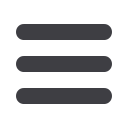

INFORMS Nashville – 2016
271
TB21
107A-MCC
Coordinated Care Delivery
Sponsored: Health Applications
Sponsored Session
Chair: Pooyan Kazemian, Harvard Medical School,
25 Shattuck Street, Boston, MA, 02115, United States,
pooyan.kazemian@mgh.harvard.eduCo-Chair: Mark P Van Oyen, University of Michigan, 1221 Beal Ave,
Ann Arbor, MI, 48109, United States,
vanoyen@umich.edu1 - The Impact Of Delay Announcements On Hospital Network
Coordination
Jing Dong, Northwestern University,
jing.dong@northwestern.edu,
Elad Yom-Tov
We investigate the impact of delay announcements on the coordination within
hospital networks using a combination of empirical observations and numerical
experiments. We provide empirical evidence that patients may take delay
information into account when choosing emergency service providers and that
such information can help increase coordination in the network. We analyze
different factors that may affect the level of coordination that can be achieved. In
particular, we show that delay estimators that are based on historical average may
cause extra oscillation in the system when patients are sensitive to delay.
2 - Proactive Inpatient Bed Allocation For Emergency Department
Patients Using Predictive Analytics
Seung Yup Lee, Wayne State University, Detroit, MI, United States,
seung.lee@wayne.edu, Ratna Babu Chinnam, Evrim Dalkiran
One of the main factors driving Emergency Department (ED) crowding is
boarding delay, where admitted patients are held in ED while waiting for an
inpatient bed to be identified and prepared. We propose a queueing network
model that allows for the development of ‘proactive’ coordination strategies. In
particular, under the proposed setting, the inpatient bed allocation process
precedes ED patient disposition. Model also accounts for the performance of the
predictive analytics model in predicting disposition decisions. We present
analytical results and insights through experiments motivated by a large Midwest
healthcare facility.
3 - Care Coordination Models Based On Longitudinal Encounter Data
Michael Rossi, Univ of Massachusetts - Amherst, Amherst, MA,
United States,
mrossi09@gmail.com,Hari Balasubramanian
We discuss a framework for analyzing data concerning healthcare encounters at
the individual level. These encounters can be of various types - outpatient,
emergency room, inpatient, pharmaceutical etc., each corresponding to one or
more diagnoses. We provide examples where such data could be used and discuss
the stochastic methods that are best suited for generating insights.
4 - Coordinating Clinic And Surgery Appointments To Meet Access
Service Levels For Elective Surgery
Pooyan Kazemian, Harvard Medical School, Boston, MA,
United States,
pooyan.kazemian@mgh.harvard.edu, Mustafa Y Sir,
Mark P Van Oyen, David Larson, Kalyan Pasupathy
Providing timely access to surgery is crucial for patients with high acuity diseases
like cancer. We present a methodological framework to coordinate clinic and
surgery appointments so that patient classes with different acuity levels can see a
surgeon in the clinic and obtain surgery (if found to be needed) within a
maximum wait time target. We evaluate six heuristic scheduling policies that
exploit information on the need for surgery obtained from the clinic visit.
Colorectal surgery at Mayo Clinic is discussed as a case study. Numerical results
suggest dramatic improvements in access for urgent patients.
TB22
107B-MCC
Data-Driven Healthcare Operations
Sponsored: Health Applications
Sponsored Session
Chair: Hessam Bavafa, University of Wisconsin, 4284C Grainger Hall,
975 University Ave., Madison, WI, 53706, United States,
hessam.bavafa@wisc.edu1 - Risk Aversion In Gatekeeping Systems: An Empirical Study Of
Admission Errors In Emergency Departments
Stefan Scholtes, University of Cambridge, Judge Business School,
Cambridge, United Kingdom,
s.scholtes@jbs.cam.ac.uk, Michael
Freeman
In a study of over 450,000 emergency department attendances, we explore the
impact of gatekeeper risk-aversion and the level of diagnostic uncertainty on
referral errors. While gatekeepers normally make binary decisions to refer or not,
we demonstrate the value of a third decision alternative, akin to a second
opinion, that can be used when the gatekeeper lacks confidence to commit. The
error reduction is particularly pronounced for more risk-averse gatekeepers, for
customers with a high level of diagnostic uncertainty, and when the unit is busy.
2 - Learning From Many: Partner Diversity And Team Familiarity In
Fluid Teams
Jonas Jonasson, London Business School,
jjonasson@london.edu,
Zeynep Aksin Karaesmen, Sarang Deo, Kamalini Ramdas
We use data from London Ambulance Service to study the impact of partner
diversity of new paramedics on their operational performance. We find that the
greater diversity in prior partners directly improves performance for an
unstandardized process. For a more standardized process, this effect is moderated
by a new recruit’s total experience. We explore the implications of our results for
team formation strategies by balancing the benefits of partner diversity with those
of team familiarity.
3 - Patient Portals In Primary Care: Impacts On Visit Frequency
And Patient Health
Hessam Bavafa, University of Wisconsin,
hessam.bavafa@wisc.eduInterest in innovative healthcare delivery models has increased due to measures
such as the Affordable Care Act, which is designed to expand insurance coverage
and contain healthcare costs. One innovation that has been forwarded as a low-
cost alternative to physician office visits is “e-visits,” or secure messaging between
patients and physicians. We evaluate the effect of e-visit adoption on patient
health and physician productivity using a panel dataset from a primary care
provider in the US.
4 - Discharge Decision In Emergency Departments: Impact Of
Operational Measures And Pay-for-Performance Incentives
Eric Park, University of Hong Kong, Hong Kong,
g,
ericpark@hku.hk, Yichuan Ding
We study how operational measures in the emergency department such as
number of patients waiting to be seen and physician’s patient load affect patient
discharge decisions. We also analyze the impact of a provincial hospital level pay-
for-performance incentive scheme on discharge decisions. We study several major
hospitals in the metro Vancouver, Canada area.
TB23
108-MCC
Models in Medical Decision Making
Sponsored: Health Applications
Sponsored Session
Chair: Zlatana Dobrilova Nenova, University of Pittsburgh,
282 Mervis Hall, Pittsburgh, PA, 15260, United States,
zdn3@pitt.edu1 - Chronic Kidney Disease: A Simulation Study
Zlatana Nenova, University of Pittsburgh,
zdn3@pitt.edu,
Jerrold H May
We developed a case-based reasoning simulation model to predict the one-year
disease progression of chronic kidney disease patients. The model bases its
projections on an analysis of the patient’s historical lab values (eGFR, albumin,
phosphate, and potassium) and vital signs (systolic and diastolic blood pressure,
and weight), together with the history of disease comorbidities and complications
(diabetes, heart failure, dialysis, PVD/CVD, and cirrhosis).
2 - Decision-making Models In Kidney Transplantation
Eric Chow, Johns Hopkins University, Baltimore, MD, 21231,
United States,
echow8@jhmi.eduClinical decision-making in kidney transplantation is a constant challenge for
patients: will you benefit from a given organ being offered or are you better off
waiting on dialysis for a better organ? The field is thus in need of mathematical
models designed to help patients make these decisions. Fortunately, there are rich
sources of big data in transplantation to support the design of these models,
including a national registry of every patient on the waiting list, every organ offer
made, and post-transplant outcomes. This presentation will review these data
sources and their integration into several existing models.
3 - Challenges In Markov Modeling Of Cancer Treatment
Jiaru Bai, University of California, Irvine, Irvine, CA, United
States,
jiarub@uci.edu,Cristina del Campo, L Robin Keller
We present a way to build a Markov decision tree to model cancer progression
and cost-effectiveness analysis for two or more cancer treatments. We propose
several problems researchers can encounter in this kind of research and provide
possible solutions.
TB23