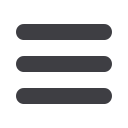

INFORMS Philadelphia – 2015
178
4 - Applications of Big Data Summarization through Polyhedral
Uncertainty Sets
Anushka Chandrababu, Research Scholar, IIITB, 26/C, Electronic
City, Bangalore, India,
anushka.babu@iiitb.org, Prasanna Gns
We present our works of summarizing structured or unstructured big data into
polyhedral uncertainty sets, orders of magnitude smaller than the original data
using a generalized multi-dimensional German tank method. Relational algebraic
operations to check disjointness, subset or intersecting relationships between such
polyhedral objects can be performed. We show the results of such big data
summarization using real world data to solve specific business needs.
5 - Assessing Demand Trends using Real Time Order
Transaction Data
Parvaneh Jahani, University of Louisville, 781 Theodore Burnett
Ct., Apt. 2, Louisville, KY, 40217, United States of America,
p0jaha01@louisville.edu, Suraj Alexander
Assessing demand trends using real time order transaction data is essential aspect
of warehouse management system. Selecting the method of demand forecasting
differs for different demand trends. We propose a new approach for classification
of Stock Keeping Units (SKUs) demand trends using Control Charts Pattern
Recognition (CCPR). After demand trend class recognition, the best method of
forecasting is selected. Bootstrapping method is used for forecasting intermittent
demand time series.
6 - Unsupervised Ensemble, or Consensus Clustering, Consists in
Finding the Optimal Combination Strategy
Ramazan Ünlö, University of Central Florida, 12100 Sterling
University Ln, Apt. 2-2419, Orlando, FL, United States of
America,
ramazanunlu@gmail.comUnsupervised ensemble, or Consensus clustering, consists in finding the optimal
combination strategy of individual clusterings that is robust with respect to the
selection of the algorithmic clustering pool. In this paper, we propose a weighting
policy for this problem that is based on internal clustering quality measures and
compare against other popular approaches.
MB19
19-Franklin 9, Marriott
OR and AI
Sponsor: Computing Society
Sponsored Session
Chair: Scott Sanner, Asst. Professor, Oregon State University, 1148
Kelley Engineering Center, Corvallis, OR, 97331,
United States of America,
ssanner@gmail.com1 - Pruning in Decision Diagrams for Optimization
Christian Tjandraatmadja, Carnegie Mellon University, 5000
Forbes Ave, Pittsburgh, PA, 15213, United States of America,
ctjandra@andrew.cmu.edu, Willem-jan Van Hoeve
Many enumerative techniques to solve discrete optimization problems benefit
greatly from using bounds to prune the search tree. We study the application of
pruning strategies to decision diagrams, which can be viewed as a compact form
of enumeration trees. In particular, we discuss how pruning strategies can be
incorporated in relaxed and restricted decision diagrams to obtain improved
primal and dual bounds.
2 - Concise Representation of Near-optimal Solutions with
Decision Diagrams
Thiago Serra, Carnegie Mellon University, 5000 Forbes Avenue,
Pittsburgh, PA, 15213, United States of America,
tserra@cmu.edu,John Hooker
Decision diagrams have recently been used to compactly encode sets of solutions
to discrete optimization problems. In this talk we study Sound Decision Diagrams
(SDDs), which encode near-optimal solutions along with worse feasible and
infeasible solutions. We provide a formal characterization of SDDs and algorithms
to find those with minimum size. Empirical results show that SDDs are smaller
than conventional decision diagrams representing the same near-optimal solution
set as its gap increases.
3 - Stochastic Optimization of the Scheduling of a
Radiotherapy Center
Antoine Legrain, Polytechnique Montreal, C.P. 6079,
Succursale Centre-ville, Montreal, QC, H3C 3A7, Canada,
antoine.legrain@polymtl.ca,Marie-andrée Fortin, Nadia Lahrichi,
Louis-Martin Rousseau, Marino Widmer
Radiotherapy centers can improve their efficiency by optimizing the utilization of
the linear accelerators. We propose an online method to schedule patients on
such machines taking into account their priority, the maximum waiting time, and
the preparation of this treatment (dosimetry). We have implemented a genetic
algorithm and a constraints program, which schedule the dosimetry. This
approach ensures the beginning of the treatment on time and thus avoids the
cancellation of treatment sessions.
MB20
20-Franklin 10, Marriott
Decision Analytics in Cloud
Cluster: Cloud Computing
Invited Session
Chair: Yue Tan, The Ohio State University, 1971 Neil Ave, Columbus,
OH, 43210, United States of America,
tanyue01@gmail.com1 - Cyber Vulnerability Maintenance Policies for Universities
Chengjun Hou, Graduate Research Associate, The Ohio State
University, 1971 Neil Ave., Columbus, Oh, 43210, United States
of America,
hou.91@buckeyemail.osu.edu, Theodore Allen
The case study application of Markov decision processes and generalizations to a
real world University policy design problem are described. Related mathematical
issues are briefly explored. The derived policy includes incentives for not
continuing the use of vulnerable software. The magnitude of saving in dollars is
estimated.
2 - throughput Scalability of Fork-join Queueing Networks
with Blocking
Yun Zeng, The Ohio State Univesity, 1971 Neil Ave, Columbus,
OH, United States of America,
zeng.153@buckeyemail.osu.edu,
Augustin Chaintreau, Don Towsley, Cathy Xia
With emerging applications such as cloud computing and big data analytics,
modern information networks are growing increasingly complex. A critical issue
concerns the throughput performance as the system expands to large scale. This
paper models the distributed information processing systems as fork-join
queueing networks with blocking. We present necessary and sufficient conditions
to ensure throughput scalability. Algorithms to check these features for given
networks are proposed.
3 - Data-driven Decision Making via Adaptive Control for Cyber
Password Management
Yue Tan, The Ohio State University, 1971 Neil Ave, Columbus,
OH, 43210, United States of America,
tanyue01@gmail.com,Cathy Xia
Cyber attacks have been widely recognized as a major international and domestic
cyber security threat. Although an increasing number of high technology
mechanisms have been developed, passwords remain as the frontline against
cyber attacks both for personal and organizational security settings in cloud
services. In this talk, we present a data-driven adaptive control framework that
converges to the optimal password expiry duration which balances between
accounts safety and user experience.
MB21
21-Franklin 11, Marriott
Re-Designing the (US) Healthcare System
Sponsor: Health Applications
Sponsored Session
Chair: Aurelie Thiele, Lehigh University, 200 W Packer Ave,
Bethlehem, PA, 18015, United States of America,
aut204@lehigh.edu1 - Designing Narrow Network Plans for Healthcare:
A Bi-objective Optimization Approach
Victoire Denoyel, ESSEC Business School, Avenue Bernard
Hirsch, Cergy, 95000, France,
victoire.denoyel@essec.edu,Aurelie Thiele, Laurent Alfandari
We build a quantitative decision model for healthcare payers willing to offer
Narrow Network (NN) plans to customers. NN have received significant attention
in the implementation of the Affordable Care Act. A payer selects a limited list
among all possible providers, and steers patients to these providers by limiting
coverage to this list. Our research question is: how to select a limited number of
providers so as to reduce the cost for the payer without decreasing the utility for
customers?
2 - The Effects of Ambulatory Surgery Centers on Hospital’s
Financial Performance
Cheng Wang, Lehigh University, 621 Taylor Street,
Department of Economics, Bethlehem, PA, 18015,
United States of America,
chw410@lehigh.eduAmbulatory surgery centers (ASCs) , which treat surgical patients who do not
need an overnight stay, are a health care service innovation that has proliferated
in the U.S. in the past four decades. This paper examines the effect of ASCs on the
net patient revenues and total operating costs of hospitals. Overall, results suggest
that ASCs are competitors to general hospitals.
MB19