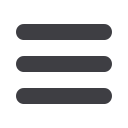

INFORMS Philadelphia – 2015
292
TB24
24-Room 401, Marriott
Urban Data Analytics and Mining
Sponsor: Artificial Intelligence
Sponsored Session
Chair: Xun Zhou, Assistant Professor, University of Iowa, S210
Papajohn Business Building, 21 E Market Street, Iowa City, IA, 52242,
United States of America,
xun-zhou@uiowa.edu1 - A Data Mining Approach to the Discovery of Emerging Hotspot
Patterns in Urban Data
Amin Vahedian Khezerlou, University of Iowa, S283
Pappajohn Business Building, The University of Iowa,
Iowa City, IA, 52242-1994, United States of America,
amin-vahediankhezerlou@uiowa.edu,Xun Zhou
Emerging hotspots can be observed in urban data, e.g., cellular service or traffic
congestions due to non-periodic events (e.g., sport games, accidents). Efficiently
identifying these patterns help city planners and service provides response early
to the congestions. Previous hotspot detection techniques focused on patterns
with fixed footprints. We propose an efficient data mining approach to detect
emerging congestion patterns with dynamic footprints and validate our method
on real urban data.
2 - Exploiting Geographic Dependencies for Real Estate Ranking
Yanjie Fu, Rutgers University, 504 N 5th St, Harrison, NJ, 07029,
United States of America,
yanjie.fu@rutgers.edu, Hui Xiong,
Hui Xiong
We propose a geographic method, named ClusRanking, for estate evaluation by
leveraging the mutual enforcement of ranking and clustering power model the
geographic dependencies of estates for enhancing estate ranking. Indeed, the
geographic dependencies of the investment value of an estate can be from the
characteristics of its own neighborhood (individual dependency), the values of its
nearby estates (peer dependency), and the prosperity of the affiliated latent
business area (zone dependency).
3 - A General Geographical Probabilistic Factor Model for Point of
Interest Recommendation
Bin Liu, Rutgers University, 900 Davidson Road, 47 Nichols
Apartment, Piscataway, NJ, 08854, United States of America,
binben.liu@rutgers.eduThe problem of point of interest recommendation is to provide personalized
places. The decision process for a user to choose a POI can be influenced by
numerous factors, such as personal preferences, geographical considerations, and
user mobility behaviors. We propose a general geographical probabilistic factor
model framework which takes various factors into consideration. Extensive
experimental results show promise of the proposed methods.
TB25
25-Room 402, Marriott
Software-Driven Innovation and Business Strategies
Sponsor: Information Systems
Sponsored Session
Chair: Narayan Ramasubbu, University of Pittsburgh,
354 Mervis Hall, Pittsburgh, PA, 15228, United States of America,
nramasubbu@katz.pitt.edu1 - Business Value of the Mobile Enterprise: An Empirical Study of
Mobile Sales Force in Banking
Ajit Sharma, Ross School of Business, 701 Tappan Street,
Ann Arbor, MI, United States of America,
asharmaz@umich.eduThe press and research on mobility has remained focused on the customer-firm
interface. While there is sufficient evidence of gains from mobile marketing in
better targeting and lift, the benefits of mobile-centric enterprise processes remain
under-studied. In this paper, we empirically assess the reduction in process time
and error rates by shifting from a traditional “sales person in the field-computer in
the office” sales process to a “sales person in the field with a tablet” sales process.
2 - Lost in Cyberspace: An Investigation of Digital Borders, Location
Recognition, and Experience Attribution
Brian Dunn, Assistant Professor, University of Oklahoma, 307
West Brooks Ste. 307D, Norman, OK, 73072, United States of
America,
bkdunn@ou.edu, Narayan Ramasubbu, Dennis Galletta,
Paul Lowry
Do website users know where they are? Given that they may visit multiple sites
in the same session, they may not, which has important implications for the
owners of those sites. However, past research has yet to account for this
possibility. To understand when users recognize where they are online and how
they attribute credit to the sites that are helpful to them, we introduce the
concepts of ‘digital borders’ and ‘border strength’ and use them in an
experimental investigation.
3 - Design Control in Open Innovation: An Examination of Open
Source Software Production
Shivendu Pratap Singh, University of Pittsburgh, Room 229,
Mervis Hall, Pittsburgh, 15260, United States of America,
shs161@pitt.eduFirms are opting for co-creating software, by attracting developers on platforms
like GitHub.This shared model of development requires flexible software design
controls to influence community engagement, which could result in proliferation
of design options. Flexible design control policy could have side effects such as
accumulation of technical debt and need to be judiciously managed. This paper
examines the antecedents and consequences of design control policies in social
software production.
4 - Time-dependent Pricing for Mobile Data: Analysis, Systems,
and Trial
Soumya Sen,
ssen@umn.edu,Carlee Joe-wong, Mung Chiang,
Sangtae Ha
Dynamic pricing of mobile data traffic can alleviate network congestion by
creating temporally-varying price discounts. But realizing it requires developing
analytical models for price point computation, systems design, and field
experiments to study user behavior. In this paper, we present the architecture,
implementation, and a user trial of a day-ahead time-dependent pricing.
TB26
26-Room 403, Marriott
Retailer Pricing
Cluster: Operations/Marketing Interface
Invited Session
Chair: Kathy Stecke, UT Dallas, SM30 JSOM, 800 W Campbell Rd,
Richardson, TX, 75080, United States of America,
kstecke@utdallas.eduCo-Chair: Xuying Zhao, University of Notre Dame, Notre Dame, IN,
Xuying.Zhao.29@nd.edu1 - Optimal Price Trajectories and Inventory Allocation for Inventory
Dependent Demand
Stephen Smith, Professor, Santa Clara University, 500 El Camino
Real, Lucas Hall 216H, Santa Clara, CA, 95053-0382, United
States of America,
ssmith@scu.edu, Narendra Agrawal
Retail demand is often inventory dependent because larger inventories create
more attractive displays and low inventories can create broken assortments. This
research jointly optimizes the price trajectory and the allocation of a given
amount of inventory across a set of non-identical stores with inventory
dependent demands.
2 - The Effect of Reward Purchase on Dynamic Pricing
Hakjin Chung, Stephen M. Ross School of Business,
University of Michigan, Ann Arbor, MI, United States of America,
hakjin@umich.edu,So Yeon Chun, Hyun-soo Ahn
In many loyalty programs, consumers are provided with an option to acquire
products by redeeming loyalty points instead of cash. We characterize when
consumers use points or cash depending on their willingness-to-pay in cash as
well as in points. Then, we incorporate this consumer choice model into the
seller’s dynamic pricing model, where the revenues from both posted price and
reimbursement for reward sales are embedded in each period.
3 - An Off-price Retailer with Two Ordering Opportunities
Moutaz Khouja, Professor, UNC Charlotte, 9201 University City
Blvd, Charlotte, NC, 28223, United States of America,
mjkhouja@uncc.edu, Jing Zhou
We develop a model of an off-price retailer who has two procurement
opportunities for next season. The first opportunity occurs after the end of the
current season where she buys excess inventory from retailers and manufacturers
and store them until next season. The second opportunity occurs before the
selling season begins again. The product quantity available in the first opportunity
is limited while the price in the second opportunity is a random variable that
depends on consumer demand.
4 - Multi-product Price Promotions with Reference Price Effects
Kevin Li, UC Berkeley, IEOR Department, Berkeley, CA, 94720,
United States of America,
kbl4ew@berkeley.edu, Candace Yano
We consider a retailer’s problem of setting prices, including promotional prices,
over a multi-period horizon for multiple products with correlated demands,
considering customer stockpiling and the effect of reference prices on customers’
buying behavior. These factors limit the efficacy of deep discounts and frequent
promotions. We present structural results and numerical examples that provide
insight into the nature of optimal policies and the impact of various parameters.
TB24