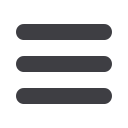

INFORMS Philadelphia – 2015
291
TB21
21-Franklin 11, Marriott
Bundled Payment Systems
Sponsor: Health Applications
Sponsored Session
Chair: Danny Hughes, Harvey L. Neiman Health Policy Institute,
1891 Preston White Drive, Reston, VA, 20191,
United States of America,
dhughes@neimanhpi.org1 - Optimizing Provider Decisions under Bundled Payments
Brenda Courtad, University of Cincinnati, 2925 Campus Green
Dr, Cincinnati, OH, 45221, United States of America,
courtabl@mail.uc.eduWhen moving from fee-for-service to bundled payments the providers focus shifts
from revenue generating to cost reducing. We develop a partially observable
Markov decision process to aid providers in deciding which interventions to
implement to reduce costs.
2 - Increasing Healthcare Value in Accountable Care Organizations
through Incentive Redesign
Christian Wernz, Virginia Tech, Industrial and Systems
Engineering, Blacksburg, VA, 24060, United States of America,
cwernz@vt.edu, Hui Zhang, Barry Barrios, Danny Hughes
ACOs are incentivized by the Centers for Medicare and Medicaid Services (CMS)
to lower costs while meeting quality standards. We determined how CMS’
incentive program can be improved, and how ACOs can optimally distribute
incentives among their members. Using multiscale decision theory, we performed
an in-depth analysis of computed tomography (CT) scanner investments and use
in ACO hospitals, calibrated the model with Medicare data, and show how CT
scan cost can be lowered and outcomes improved.
3 - Mitigating Small Provider Financial Risk under Prospective
Bundled Payment Systems
Danny Hughes, Harvey L. Neiman Health Policy Institute,
1891 Preston White Drive, Reston, VA, 20191, United States of
America,
dhughes@neimanhpi.org, Jeremy Eckhause
Retrospective bundled payment models, which cover all medical services within
an episode of care, usually include stop loss provisions to manage financial risk.
As payments shift to prospective bundled payments, the mechanisms to manage
these stop loss provisions may no longer exist. We develop nonlinear
programming models to develop pricing strategies that address the inherent
higher risk to smaller providers under such a payment system.
4 - Bundled Payments: The Roles of Organization and Diagnosis
Turgay Ayer, Georgia Institute of Technology, 765 Ferst Dr.,
Atlanta, GA, 30332, United States of America,
ayer@isye.gatech.edu,Mehmet Ayvaci, Jan Vlachy
Medicare has started contracting with healthcare providers for bundled payments.
However, most providers do not have experience with the risks and opportunities
for such payment mechanism. We propose a game-theoretic model to capture the
power dynamics between physicians and the hospital under various patient
pathways. We use the model to generate hypotheses and test these hypotheses
using real data.
TB22
22-Franklin 12, Marriott
Learning High-dimensional/ Sparse Models
Sponsor: Applied Probability
Sponsored Session
Chair: Varun Gupta, University of Chicago Booth School of Business,
Chicago, IL, United States of
AmericaVarun.Gupta@chicagobooth.edu1 - Robust Methods for High-dimensional Regression
Po-ling Loh, Assistant Professor, University of Pennsylvania, 3730
Walnut St, 466 Jon M. Huntsman Hall, Philadelphia, PA, 19104,
United States of America,
loh@wharton.upenn.eduWe discuss new methods for robust regression in high-dimensional settings. Our
procedures draw upon classical approaches in robust statistics, designed for
scenarios where the number of parameters is fixed and the sample size grows to
infinity — however, these methods may be adapted to perform robust inference
in high dimensions, as well. We also prove that the robust estimators, which
involve minimizing nonconvex functions, may nonetheless be optimized to
desirable accuracy.
2 - Statistical Guarantees for Individualized Rank Aggregation
Sahand Negahban, Yale University, 24 Hillhouse Ave,
New Haven, CT, 06510, United States of America,
sahand.negahban@yale.eduWe study a version of rank aggregation known as collaborative ranking. In this
problem we assume that individual users provide us with pairwise preferences
and from those preferences we wish to obtain rankings on items that the users
have not had an opportunity to explore. We provide a theoretical justification for
a nuclear norm regularized optimization procedure.
3 - Inference in High-dimensional Varying Coefficient Models
Mladen Kolar, Assistant Professor, Chicago Booth, 5807 South
Woodlawn Avenue, Chicago, IL, 60637, United States of America,
mkolar@chicagobooth.edu, Damian Kozbur
We focus on the high-dimensional linear varying-coefficient model and develop a
novel procedure for estimating the coefficient functions. Our procedure works in
a high-dimensional regime, under arbitrary heteroscedasticity in residuals, and is
robust to model misspecification. We derive an asymptotic distribution for the
normalized maximum deviation of the estimated coefficient function and
demonstrate how these results can be used to make inference in high-
dimensional dynamic graphical models.
4 - Elementary Estimators for High-dimensional Statistical Models
Eunho Yang, IBM T.J. Watson, P.O. Box 218, Yorktown Heights,
United States of America,
eunho@cs.utexas.eduWe propose a class of closed-form estimators for sparsity-structured high-
dimensional models. Our approach builds on observing the precise manner in
which the classical MLE breaks down under high-dimensional settings. We
provide a rigorous statistical analysis that shows that our simple estimators
recovers the same asymptotic convergence rates as those of computationally
expensive L1-regularized MLEs. We corroborate statistical performance, as well as
computational advantages via simulations.
TB23
23-Franklin 13, Marriott
New Advances in Production Planning and
Scheduling
Cluster: Stochastic Models: Theory and Applications
Invited Session
Chair: Jingshan Li, Professor, 1513 University Ave, Madison, WI,
53706, United States of America,
jli252@wisc.edu1 - Coordination in Multi-product Manufacturing Systems: Modeling
and Analysis
Cong Zhao, Research Assistant, University of Wisconsin-Madison,
1513 University Ave, Room 3235, Madison, Wi, 53706,
United States of America,
czhao27@wisc.edu, Ningxuan Kang,
Li Zheng, Jingshan Li
Multi-product systems are common in today’s manufacturing process. Effective
coordination between products in such systems is important in operation. We
study a two-product geometric manufacturing system and derive closed-form
expressions of performance measures. An optimal allocation policy of buffer
thresholds is developed, and the monotonicity of optimal buffer size with respect
to machine parameters is investigated. The managerial insights to achieve optimal
production control are discussed.
2 - Level Scheduling in Automotive Assembly Lines and its Effect on
the Consumption of Ressources
Heinrich Kuhn, Professor, Catholic University of Eichstaett-
Ingolstadt, Supply Chain Management & Operations,
Auf der Schanz 49, Ingolstadt, 85049, Germany,
heinrich.kuhn@ku-eichstaett.de,Dominik Woerner
Level scheduling approaches in sequencing of assembly lines are used as
substitutional model for the underlying economic and sustainable objectives since
a leveled distribution of materials requirements does not necessarily contribute
directly to these objectives. We conduct a case study at a major German
automotive company selecting relevant part families whose consumption is
currently unequal distributed by an extensive simulation study.
3 - A New Scenario Based Sales and Operations Planning Model
Nico Vandaele, Professor, KU Leuven, Naamsestraat 69 Box 3555,
Leuven, 3000, Belgium,
nico.vandaele@kuleuven.be,
Catherine Decouttere, Gerd Hahn, Torben Sens
We apply a scenario-based approach to the sales and operations planning process
where both model-based and non-model based Key Performance Indicators are
taken into account. This allows us to balance customer service, derived from
aggregate order lead times, and relevant costs of operations when determining
volume/mix decisions for internal and external production. An industry-derived
case example with distinct outsourcing options is used to highlight the benefits of
the approach.
TB23