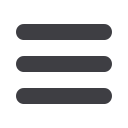

INFORMS Philadelphia – 2015
288
3 - Studying Influence of Comments in Online News Papers
Iljoo Kim, Assistant Professor, Saint Joseph’s University, 347
Mandeville Hall, 5600 City Avenue, Philadelphia, PA, 19131,
United States of America,
ikim@sju.edu,Gautam Pant
In this work, we study online comments and their influence in online news
articles. Using text-mining techniques, we attempt to explain and/or predict
influence of online newspaper comments on the context of the original article or
even on creating a new agenda through the discussions among commenters. This
is done based on the textual signals embedded within comments as well as news
articles.
4 - Politics and Information Technology Investments in
The U.S. Federal Government in 2003-2015
Min-Seok Pang, Assistant Professor, Temple University,
1810 N 13th St, Speakman 201e, Philadelphia, PA, 19122,
United States of America,
minspang@temple.eduWhat makes some US federal agencies digitally advanced and others lagging? This
study investigates how politics affects IT investment in federal agencies. With a
panel dataset from 133 federal agencies, our empirical analyses produce several
intriguing findings. A federal agency makes more capacity-building IT
investments (i) when its head is appointed with legislative approval, (ii) when the
federal government is less divided, and (iii) when it is neither too conservative
nor too liberal.
TB11
11-Franklin 1, Marriott
Machine Learning under a Modern Optimization Lens
Sponsor: Optimization/Integer and Discrete Optimization
Sponsored Session
Chair: Dimitris Bertsimas, Professor, MIT, 77 Massachusetts Ave.,
Cambridge, MA, 02139, United States of America,
dbertsim@mit.edu1 - Sparse Principal Component Analysis via a Modern
Optimization Lens
Lauren Berk, Massachusetts Institute of Technology, 77
Massachusetts Avenue, Bldg. E40-149, Cambridge, MA, 02139,
United States of America,
lberk@mit.edu, Dimitris Bertsimas
We develop tractable algorithms that provide provably optimal solutions to the
exact Sparse Principal Component problems of up to 1000 dimensions, using
techniques from Mixed Integer Optimization and first order methods. Unlike
earlier SPCA methods, our approach retains complete control over the degree of
sparsity of the components, and provides solutions with higher explained
variance.
2 - Robust Support Vector Machines
Colin Pawlowski, MIT, 77 Massachusetts Ave., Cambridge, MA,
02139, United States of America,
cpawlows@mit.edu,Dimitris Bertsimas
We consider a maximal-margin classifier which is the non-regularized
formulation of SVM. Using Robust Optimization, we develop new,
computationally tractable methods that are immunized against uncertainty in the
features and labels of the training data. Experiments on real-world datasets from
the UCI Machine Learning Repository show out-of-sample accuracy
improvements for robust methods in a significant number of problems analyzed.
3 - Optimal Trees
Jack Dunn, Operations Research Center, MIT, 77 Mass Ave,
Bldg E40-130, Cambridge, MA, 02139, United States of America,
jackdunn@mit.edu,Dimitris Bertsimas
Decision trees are widely used to solve the classical statistical problem of
classification. We introduce a new method for constructing optimal decision trees
using Mixed-Integer Optimization, and show using real data sets that these trees
can offer significant increases in accuracy over current state-of-the-art decision
tree methods. We also demonstrate the benefits of using Robust Optimization
when constructing these trees.
4 - Logistic Regression using Robust Optimization
Daisy Zhuo, Massachusetts Institute of Technology, 77
Massachusetts Avenue, Cambridge, MA, 02139,
United States of America,
zhuo@mit.edu,Dimitris Bertsimas
Logistic regression is one of the most commonly used classification methods, yet
the solution can be sensitive to inaccuracy and noise in data. Here we propose an
approach using Robust Optimization to find stable solutions under uncertainties
in data features and labels. Using more than 80 real-world problems, we
demonstrate that the robust logistic regressions lower misclassification error
significantly in the majority of the data sets.
TB12
12-Franklin 2, Marriott
Nonlinear Programming in Stochastic and
Multilevel Problems
Sponsor: Optimization/Mixed Integer Nonlinear Optimization and
Global Optimization
Sponsored Session
Chair: Alexander Vinel, Auburn University, 3301 Shelby Center,
Auburn, AL, 36849-5346, United States of America,
alexander.vinel@auburn.edu1 - Branch-and-cut Algorithm for Integer Bilevel Linear
Optimization Problems
Sahar Tahernejad, Graduate Student, Lehigh University, 12 Duh
Drive- No. 132, Bethlehem, PA, 18015, United States of America,
sat214@lehigh.edu,Ted Ralphs
We extend the branch-and-cut framework of Denegre and Ralphs for solving
integer bilevel linear optimization problems (IBLPs). IBLPs differ from standard
integer optimization problems in that there are solutions which are integer but
not feasible and they should be removed from the feasible solution set. Our
proposed algorithm applies a variety of cut generation techniques for removing
such solutions. We report on numerical experiments on some benchmark IBLPs.
2 - On Pessimistic Versus Optimistic Bilevel Linear Programs
M. Hosein Zare, University of Pittsburgh, 1048 Benedum Hall,
Pittsburgh, PA, 15261, United States of America,
moz3@pitt.edu,
Osman Ozaltin, Oleg Prokopyev
We study the relationships between Pessimistic and Optimistic Bilevel Linear
Programs. In particular, we focus on the case when the upper-level decision-
maker (i.e., the leader) needs to consider the uncertain behavior of the
lower-level decision maker (i.e., the follower). We derive some computational
complexity properties, and illustrate our results using a defender-attacker
application.
3 - Identifying Risk-averse Low-diameter Clusters in Graphs with
Random Vertex Weights
Maciej Rysz, NRC-AFRL, 1350 N. Poquito Road, Shalimar, FL,
United States of America,
mwrysz@yahoo.com, Pavlo Krokhmal
We consider the problem of finding a k-club of minimum risk contained in a
graph whose vertices have stochastic weights. A stochastic programming
framework that is based on the formalism of coherent risk measures is used to
find the corresponding subgraphs. A combinatorial branch-and-bound solution
algorithm is proposed.
4 - Solution Procedures for a Class of Mixed-integer Nonlinear
Programming Problems
Alexander Vinel, Auburn University, 3301 Shelby Center,
Auburn, AL, 36849-5346, United States of America,
alexander.vinel@auburn.edu, Pavlo Krokhmal
We study solution approaches for a class of mixed-integer non-linear
programming problems with our interest stemming from recent developments in
risk-averse stochastic programming. We explore possible applications of some of
the solution techniques that have been successfully used in mixed-integer
second-order conic programming and show how special structure of problems
under consideration can be utilized.
TB13
13-Franklin 3, Marriott
Stochastic Approximation
Sponsor: Optimization/Optimization Under Uncertainty
Sponsored Session
Chair: Raghu Pasupathy, Associate Professor, Department of Statistics,
Purdue University, 250 N University Street, West Lafayette, IN, 47907,
United States of America,
pasupath@purdue.edu1 - Budget-constrained Stochastic Approximation
Uday Shanbhag, The Pennsylvania State University, 310
Leonhard Building, University Park, PA, 16801, United States of
America,
udaybag@engr.psu.edu, Jose Blanchet
We consider a convex constrained stochastic convex optimization problem in
which the simulation budget is fixed and computation is expensive. We consider
stochastic approximation schemes in which the sample-size is either constant or
updated at every step while meeting this budget and provide suitable finite-time
error bounds.
TB11