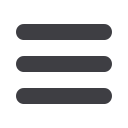

INFORMS Philadelphia – 2015
182
MB31
31-Room 408, Marriott
Data Mining and Predictive Analytics in Health Care
Sponsor: Data Mining
Sponsored Session
Chair: Lior Turgeman, Data Mining and Operations Research, Joseph
M. Katz Graduate School of Business, Roberto Clemente Dr, Pittsburgh,
PA 1526, Pittsburgh, United States of America,
tur.lior@gmail.com1 - Predicting Hospital Readmission using Patient Encounter Data
Atish Sinha, Professor, University of Wisconsin-Milwaukee,
Lubar School of Business, Milwaukee, WI, 53201-0742,
sinha@uwm.edu, Amit Bhatnagar, Arun Sen
Under the Affordable Care Act, readmission rate has become a critical issue for
hospitals. We analyze patient encounter data, obtained from an HIE, during a
two-year period for a chain of hospitals. Our model incorporates two sets of
factors, consumer demographics and encounter data, to predict readmission
likelihoods and durations.
2 - A Mixed-ensemble Predictive Model for Hospital Readmission
Lior Turgeman, Data Mining and Operations Research, Joseph M.
Katz Graduate School of Business, Roberto Clemente Dr,
Pittsburgh, PA 1526, United States of America,
tur.lior@gmail.com, Jerrold H. May, Johnson Moore,
Youxu Cai Tjader
We present a novel approach for predictive modeling, using a mixed-ensemble
classifier. The approach integrates a C5.0 tree as the base ensemble classifier, and
a support vector machine (SVM) as a secondary classifier. By implementing our
method for predicting hospital readmission of CHF patients, we were able to
overcome some of the limitations of both C5.0 and SVM, as well as to increase the
classification accuracy for the minority class, particularly when strong predictors
are not available.
3 - A Decision Analytic Approach to Modeling Heart
Transplant Survival
Asil Oztekin, Assistant Professor Of Operations & Information
Systems, Participating Faculty Of Biomedical Engineering &
Biotechnology Program, University of Massachusetts Lowell,
One University Ave. Southwick 201D, Lowell, MA, 01850,
United States of America,
Asil_Oztekin@uml.edu,Ali Dag,
Fadel Megahed
Due to the scarcity of donor hearts for transplantation, an accurate prediction of
transplantation success plays an important role in the matching procedure
between donors and recipients. A decision analytic framework based on Bayesian
Belief Network is deployed here to address this issue. The results indicate that this
decision analytic methodology yields superior results than the ones in the
transplantation
literature.Itis a generic model which can be implemented in other
transplant cases.
MB32
32-Room 409, Marriott
Big Data Analytics in Genomics
Cluster: Big Data Analytics in Computational Biology/Medicine
Invited Session
Chair: Michael Hoffman, Scientist, Assistant Professor, Princess
Margaret Cancer Centre/University of Toronto, Toronto Medical
Discovery Tower 11-311, 101 College St, Toronto, ON, M5G 1L7,
Canada,
michael.hoffman@utoronto.ca1 - A Spectral Approach for the Integration of Functional Genomics
Annotations for Genetic Variants
Iuliana Ionita-laza, Assistant Professor, Columbia University, 722
West 168 St, New York, NY, 10032, United States of America,
ii2135@columbia.eduOver the past few years, substantial effort has been put into the functional
annotation of variation in human genome sequence. Such annotations can play a
critical role in identifying putatively causal variants among the abundant natural
variation that occurs at a locus of interest. The main challenges in using these
various annotations include their large numbers, and their diversity. I will discuss
an unsupervised approach to derive an integrative score of these diverse
annotations.
2 - Big Data Regression and Prediction in Functional Genomics
Weiqiang Zhou, Johns Hopkins University Bloomberg School of
Public Health, 615 N Wolfe Street, Rm E3638, Baltimore, MD,
21205, United States of America,
kenandzhou@hotmail.com,
Hongkai Ji
The rapid growth of functional genomic data makes it possible to build models for
predicting one high-throughput genomic data type from another data type. This
can be formulated as a challenging big data regression problem which involves
fitting millions of high-dimensional regressions simultaneously. To cope with the
high dimensionality and heavy computation, we developed BIRD algorithm that
leverages the correlation structure in the data to make computation fast and
predictions accurate.
3 - Semi-automated Human Genome Annotation using
Chromatin Data
Michael Hoffman, Scientist, Assistant Professor, Princess Margaret
Cancer Centre/University of Toronto, Toronto Medical Discovery
Tower 11-311, 101 College St, Toronto, ON, M5G 1L7, Canada,
michael.hoffman@utoronto.caSegway is an integrative method to identify patterns from multiple functional
genomics experiments. It discovers joint patterns in multiple genomic datasets
using a dynamic Bayesian network model, simultaneously segmenting the
genome and identifying clusters of similar segments. We apply Segway to
ENCODE ChIP-seq and DNase-seq data and identify patterns associated with
transcription start sites, gene ends, enhancers, and repressed regions.
4 - Identifying Genetic Risk Factors for Complex Traits using
Functional and Association Data
Jo Knight, Centre for Addiction and Mental Health, 250 College
Street, Toronto, Canada,
jo.knight@camh.ca,Mike Barnes,
Mike Weale, Sarah Gagliano
Our aim is to identify the genetic risk variants that contribute to disease. Genome
wide association studies have identified some but many remain unknown. We
seek to combine the association data with functional characteristics of the
genome. Machine learning is used to derive a score to indicate whether a genetic
variant is likely to be causal based on large amounts of functional data. We
combine the functional score and the association score together in a Bayesian
framework.
MB33
33-Room 410, Marriott
Joint Session HAS/MSOM-Healthcare:
Health Care Operations
Sponsor: Health Applications
Sponsored Session
Chair: Tolga Tezcan, Associate Professor, London Business School,
Regent’s Park, London, UK, NW14SA, United Kingdom,
ttezcan@london.eduCo-Chair: Nicos Savva, London Business School, Park Road,
London, NW14SA, United Kingdom,
nsavva@london.edu1 - Why is Big Data Underutilized?
Kraig Delana, London Business School, Regent’s Park, London,
NW1 4SA, United Kingdom,
kdelana@london.edu,Nicos Savva,
Tolga Tezcan
The advent of big data has brought the opportunity to track customer needs and
offer service proactively. Motivated by a healthcare application we develop a
queueing and game theoretic model to show that enrolling in such a data-
tracking scheme generates positive externalities in the form of reduced waiting
times. Nevertheless, we show that self-interested consumers will under-utilize
this opportunity, leading to a welfare loss.
2 - A Two-sided Mechanism to Coordinate the Influenza Vaccine
Supply Chain
Kenan Arifoglu, University College London, Gower Street,
London, WC1E 6BT, United Kingdom,
k.arifoglu@ucl.ac.ukRational consumer behavior and uncertain yield lead to frequent supply/demand
mismatches in the influenza (flu) vaccine supply chain. To eliminate the
inefficiency in the flu vaccine supply chain, we propose a two-sided mechanism
which implements tax/subsidy payments on the demand side and a transfer
payment on the supply side and aligns consumers’ and vaccine manufacturer’s
incentives with the social optimum. The two-sided mechanism improves social
welfare significantly.
3 - Analysis of Triage Systems in Emergency Departments
Ozlem Yildiz, Simon Business School , University of Rochester,
CSH 4-333. Simon Business School, Rochester, NY, 14627,
United States of America,
oyildiz@london.edu, Tolga Tezcan,
Michael Kamali
We study triage method decisions in emergency departments and provide a policy
for determining when to apply provider triage (PT) based on operational and
financial considerations using a queueing framework. We obtain closed-form
expressions for the range of arrival rates in which PT economically outperforms
traditional nurse triage using a steady-state many-server fluid approximation. We
show via simulation experiments that the proposed policy performs within 0.82%
of the best solution.
MB31