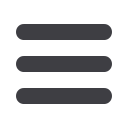

INFORMS Nashville – 2016
270
TB18
106A-MCC
Finance, Portfolio II
Contributed Session
Chair: Jonathan Liu, Stony Brook University, 61-40 163rd Street,
Flushing, NY, 11365, United States,
Jonathan.m.liu@stonybrook.edu1 - Portfolio Selection With The Probabilistic Ordered
Weighted Average
Jose M. Merigo, Full Professor, University of Chile, Av. Diagonal
Paraguay 257, Santiago, 8330015, Chile,
jmerigo@fen.uchile.cl,Sigifredo Laengle, Gino Loyola
This article presents a generalization of Markowitz’s mean-variance portfolio
selection approach by using the probabilistic ordered weighted average. The main
advantage is that it can under or overestimate the information provided in the
classical portfolio approach according to the attitudinal character of the decision
maker. Moreover, it includes the classical Markowitz model as a particular case
when the attitudinal character is not needed, by using only the expected value
which is a particular case of the probabilistic ordered weighted average. The work
considers a wide range of scenarios in financial decision making problems.
2 - Structural Approach To Portfolio Analysis With
Diversification Constraints
Kyungchan Park, PhD Candidate, Yonsei University, Seodaemun-
gu Yonsei-ro 50, School of Business (Bld.#212) Rm.#411, Seoul,
03722, Korea, Republic of,
hippogras@gmail.com,Hongseon Kim,
Seongmoon Kim
The maximum weight of single stock in mutual fund is limited by regulations to
enforce diversification. Under incomplete information with added constraints on
portfolio weights, enhanced performance had been reported in previous
researches. We use a structural approach to analyze the effects of additional
constraints on the portfolio’s performance by computing the Euclidean distance
from the tangency portfolio, as opposed to analyzing ex-post return only. We find
that the diversification-constrained portfolios generally have a smaller distance
than the unconstrained portfolios.
3 - Monetary Policy And Real Estate Price Fluctuation:
An Analysis On Regional Heterogeneity From China
Charl Chan, Tongji University, Siping Road 1500, Room 1211,
Shanghai, 200092, China,
charlc@yeah.net,Jiangang Shi
This paper analyzes empirically the relationship among monetary policy, real
estate price and policy aims using PVAR model. The results show that not all areas
of real estate market as a carrier can play a normal function in the process of
transmission from the policy tools to the ultimate goal. Extended McCallum rule
executed against the regional real estate price bubble will affect the normal
supply of the basic currency to other area. And Extended Taylor rule executed
will exacerbate deflation in the areas which real estate market is ineffective as a
carrier, while it can bring negative effects to other area economic fundamentals.
4 - Efficient Allocation Of Tarp Funds To U.s. Banks
Jonathan Liu, Stony Brook University, 61-40 163rd Street,
Flushing, NY, 11365, United States,
Jonathan.m.liu@stonybrook.edu,Herbert F Lewis, Dmytro Holod
In 2008 the government allocated hundreds of billions of dollars to banks all
across the United States under the Troubled Asset Relief Program (TARP). The
main purpose of TARP was to encourage bank lending during the financial crisis.
In this paper, we apply data envelopment analysis (DEA) as part of a two-step
process to measure the efficiency of the distribution of funds. DEA is a linear
programming-based methodology for measuring relative efficiency of decision
making units (DMU). A reallocation model is used to show how funds could have
been assigned in a more efficient manner and how additional lending could have
been achieved. Finally, we find common characteristics among the inefficient
banks.
TB19
106B-MCC
Computational Methods for Healthcare Applications
Sponsored: Computing
Sponsored Session
Chair: Burak Eksioglu, Clemson University, Clemson University,
Clemson, SC, 29634, United States,
burak@clemson.edu1 - Optimal Compromises Between Efficiency And Practicality
Andrew C Trapp, Worcester Polytechnic Institute,
atrapp@wpi.eduIn many real-world contexts, the output of an optimization model may be
impractical to implement directly due to a variety of factors. For example, an
optimized medical personnel schedule may specify such a large deviation from
the (non-optimized) status quo, that it might only be possible to roll out over
time. We discuss our methodology, TOpS (Transition toward the Optimal
Solution), that creates optimal deployment plans for binary integer programs.
TOpS illuminates the most improving steps within a specified deviation from the
status quo. We implement TOpS in two popular modeling environments, and
demonstrate how it can find the best improving steps while managing change
from present conditions.
2 - Two-stage Stochastic Programming For Vaccine
Vial Replenishment
Zahra Azadi, Clemson University,
zazadi@clemson.edu,
Harsha Gangammnavar, Sandra D Eksioglu
Multi-dose vaccine vials must be utilized within a short time if not protected in
appropriate temperature. The remaining doses are discarded. Single-dose vials do
not contribute to Open Vial Wastage (OVW), but have higher purchase and
inventory holding costs per dose than multi-dose vaccine vials. This study
presents a two-stage stochastic programming model that aids health care
practitioners identify inventory replenishment and vial opening policies which
minimize costs and OVW. We compare the performance of optimal with myopic
policies in the presence of random demand.
3 - Optimal Quarantining Policy For Ebola Epidemic
Ceyda Yaba, Clemson University,
cyaba@clemson.edu,Burak Eksioglu, Amin Khademi
The actions taken during a deadly epidemic can be crucial in order to eradicate
the disease. We model a Markov decision model for an Ebola epidemic in two
districts with the objective of minimizing incidence while quarantining infectious
people. However, as the capacities of the quarantine facilities are limited, we need
to find a decision rule for quarantining infectious population so that the incidence
is minimized in both of the districts.
TB20
106C-MCC
Robust Multiobjective Optimization for Decision
Making under Uncertainty and Conflict
Tutorial Session
Chair: Margaret M Wiecek, Clemson University, Mathematical Sciences
Dept, Clemson, SC, 29634-1907, United States,
wmalgor@clemson.edu1 - Robust Multiobjective Optimization For Decision Making Under
Uncertainty And Conflict
Margaret M Wiecek, Clemson University, Mathematical Sciences
Dept, Clemson, SC, 29634-1907, United States,
wmalgor@clemson.edu, Garrett M Dranichak
Many real-life problems in engineering, business, and management are
characterized by multiple, conflicting objectives, as well as the presence of
uncertainty. The conflicting criteria originate from various ways to assess system
performance and the multiplicity of decision makers, while uncertainty results
from inaccurate or unknown data due to imperfect models and measurements,
lack of knowledge, and volatility of the global environment. In this tutorial, the
deterministic approaches to uncertainty that are integrated with multiobjective
optimization to address decision making under uncertainty and conflict are
discussed. The approaches are based on robust optimization and parametric
optimization, both developed for single-objective settings. Six sources of
uncertainty are presented, and each type of uncertainty is placed in the
multiobjective optimization problem (MOP), yielding several types of uncertain
MOPs (UMOPs). Some of the sources are adopted from earlier studies in (single-
objective) engineering optimization, while the others result from the
multiobjective optimization modus operandi. The UMOP models are classified first
according to the location of the uncertainty in their formulation, second with
respect to the undertaken optimization approach, and third on the basis of the
proposed definition of robust efficient solutions. The models are presented along
with the accompanying results on solution concepts, properties, methods, and
applications that are specific to each case. It is expected that the topics selected in
this tutorial and their organization may help beginners to become familiar with
the area of robust multiobjective optimization while serving as a reference to
researchers.
TB18