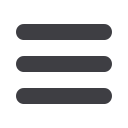

INFORMS Philadelphia – 2015
290
2 - Computational Study of a Second Order Cone Relaxation for
Binary Quadratic Polynomial Problems
Julio Goez, Postdoctoral Fellow, Ecole Polytechnique Montreal
and GERAD, 2900 Boulevard Edouard-Montpetit, Montréal, QC,
H3T 1J4, Canada,
jgoez1@gmail.com,Miguel Anjos
This work presents a computational study of the second order cone relaxation for
binary quadratic problems proposed by Ghaddar, Vera and Anjos (2011) who
used a polynomial optimization approach. We explore how this relaxation can be
strengthened using additional constraints, and also, we explore the relation of
disjunctive conic cuts with this relaxation.
3 - Computational Approaches to Mixed Integer Second Order
Cone Optimization
Aykut Bulut, PhD Candidate, Lehigh University, 200 W. Packer
Ave., Bethlehem, PA, 18015, United States of America,
aykut@lehigh.edu, Ted Ralphs
We introduce an open-source Mixed Integer Second Order Cone Optimization
(MISOCO) solver. We present computational experiments on various approaches
to solve MISOCO problem. We test using outer approximation method to solve
continuous relaxations. We also discuss using various valid inequalities to
improve the continuous relaxations. We discuss computational performance of
these approaches on conic benchmark library (CBLIB 2014) problems.
4 - Solving Robust Portfolio Optimization Problems in Practice
Sarah Drewes, Senior Consultant, Dr., MathWorks, Adalperostr.
45, Ismaning, Germany,
Sarah.Drewes@mathworks.deRobust versions of the Markowitz mean-variance model can reduce the
estimation risk induced by its sensitivity to changes in expected returns or the
covariance matrix. Probabilistic versions of the classical model can be formulated
as nonlinear and often second order cone programs. We study how to solve these
problems also by general nonlinear solvers (MATLAB Optimization Toolbox) and
in case of discrete variables. We evaluate both computational performance and
complexity of implementation.
TB17
17-Franklin 7, Marriott
Network Modeling and Design
Sponsor: Optimization/Network Optimization
Sponsored Session
Chair: Mario Ventresca, Assistant Professor, School of Industrial
Engineering, Purdue University, 315 N Grant St, Lafayette, IN, 47906,
United States of America,
mventresca@purdue.edu1 - Action-based Network Models
Viplove Arora, Graduate Student, School of Industrial
Engineering, Purdue University, 45 N Salisbury St, Apt. 9,
West Lafayette, IN, 47906, United States of America,
arora34@purdue.edu,Mario Ventresca
Complex networks are very useful representations of real world complex systems.
A number of network generation procedures have been proposed that are capable
of producing networks with a restricted subset of structural properties. However,
a unifying model remains elusive. We present initial results on an action-based
perspective that has potential to yield more general network structures than
existing techniques. A machine learning approach to learn a probabilistic model
will be presented.
2 - Automatically Inferring Complex Network Models
Mario Ventresca, Assistant Professor, School of Industrial
Engineering, Purdue University, 315 N Grant St., Lafayette, IN,
47906, United States of America,
mventresca@purdue.eduComplex networks are becoming increasingly important across many disciplines.
However, the problem of network modeling is extremely intricate and time
consuming. Hence, frameworks have been proposed to estimate model
parameters, but are focused on capturing a small subset predefined network
characteristics such as degree distribution. I will present recent work on a highly
robust automated inference approach that is able to discover arbitrary network
models with minimal human insight.
3 - Cut-set Separation Schemes for the Robust Single-commodity
Network Design Problem
Daniel Schmidt, Carnegie Mellon University, 720 S Negley Ave,
Pittsburgh, PA, 15232, United States of America,
schmidtd@cmu.edu,Chrysanthos Gounaris
We address the exact solution of the Robust Single-Commodity Network Design
Problem in which customer demands are uncertain and realize from within
anappropriately defined uncertainty polytope. We explore techniques to
approximate the arc-flow based formulation with fewer variables. We also
evaluate the use of meta-heuristics for the NP-hard problem of separating cut-set
inequalities in the context of a branch-and-cut solution approach.
4 - Network Design Problem for Battery Electric Bus
Yousef Maknoon, EPFL, Route Cantonale, Lausanne, Switzerland,
yousef.maknoon@epfl.ch, Shadi Sharif Azadeh, Michel Bierlaire
In electric bus planning, for battery installation, we need to investigate two
points: (1) the type and location of charger stations (2) the capacity of battery of
each bus. In this presentation, first we describe the problem and the design
elements. Then, we present its mathematical form followed by the resolution
approach. Finally, we demonstrate the computational results on our case study
and discuss about the robustness of the plan.
TB18
18-Franklin 8, Marriott
Data Mining for Different Type of Big Data
Cluster: Modeling and Methodologies in Big Data
Invited Session
Chair: Young-seon Jeong, Chonnam National University,
Department of Industrial Engineering, Gwangju, Korea, Republic of,
youngseonjeong@gmail.com1 - Classification of Uncertain Data using Group to Object Distances
Behnam Tavakkol, PhD Candidate, Rutgers University,
5200 BPO Way, Piscataway, NJ, 08854, United States of America,
btavakkol66@gmail.com, Myong K (MK) Jeong, Susan Albin
Uncertain data problems have features represented by multiple observations or
their fitted PDFs. We propose two approaches for classifying uncertain data
objects. The first uses existing Probabilistic Distance Measures for object-to-object
distances and classifies with KNN. The second features a new probabilistic
distance measure for object to class distances.
2 - The Classification Methodology of Chip Quality using Canonical
Correlation Analysis
Ki-hyun Kim, Samsung Electronics Co., Banwol-dong,
Hwaseong-si, Gyeonggi-do, Korea, Republic of,
bluenamja@daum.netIn this study, we proposed classification methodology using a canonical
correlation analysis as feature selection method at multi-dimensional chip level
data generated in the semiconductor manufacturing industry. As the result of this
research, we were able to extract important varialbes in the varous PCM variables
from the correlation of the multiple FBC variables and PCM variables. The
proposed method was improved the accuracy of quality classification for a chip
tested in the probe test.
3 - Multivariate Monitoring for Metal Fabrication Process in Mobile
Devices Manufacturing
Seonghyeon Kang, M.s. Candidate, Korea University, Innovation
Hall 817, Korea University, 145 Anam-ro, Seongbuk-gu, Seoul,
136-713, Korea, Republic of,
shyeon.kang@gmail.com,
Seoung Bum Kim
In mobile industry, using metal case of devices is rapidly increased for thin and
attractive design. However, fabricating metal is the difficult process because
accurate control of equipment are required. In this study, we propose an efficient
multivariate monitoring procedure to observe more than 40 parameters of metal
fabrication equipment. The effectiveness of the proposed procedure is
demonstrated by real data from the mobile plant in one of the leading mobile
companies in South Korea.
4 - Multivariate Monitoring of Automated Material Handling Systems
in Semiconductor Manufacturing
Sangmin Lee, Korea University, Innovation Hall 817, Korea
University, 145 Anam-ro, Seongbuk-gu, Seoul, 136-713, Korea,
Republic of,
smlee5679@gmail.com,Seoung Bum Kim
Monitoring all possible contingencies in automated material handling system
(AMHS) of semiconductor manufacturing is a difficult task because tremendous
hardware and software systems are involved. This study presents an efficient
multivariate monitoring procedure to monitor more than 100 KPIs in AMHS. The
effectiveness and applicability of the proposed procedure is demonstrated by real
data from semiconductor fabrication plant in one of the leading semiconductor
companies in South Korea.
5 - Quantifying the Level of Risk of Functional Chips in
Semiconductor Wafers
Young-seon Jeong, Chonnam National University, Department of
Industrial Engineering, Gwangju, Korea, Republic of,
youngseonjeong@gmail.com,Byunghoon Kim,
Seung-hoon Tong, Inkap Chang, Myong K (MK) Jeong
This talk presents the procedure to quantify the level of risk of functional chips in
dynamic random access memory (DRAM) wafers. To screen risky functional
chips, the risk level of each chip is estimated by the posterior probability for
functional chips. The functional chips closer to the class of defective chips may
have a higher probability of being failed in the near future. The experimental
results by using real-life wafers show the effectiveness of the proposed method.
TB17