He, Georgia Tech, Atlanta, GA
United States of America,
nhe6@gatech.eduWe examine convex optimization problems involved with misspecified
parameters or distributions, while only a limited number of indirect observations
are available. We establish safe and computationally tractable approximations of
these problems and equip them with efficient algorithms. We show that in several
important cases, the estimates yielded by our approach exhibit consistency and
sublinear convergence. We also demonstrate the efficiency of our approach
through several examples.
MB17
17-Franklin 7, Marriott
Cliques and Clique Relaxations
Sponsor: Optimization/Network Optimization
Sponsored Session
Chair: Sergiy Butenko, Texas A&M University, 4037 ETB,
College Station, TX, United States of America,
butenko@tamu.edu1 - Characterizing and Detecting Independent Union of Cliques
Zeynep Ertem, Texas A&M, 2400 Central Park Ln, Apt. 407,
College Station, TX, United States of America,
zeynepertem@tamu.edu, Sergiy Butenko, Yiming Wang
This paper introduces maximum independent union of cliques problem for which
maximum clique and maximum independent set solutions are lower bound. We
present structural properties as well as complexity results. We show IP
formulation, B&B algorithm and the heuristic approaches.
2 - The Maximum S-stable Cluster Problem
Chitra Balasubramaniam, Texas A&M University, College Station,
TX, United States of America,
bcvaidyanath@tamu.edu,
Sergiy Butenko
We introduce a new clique relaxation model called s-stable cluster, that restricts
the size of the independent set found in the cluster, and is applicable in areas that
employs data mining techniques. In particular, the structural properties of the
model will be explored, and exact algorithms for solving the maximum s-stable
cluster problem will be presented.
MB18
18-Franklin 8, Marriott
Big Data Analytics: Methodology and Applications
Cluster: Modeling and Methodologies in Big Data
Invited Session
Chair: Danica Xiao, PhD Candidate, University of Washington -
Seattle, 3900 Northeast Stevens Way, Seattle, WA, 98195,
United States of America,
xiaoc@uw.edu1 - “Big Data” in Bioenergy Supply Chain
Shiyang Huang, Iowa State University, 0076 Black Engineering,
Ames, IA, 50011, United States of America,
shuang@iastate.edu,Guiping Hu
We study the development of bioenergy and its supply chain, which consists of
large numbers of independent stakeholders and sophisticated spatial and
environmental information. We introduce “Big data” optimization methodologies
into the study to mine the hidden information that can help in promoting the
bioenergy production.
2 - Learning from Business Transactions Data
Sunil Kothari, Researcher, Hewlett-Packard, 1501 Page Mill Road,
Bldg 2U, Palo Alto, CA, 94304, United States of America,
sunil.kothari@hp.com,Zhijun Yin, Gary Dispoto, Jun Zeng,
Gregory Player
In a contractual environment, the engagement starts with the sales process and
continues through the contract period generating valuable data across the life
cycle of the contract period. HP’s managed print services business generates
transaction data covering the entire life cycle of a contract. We apply the machine
learning techniques to counter the notion that price alone determines the
win/loss outcome for a deal and predict the win/loss probabilities for deals
currently in the pipeline.
3 - An Integer Programing Formulation of Training Set Selection
Walter Bennette, Research Engineer, Air Force Research
Laboratory, 26 Electronic Parkway, Rome, NY, 13441,
United States of America,
wdbennette@gmail.comAspects of a data set can make building a useful classifier difficult. Training Set
Selection (TSS) addresses some of these aspects by selecting a subset of the data in
such a way that learning from the reduced data set leads to a better model. This
work utilizes an integer programming formulation of TSS that relies on column
generation to obtain an improved selection of training instances. We then inform
current state of the art genetic algorithms for TSS with our formulation.
MB18