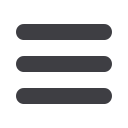

INFORMS Philadelphia – 2015
212
3 - Towards Multi-resource Fairness in Big Data Systems
Zhenhua Liu, Assistant Professor, Stony Brook University,
Stony Brook, NY, 11794, United States of America,
zhenhua.liu@stonybrook.eduBig data systems nowadays involve multiple resources such as CPU, memory,
network during multiple stages. On the other hand, these systems are usually
shared among multiple tenants with different demand characteristics. How to
optimally align these two complexities while maintaining fairness among tenants
has significant theoretical challenges, while generates great practical value. In this
talk, I will briefly introduce our recent progress along this.
MC21
21-Franklin 11, Marriott
Pierskalla Award Finalists
Sponsor: Health Applications
Sponsored Session
Chair: Mohsen Bayati, Assistant Professor, Stanford Graduate School of
Business, 655 Knight Way, Stanford, CA, United States of America,
bayati@stanford.eduCo-Chair: Soo-Haeng Cho, Associate Professor, Carnegie Mellon
University, 5000 Forbes Ave, Pittsburgh, PA, 15213,
United States of America,
soohaeng@andrew.cmu.edu1 - Pierskalla Award Finalists
Mohsen Bayati, Assistant Professor, Stanford Graduate School of
Business, 655 Knight Way, Stanford, CA, United States of
America,
bayati@stanford.edu, Soo-Haeng Cho, Joel Goh
The Health Applications Society of INFORMS sponsors an annual competition for
the Pierskalla Award, which recognizes research excellence in the field of health
care management science. The award is named after Dr. William Pierskalla to
recognize his contribution and dedication to improving health services delivery
through operations research. The Pierskalla award information can be found on
the website at:
https://www.informs.org/Community/HAS/Pierskalla-AwardMC22
22-Franklin 12, Marriott
Message Passing for Inference
Sponsor: Applied Probability
Sponsored Session
Chair: Jinwoo Shin, Korea Advanced Institute of Science and
Technology, 291 Daehak-ro, Yuseong-gu, Daejeon, Korea, Republic of,
jinwoos@kaist.ac.kr1 - How Hard is Inference for Structured Prediction?
David Sontag, Assistant Professor, NYU, 715 Broadway,
12th Floor, Room 1204, New York, NY, 10003,
United States of America,
dsontag@cs.nyu.eduStructured prediction tasks in machine learning involve the simultaneous
prediction of multiple labels. This is typically done by maximizing a score function
on the space of labels, which decomposes as a sum of pairwise terms, each
depending on two specific labels. Although marginal and MAP inference for these
models are NP-hard in the worst-case, approximate inference algorithms are often
remarkably successful. In this talk, we develop a theoretical framework to explain
why.
2 - Tractable Graphical Modeling and the Bethe Approximation
Tony Jebara, Professor, Columbia University, 500 West 120 St.,
Room 450, Mail Code 0401, New York, NY, 10027,
United States of America,
jebara@cs.columbia.eduWe consider three NP-hard graphical modeling problems. For maximum a
posteriori inference, we identify the limits of tractability via perfect graph theory.
For marginal inference, we provide efficient solutions using Bethe free energy
approximations and discretization. For learning, we combine Bethe with a Frank-
Wolfe algorithm to avoid intractable partition functions. Applications include link
prediction, social influence estimation, computer vision, financial networks and
power networks.
3 - Lifts of Graphs and Approximate Inference
Nicholas Ruozzi, Assistant Professor, UT Dallas, 2601 N. Floyd Rd.
MS EC31, Richardson, TX, 75080, United States of America,
nicholas.ruozzi@utdallas.eduThe approximate maximum a posteriori inference problem (MAP) for graphical
models over finite state spaces is an NP-hard problem in general. As a result,
approximate MAP inference techniques based on convex relaxations are often
employed in practice. These convex relaxations are relatively well-understood in
the discrete case but many open questions remain in the continuous setting. I will
discuss how to extend many of the discrete results to the continuous setting using
lifts of graphs.
4 - Factor Graphs, Kramers-Wannier Duality, and the Sum-product
Algorithm
Ali Al-Bashabsheh, Postdoc, The Chinese University of Hong
Kong, Hong Kong, Hong Kong - PRC,
entropyali@gmail.com,
Pascal O. Vontobel
A key object associated with a graphical model is its partition function. Although
the partition function is often intractable, it can be estimated (e.g., via the sum-
product algorithm) or analyzed (e.g., via factor graph transforms). An example of
the latter, and also the main focus of this talk, is the analysis of 2D-Ising models
via Kramers—Wannier duality. At various places we will point out connections to
optimization problems.
MC23
23-Franklin 13, Marriott
Optimal Control of Stochastic Systems
Sponsor: Applied Probability
Sponsored Session
Chair: Jiheng Zhang, HKUST, Clear Water Bay, Hong Kong, Hong Kong
- PRC,
j.zhang@ust.hk1 - Distributionally Robust Inventory Control when Demand
is a Martingale
Linwei Xin, Assistant Professor, University of Illinois at
Urbana-Champaign, 104 S. Mathews Ave., Urbana, IL, 61801,
United States of America,
lxin@illinois.edu, David Goldberg
Independence of random demands across different periods is typically assumed in
multi-period inventory models. In this talk, we consider a distributionally robust
model in which the sequence of demands must take the form of a martingale
with given mean and support. We explicitly compute the optimal policy and
value, and shed light on the interplay between the optimal policy and worst-case
martingale. We also compare to the analogous setting in which demand is
independent across periods.
2 - Join the Shortest Queue with Customer Abandonment
Ping Cao, University of Science and Technology of China, Room
707A, School of Management, Hefei, China,
pcao@ustc.edu.cn,
Junfei Huang
We consider an overloaded queueing system with many servers and customer
abandonment under the join-the-shortest-queue policy. Diffusion approximations
for system performances are established. The approximation expressions depend
on the traffic intensity: in some cases a one-dimensional Ornstein-Uhlenbeck
process is enough while in other cases a two-dimensional process is necessary. We
also compare the results with that of the one-global-queue system.
3 - Asymptotic Optimal Control of Perishable Inventory
Jiheng Zhang, HKUST, Clear Water Bay, Hong Kong,
Hong Kong - PRC,
j.zhang@ust.hk, Rachel Zhang, Hailun Zhang
We study joint replenishment and clearance of perishable products when the
demand rate is large. We proposes two policies based on fluid and diffusion
approximations, respectively. The fluid based policy can achieve asymptotic
optimality with the gap explicitly computed. The diffusion based policy can
significantly improve the gap when the initial inventory is small. When the initial
inventory is large, we prove that depletion-once is enough to achieve asymptotic
optimality.
MC24
24-Room 401, Marriott
Network Modeling and Analysis
Sponsor: Artificial Intelligence
Sponsored Session
Chair: Junming Yin, University of Arizona, Department of MIS, Tucson,
AZ, 85721, United States of America,
junmingy@email.arizona.edu1 - Analysis of Network Experiments with Nonnegative
Treatment Effects
David Choi, Carnegie Mellon University, 5000 Forbes Avenue,
Pittsburgh, United States of America,
davidch@andrew.cmu.eduRandomized experiments in network settings are potentially useful for
understanding the effects of peer influence and other social mechanisms.
However, the analysis of experiments is an open problem when the individuals in
the experiment are assumed to be able to influence each other’s decisions. We
propose a new method that requires much weaker assumptions than existing
methods, which often impose stylized models of individual behavior that may not
be valid in practice.
MC21