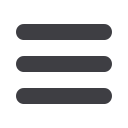

INFORMS Philadelphia – 2015
179
3 - Examining Change in Hospital Quality and Efficiency after ACA
using Dynamic Network DEA
Yasar Ozcan, Professor, Virginia Commonwealth University,
P.O. Box 980203, Richmond, VA, 23298-0203,
United States of America,
ozcan@vcu.edu, Jaya Khushalani
Dynamic Network DEA was used to examine change in both quality and
efficiency of hospitals between 2009 and 2013, pre and post Affordable Care Act
(ACA). Quality and efficiency improved significantly with no trade-off between
the two. Urban and teaching hospitals were less likely to improve quality and
efficiency together.
4 - Robust Decisions for the Partially Diversified Disease
Management Model
Shuyi Wang, Lehigh University, 200 W Packer Ave,
Bethlehem, PA, United States of America,
shw210@lehigh.eduWe discuss a model to help pharmaceutical companies determine the optimal
strategy under high uncertainty for a business model called the Partially
Diversified Disease Management Model, which includes disease care pathways as
well as health management, diagnostics&devices, and medication, and
incentivizes patients’ health. Our MIP provides a tradeoff between diversification
and specialization.
5 - When is the Outside Care Utilization Optimal for Acos?
Trade-off Between Cost, Access, and Quality
Tannaz Mahootchi, Postdoctoral Research Associate,
Northeastern University, 360 Huntington Ave, Boston, MA,
02115, United States of America,
t.mahootchi@neu.eduAccountable Care Organizations (ACOs) are responsible for the health outcomes
and the care expenses of their patients. We investigate the details of patient
diversion process to an alternative provider when the primary ACO is
experiencing congestion. ACOs choose the alternative provider based on the
performance measures and the costs of patient diversion. We derive the transfer
price and the performance measures that makes the diversion decision optimal.
MB22
22-Franklin 12, Marriott
Learning and Random Graphs
Sponsor: Applied Probability
Sponsored Session
Chair: Marc LeLarge, INRIA-ENS, 23 Avenue d’Italie, Paris, France,
marc.lelarge@ens.fr1 - Typical Distances in Directed Random Graphs
Mariana Olvera-Cravioto, Associate Professor, Columbia
University, New York, NY, 10027, United States of America,
mo2291@columbia.eduWe study the distance between two randomly selected nodes in a directed
configuration model under the assumption that the degree distributions have
finite variance. In particular, we show that the distance grows logarithmically in
the size of the graph. The method of proof uses a coupling between a graph
exploration process and a weighted branching tree, since unlike the undirected
case, we need to keep simultaneous control of both the in-degrees and the out-
degrees.
2 - Competitive Contagion in Networks
Moez Draief, Imperial College London and Huawei Research
Paris, South Kensington Campus, London, United Kingdom,
moez.draief@huawei.comThere has been a growing interest, over the past few years, in studying models of
competing products/opinions on social networks. The question of interest is what
is the impact of the first adopters of a product on the outcome of a series of
adoption by other nodes in the system influenced by those initial nodes. More
precisely, the decision of a node to adopt a product is influenced by the behaviour
of its neighbours in the social network. This raises challenging and intriguing
mathematical, algorithmic and game theoretic questions. In this talk, I will
present an overview of recent developments in this topic.
3 - Learning in Networks: Multi-armed Bandits with Structure
Richard Combes, Assistant Professor, Centrale-Supelec, Plateau de
Moulon, 3 rue Joliot-Curie, Gif-Sur-Yvette, 91192, France,
richard.combes@supelec.frThe design of networks and online services can often be mapped to a multi-armed
bandit problem with structure. With this approach, problems such as link
adaptation, resource allocation, or ad-display optimization can be solved in a
provably optimal manner. Namely, the learning speed of the proposed schemes
matches a fundamental limit verified by any scheme. A review of the relevant
mathematical tools and litterature is provided.
4 - Community Detection with the Non-backtracking Operator
Marc LeLarge, INRIA-ENS, 23 Avenue d’Italie, Paris, France,
marc.lelarge@ens.fr,Charles Bordenave, Laurent Massoulie
Community detection consists in identification of groups of similar items within a
population. In the context of online social networks, it is a useful primitive for
recommending either contacts or news items to users. We will consider a
particular generative probabilistic model for the observations, namely the so-
called stochastic block model and prove that the non-backtracking operator
provides a significant improvement when used for spectral clustering.
5 - Rumor Source Obfuscation
Peter Kairouz, Graduate Research Assistant, University of Illinois
at Urbana Champaign, 408 E Clark St, Apt. 6, Champaign, IL,
61820, United States of America,
kairouz2@illinois.edu,
Sewoong Oh, Pramod Viswanath
Anonymous messaging platforms have recently emerged as important tools for
sharing one’s thoughts without the fear of being judged by others. Such platforms
are crucial in nations with authoritarian regimes where the right to free
expression depends on anonymity. Existing messaging protocols are vulnerable
against adversaries who can collect metadata. We introduce a novel messaging
protocol and show that it spreads the messages fast and achieves perfect
obfuscation of the source.
MB23
23-Franklin 13, Marriott
Role of Information in Large-scale Stochastic
Resource Allocation Problems
Sponsor: Applied Probability
Sponsored Session
Chair: Kuang Xu, Stanford University, Stanford, CA,
United States of America,
kuangxu@stanford.edu1 - Centralized Seat Allocation for Engineering Colleges in India
Yash Kanoria, Assistant Professor, Columbia University, New
York, NY, United States of America,
ykanoria@columbia.eduThe central government funds over 75 engineering colleges in India with 50,000
seats a year, and a diversity of programs and admissions criteria. We deploy a
new, centralized, seat allocation mechanism, that accounts for the preferences of
students as well as the admissions criteria for different colleges/programs using a
deferred acceptance inspired approach.
2 - Learning to Optimize via Information-directed Sampling
Daniel Russo, Stanford University, 218 Ayrshire Farm Lane,
Apt. 102, Stanford, CA, 93405, United States of America,
djrusso@stanford.edu, Benjamin Van Roy
We offer a fresh, information-theoretic, perspective on the
exploration/exploitation trade-off and propose a new algorithm—information-
directed sampling—for a broad class of online optimization problems. We
establish a general expected regret bound and demonstrate strong simulation
performance for the widely studied Bernoulli, Gaussian, and linear bandit
problems. Simple analytic examples show information-directed sampling can
dramatically outperform Thompson sampling and UCB algorithms.
3 - Online Advertising Matching in the Large Market
Jian Wu, Cornell University, Ithaca, NY, United States of America,
jw926@cornell.edu, Peter Frazier, J. G. Dai
We study online advertising matching in a large market asymptotic regime, in
which the number of opportunities and the number of advertisers increase
simultaneously. We develop a matching policy based on the LP solution to a
certain deterministic problem. Under certain conditions, we prove that the policy
is asymptotically optimal under the fluid-scaling to maximize click-through-rate
(CTR) while satisfying all contractual agreements with overwhelming probability.
4 - Robust Scheduling in a Flexible Fork-join Network
Yuan Zhong, Columbia University, 500 W. 120th Street,
New York, NY, 10027, United States of America,
yz2561@columbia.edu,Ramtin Pedarsani, Jean Walrand
We consider a general flexible fork-join processing network, motivated by
applications in e.g., cloud computing, manufacturing, etc, in which jobs are
modeled as directed acyclic graphs, and servers are flexible with overlapping
capabilities. A major challenge in designing efficient scheduling policies is the lack
of reliable estimates of system parameters. We propose a robust scheduling policy
that does not depend on system parameters, and analyze its performance
properties.
MB23