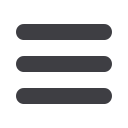

INFORMS Philadelphia – 2015
295
2 - Contact Center Qualifying Transfer Rate Modeling and Analysis
Jie Yu, Operations Research Scientist, University of Phoenix,
3137 E Elwood St, Phoenix, AZ, 85034, United States of America,
jie.yu@phoenix.edu, Roger Gung, Lin Wang
Impact analysis on transferring marketing inquiries to qualifying leads for
potential enrollments and the performance of contact center agents are crucial to
contact center and enrollment operations. An impact analysis was conducted on
transfer rate with drivers including speed to lead, lead source, time of request as
well as program level. A mixed effect logistic regression model was built to rank
agents’ performance in terms of expected transfer rate with given marketing
inquiries. The model was also employed to evaluate the impact of reducing
contact center and enrollment operating hours.
3 - Enrollment Service Contact Strategy Optimization
Pan Hu, Operations Research Scientist, University of Phoenix,
3137 E Elwood St, Phoenix, AZ, 85034, United States of America,
pan.hu@phoenix.edu, Yun Ouyang, Jie Yu, Lin Wang,
Roger Gung
This project is to study how contact behaviors of enrollment representatives
influence enrollment progression of higher education pursuers. To better serve
the needs of potential students, it is critical to communicate effectively by
bringing up right topics in the best timing. We examined a list of conversation
topics suggested in the internal guideline of University of Phoenix for enrollment
representatives, and identified the best contact strategy using statistical models.
TB33
33-Room 410, Marriott
Joint Session HAS/MSOM-Healthcare: Modeling
Applications for Emergency Departments
Sponsor: Health Applications
Sponsored Session
Chair: Sean Barnes, University of Maryland, 4352 Van Munching Hall,
University of Maryland, College Park, MD, 20742,
United States of America,
sbarnes@rhsmith.umd.edu1 - Review of Queueing Theory Applied to Emergency Departments
with Comparable Simulation Studies
Summer (Xia) Hu, PhD Student, University of Maryland,
Department of Mathematics, College Park, United States of
America,
xhu64@umd.edu, Sean Barnes, Bruce Golden
Queueing Theory (QT) is an important tool for Emergency Department (ED)
design and management. By reviewing all papers with ED QT analysis or
applications since 1972, this survey examines the contributions of QT to modeling
EDs and identify its benefits and limitations when compared to discrete-event
simulation (DES) under similar ED operational settings. Our results indicate that
the combination of queueing and DES methods can be a powerful approach to
better ED modelling.
2 - Using Simulation to Assess the Impact of an Observation Unit in a
Pediatric Emergency Department
Mark Grum, University of Michigan, 1205 Beal Avenue, Ann
Arbor, MI, 48109, United States of America,
mgrum@umich.edu,Gabriel Zayas-Caban, Michelle Macy, Allison Cator, Amy Cohn
Observation units (OUs) provide an alternative disposition decision for ED
patients who may benefit from further observation, such as those are not ill
enough to be admitted, but not well enough to be discharged. Patients can be
placed in an OU for monitoring, diagnostic evaluation, and/or treatment prior to
disposition. In this talk, we discuss our approaches (e.g. simulation) for assessing
the impact of an OU in the Pediatric ED at the University of Michigan.
3 - Operational Causes of Patients Leaving Before Treatment is
Completed in Emergency Departments
David Anderson, Assistant Professor, Baruch,
davidryberganderson@gmail.com,Bruce Golden, Edward Wasil,
Laura Pimentel, Jon Mark Hirshon
Patients leaving before treatment (LBTC) is completed is an indicator of poor
Emergency Department performance. Contrary to previous research, volume is
not the main driver of patients leaving before treatment is complete. First
provider time and lengths of treatment are much more strongly associated with
LBTC rate. We show that operational factors such as treatment time and staffing
decisions play a role in waiting time and, thus, in determining the LBTC rate.
4 - Strategies for Ebola Containment: A Biological-behavioral-
operational Modeling Decision Framework
Eva Lee, Georgia Tech, Atlanta, GA,
eva.lee@gatech.eduThis work is joint with CDC. We present a computational decision modeling
framework that integrates an agent-based biological disease spread model, a
dynamic network-based social-behavior model that captures human behavior and
interaction, and a stochastic queueing model that describes treatment
characteristics, day-to-day hospital and homecare processes, and resource usage.
An optimization engine determines the minimum resource needed to contain the
Ebola epidemic in W. Africa.
TB34
34-Room 411, Marriott
Data-driven Modeling and Analysis of Health
Care Systems
Sponsor: Health Applications
Sponsored Session
Chair: Anil Aswani, UC Berkeley, 4141 Etcheverry Hall, Berkeley, CA,
94720-1777, United States of America,
aaswani@berkeley.edu1 - Constructing Behavioral Models for Personalized Weight Loss
Interventions using Integer Programming
Yonatan Mintz, Graduate Student, UC Berkeley, 1822 Francisco
St., Apt. 10, Berkeley, CA, 94703, United States of America,
ymintz@berkeley.edu, Philip Kaminsky, Yoshimi Fukuoka,
Anil Aswani, Elena Flowers
In this paper we describe two (a machine learning and a utility maximization)
models for weight loss using clinical trial data. We believe these quantitative
models of behavior change can be used to provide personalized interventions,
improve adherence and lower costs of current weight loss programs. Given the
high prevalence of obesity, these results provide significant insight into more
effective approaches to implement weight loss programs.
2 - Modeling Treatment Adherence Behavior in the Treatment of
Obstructive Sleep Apnea
Yuncheol Kang, Pennsylvania State University, 236 Leonhard
Building, State College, 16801, United States of America,
kang.yuncheol@gmail.com, Paul Griffin, Vittal Prabhu,
Amy Sawyer
We target patients who suffered from Obstructive Sleep Apnea (OSA) and their
treatment behaviors when using Continuous Positive Airway Pressure (CPAP)
devices. We model underlying dynamics and patterns of patient treatment
behavior using Markov models as a basis for designing effective and economical
intervention. Also we suggest a guideline for designing a cost-effective
intervention to economically treat the patients.
3 - Inverse Optimization with Noisy Data
Auyon Siddiq, UC Berkeley, 4141 Etcheverry Hall, University of
California, Berkeley, Berkeley, 94720, United States of America,
auyon.siddiq@berkeley.edu,Zuo-jun Max Shen, Anil Aswani
We present an approach for inverse parametric optimization with noisy solution
data for convex forward problems. The proposed method yields well-behaved
estimates that attain risk consistency or parameter estimation consistency under
reasonable conditions. While the formulation is non-convex in general, we
provide an approximation algorithm that yields consistent estimates for a class of
quadratic programs. Numerical results show competitive performance with state-
of-the-art techniques.
4 - Quantifying the Resilience of Hospital Unit Management under
High Workloads
Mo Zhou, PhD Student, UC-Berkeley, 4470 Etcheverry Hall,
Berkeley, CA, 94709, United States of America,
mzhou@berkeley.edu,Anne Miller, Anil Aswani, Jason Slagle,
Daniel France
Hospital unit shifts with high admissions/discharges (ADTs) and low nurse-to-
patient ratios (NPRs) increase mortality. Nurse managers promote unit resilience,
and we quantify this using time series and network analysis of hourly phone calls,
ADTs, and NPRs over 2 years from an Intensive Care Unit. Statistical variable
selection assessed variable dependency, and time-series estimation demonstrated
the validity of phone calls as a resilience measure. Future studies will elucidate
adaptive limits.
TB34