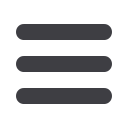

INFORMS Nashville – 2016
277
4 - Data Analysis And Experimental Design For Accelerated Life
Testing With Heterogeneous Group Effects
Kangwon Seo, PhD Candidate, Arizona State University, 699 S Mill
Ave., Tempe, AZ, 85281, United States,
kseo7@asu.edu, Rong Pan
In accelerated life tests (ALTs), complete randomization is hardly achieved
because of economic or engineering constraints. Typical experimental protocols
such as subsampling or random blocks in ALT result in a grouped structure of test
units, which leads to correlated lifetime observations. In this talk, generalized
linear mixed model (GLMM) approach is proposed to analyze ALT data and find
the optimal ALT design with consideration of heterogeneous group e ffects. First,
we will demonstrate how the random group effects of ALT affect the life-stress
relationship. Second, D-optimal ALT test plan will be derived when we run
experiments with multiple test chambers.
TB39
207A-MCC
Applied Probability and Simulation I
Sponsored: Applied Probability
Sponsored Session
Chair: Henry Lam, University of Michigan, 500 S. State Street,
Ann Arbor, MI, 48109, United States,
khlam@umich.edu1 - Rare Event Estimation For Gaussian Random Vectors
Ton Dieker, Columbia University,
dieker@columbia.edu,
Richard Gabriel Birge
We present a new technique for estimating the probability P(g(X)>x), where X is
a Gaussian random vector and g is a function for which the probability becomes a
rare event probability. In this setting, direct Monte Carlo is computationally
expensive. We establish quantitative properties on the performance of our
technique and illustrate them through numerical examples.
2 - On Adaptive Recursion For Integral Optimization
Raghu Pasupathy, Purdue University,
pasupath@purdue.eduWe consider \emph{integral optimization problems}, that is, high-dimensional
optimization problems where the objective function is expressed as an integral
that can only be approximated using numerical quadrature. For efficient
optimization, we propose an adaptive line-search recursion that dynamically
determines the extent of work to be exerted during numerical quadrature.
Assuming a general quadrature error-rate, we prove consistency and sample
complexity results. The achieved rate in all cases is \emph{optimal} in a certain
sense that we make clear.
3 - Three Asymptotic Regimes For Ranking And Selection With
General Sample Distribution
Yi Zhu, Northwestern University,
yizhu2020@u.northwestern.eduIn this paper, we study three asymptotic regimes that can be applied to ranking
and selection (R&S) problems with general sample distributions. These asymptotic
regimes are constructed by sending problem parameters (probability of incorrect
selection, difference between the best and second best system) to zero. We
establish asymptotic validity of the corresponding R&S procedures under each
regime. We also analyze the connection among different asymptotic regimes and
compare their pre-limit performances.
TB40
207B-MCC
Markov Decision Processes: Theory
Sponsored: Applied Probability
Sponsored Session
Chair: Matthew J. Sobel, Emeritus Professor, Case Western Reserve
University, 10900 Euclid Ave., Cleveland, OH, 44106-7235,
United States,
matthew.sobel@case.eduCo-Chair: Jie Ning, Case Western Reserve University, 10900 Euclid
Ave., Cleveland, OH, 44106-7235, United States,
jie.ning@case.edu1 - Atomless Discounted Markov Decision Processes With
Multiple Criteria
Eugene A Feinberg, Stony Brook University,
eugene.feinberg@stonybrook.edu, Aleksey Piunovskiy
A Markov Decision Process (MDP) is called atomless if the initial distribution and
transition probabilities are atomless. We show that, for an atomless MDP with
multiple cost functions, for an arbitrary policy there is a nonrandomized
stationary policy with the same vector of the total expected discounted costs. We
also discuss the relevance of this result to Lyapunov’s convexity theorem, to the
classic results by Dvoretzky, Wald, and Wolfowitz on sufficiency of
nonrandomized policies for atomless decision problems, and to our previous
results on sufficiency of nonrandomized Markov policies for atomless MDPs.
2 - Optimal Policies In Decentralized Stochastic Control: Existence
And Approximations
Serdar Yuksel, Queen’s University, Kingston, ON, K7L 3N6,
Canada,
yuksel@queensu.caWe will study optimal solutions in decentralized stochastic control. First, strategic
measures will be introduced; these are probability measures induced by
admissible policies. Properties such as convexity and compactness will be studied
leading to existence of and structural results for optimal policies. Finally,
asymptotic optimality of finite model representations will be established. These
lead to asymptotic optimality of quantized control policies, so that one can
construct a sequence of finite models obtained through the quantization of
measurement and action spaces whose solutions converge to the optimal cost.
Witsenhausen’s counterexample will be a running case study.
3 - Easy Affine Mdps: Theory
Matthew J. Sobel, Case Western Reserve University,
matthew.sobel@case.edu, Jie Ning
An MDP with continuous state and action vectors is shown to have an extremal
optimal policy if it has affine immediate rewards and dynamics, decomposable
constraints on the actions, and maximizes the expected present value of the
rewards. Identifying an optimal policy and computing its value function reduces
to solving a small system of auxiliary equations. This exorcises the curse of
dimensionality. The same structure in a sequential game yields the existence and
simple characterization of an extremal Nash equilibrium. A companion paper
with algorithms and applications is in another session.
TB41
207C-MCC
Quantitative Methods in Finance XII
Sponsored: Financial Services
Sponsored Session
Chair: Woo Chang Kim, Associate Professor, KAIST, KAIST 291,
Daehak-ro, Yuseong-gu, Daejeon, 34141, Korea, Republic of,
wkim@kaist.ac.krCo-Chair: Changle Lin, Princeton University, Jersey City, NJ, United
States,
changlel@princeton.edu1 - Robo-advisor And Personalized Asset & Liability system
Changle Lin, Merrill Lynch Wealth Management,
changlelin1@gmail.com2 - Robo-advisor: Goal-based Investing And Gamification
Paolo Sironi, IBM,
paolo.sironi@de.ibm.comThe WM industry changes from distribution of products to channel of financial
advice. Robo-Advisors need to innovate FIN not only TECH, embrace Goal Based
Investing, move beyond MPT and craft better inter-temporal understanding of
future performance of actual products, liabilities and portfolios against targets
(asset values or post-retirement income). Probabilistic Scenario Optimisation
facilitates the shift from market-oriented to client-centric allocations. Fears and
ambitions enter the new equation if investors change their behaviour:
Gamification could help to learn what money is for, how to invest and what to
believe in when setting investment goals across scenarios.
3 - Robo-advisor: Application Of Advanced Portfolio Technology
Woo Chang Kim, KAIST,
woochang.kim@gmail.com4 - Evidence-based Improvements To Investor Behavior:
Betterment’s Approach
Daniel Egan, Betterment, 61 W. 23rd Street, 4th Floor, New York,
NY, 10010, United States,
dan@betterment.comAs the largest independent robo-advisor, Betterment relies on technology to
further its mission of providing affordable, personalized advice to investors,
regardless of their account size. Dan Egan, Vice President of Behavioral Finance
and Investing at Betterment, will discuss the firm’s efforts to build features that
address behavioral biases that cause retail investors to make systematic
investment “mistakes,” including under-saving and excessive trading. He will also
discuss Betterment’s internal culture and how it contributes to a focus on
innovation and to ongoing, evidence-based behavioral improvements.
TB41