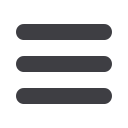

INFORMS Philadelphia – 2015
215
3 - Engineering Technician Location Analytics
Dwayne Otis, Air Products and Chemicals, 7201 Hamilton
Boulevard, Allentown, PA, 18195, United States of America,
otisdk@airproducts.comIn this talk we present a model whose objective is to determine, within a given
physical region, the near-optimal required number of technicians and their
assigned home base locations in order to satisfy defined customer service levels.
Ideally, the workload for each technician would be balanced and the total cost to
meet the desired service level is minimized. Furthermore, we examine additional
areas where this general technique could be applied.
MC31
31-Room 408, Marriott
Data Mining in Healthcare
Sponsor: Data Mining
Sponsored Session
Chair: Ramin Moghaddass, Assistant Professor, University of Miami,
1251 Memorial Dr, Coral Gables, FL, 33146, United States of America,
ramin@miami.edu1 - Influential Neighbor Analysis with a Hierarchical Bayesian Model
Ramin Moghaddass, Assistant Professor, University of Miami,
1251 Memorial Dr, Coral Gables, FL, 33146,
United States of America,
ramin@miami.edu, Cynthia Rudin
For a doctor with enough experience, almost every patient would have
similarities to key cases seen in the past and each new patient could be viewed as
a mixture of important pieces of these key past cases. Because doctors often tend
to reason this way, an efficient computationally aided diagnostic tool might be
helpful in locating key past cases of interest that could assist with diagnosis. We
develop a model to mimic the type of logical thinking that physicians use when
considering past cases.
2 - Falling Rule Lists
Fulton Wang, MIT, 5 Cambridge Center #792, Cambridge, MA,
02142, United States of America,
fultonwang@gmail.com,Cynthia Rudin
Falling rule lists are classification models consisting of an ordered list of if-then
rules, where (i) the order of rules determines which example should be classified
by each rule, and (ii) the estimated probability of success decreases monotonically
down the list. These kinds of rule lists are inspired by healthcare applications
where patients would be stratified into risk sets and the highest at-risk patients
should be considered first.
3 - Machine Learning for Clinical Decision Support for Heart
Failure(HF) Readmission
Wei Jiang, PhD Student, Johns Hopkins University,
6606 Copper Ridge Drive Apt. 201, Baltimore, MD, 21209,
United States of America,
wjiang1990@gmail.com,Sean Barnes,
Matthew Toerper, Scott Levin, Eric Hamrock, Sauleh Siddiqui,
Stephanie Cabral
Predicting risk of HF readmission have gained increasing attention.Previous
studies mainly used administrative data. We will focus on using clinical data from
EMR for predicting HF readmission by creating structured data from unstructured
clinical data and combining it with administrative data. Then we use classification
models such as random forest and support vector machine for predicting purpose.
In the end, we will demonstrate the value of clinical data in predicting HF
readmission.
4 - Or’s of and’s for Interpretable Machine Learning:
Prediction and Explanation
Tong Wang, Graduate Student, MIT, 70 Pacific Street, apt 242A,
Cambridge, MA, 02139, United States of America,
tongwang@mit.edu,Cynthia Rudin
We present a form of interpretable models for data prediction and explanation.
The model is comprised of a small number of disjunctions of conjunctions (or’s of
and’s). We apply OA models to solve two machine learning tasks, classification
and causal effect analysis. We formed two integer linear programs to construct the
optimal OA models that can achieve good performance and interpretability, by
incorporating regularizations on the sparseness. We show that regularizations
reduce computation.
MC32
32-Room 409, Marriott
Statistical Innovations in Computational Biology
and Genomics
Cluster: Big Data Analytics in Computational Biology/Medicine
Invited Session
Chair: Mingyao Li, Statistical Innovations in Computational Biology
and Genomics, University of Pennsylvania, 213 Blockley Hall, 423
Guardian Drive, Philadelphia, PA, 19104, United States of America,
mingyao@mail.med.upenn.edu1 - Prediction using Multinomial Inverse Regression in
Microbiome Studies
Hongzhe Li, Professor of Biostatistics, University of Pennsylvania,
215 Blockley Hall, Philadelphia, PA, United States of America,
hongzhe@mail.med.upenn.eduNext-generation sequencing technologies allow 16S rRNA gene surveys or
metagenome shotgun sequencing in order to characterize taxonomic composition.
The data can be summarized as k-mer counts. We consider the regression problem
for for such high dimensional in order to build a model for predicting the clinical
outcomes based on microbiome data and demonstrate its applications.
2 - Computational Validation of NGS Variant Calls using
Genotype Data
Margaret Taub, Assistant Scientist, Johns Hopkins Department of
Biostatistics, 615 N Wolfe St, E3527, Baltimore, MD, United
States of America,
mtaub@jhsph.edu, Suyash Shringarpure,
Ingo Ruczinski, Rasika Mathias, Kathleen Barnes
We performed a comparison of different variant calling algorithms on 642
samples whole-genome sequenced to an average depth of 30x, focusing on
characteristics of variants called by different subsets of callers. We developed a
classifier which uses genotyping array data, often collected for all sequenced
individuals, as a gold standard to improve calibration of variant calls. We found
little difference in quality between single- and multi-sample calling methods at
30x coverage.
MC33
33-Room 410, Marriott
Topics in Health Systems
Sponsor: Health Applications
Sponsored Session
Chair: Douglas King, University of Illinois at Urbana-Champaign,
117 Transportation Bldg., 104 S. Mathews Ave., MC-238, Urbana, IL,
61801, United States of America,
dmking@illinois.edu1 - Methods in Treatment Planning with Continuous Dose Delivery
Kimia Ghobadi, Massachusetts Institute of Technology, 77
Massachusetts Ave, Cambridge, MA, United States of America,
kimiag@mit.edu,David Jaffray, Dionne Aleman, Caroline Chung
In this work we investigate continuous dose delivery models and algorithms for
head-and-neck patients. We discuss the necessary changes and considerations in
the optimization models and algorithms for different tumour sites. We will also
present the clinical realization of the plans and compare the obtained clinical
results with the simulated treatment plans.
2 - Optimal Timing of Living-donor Liver Transplantation
under Risk-aversion
Ozlem Cavus, Bilkent University, Department of Industrial
Engineering, Ankara, 06800, Turkey,
ozlem.cavus@bilkent.edu.tr,
Umit Emre Kose, Oguzhan Alagoz, Andrew J. Schaefer
The timing of liver transplantation is crucial as it affects the quality and the length
of the patients’ life. Previous studies used risk-neutral Markov Decision Processes
to optimize the timing of the transplantation. In this study, we model the risk-
averse behavior of the patients using coherent dynamic measures of risk. We
obtain optimal policies for different patients and donated organs. We also derive
the structural properties of the optimal policy. Supported by TUBITAK [Grant
213M442].
MC33