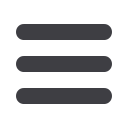

INFORMS Nashville – 2016
278
TB42
207D-MCC
Learning, Estimation, and Experimentation in
RM and Pricing
Sponsored: Revenue Management & Pricing
Sponsored Session
Chair: Stefanus Jasin, University of Michigan, Ann Arbor, MI, United
States,
sjasin@umich.edu1 - Demand Forecasting In The Presence Of Unobserved Lost Sales
Shivaram Subramanian, IBM,
subshiva@us.ibm.com,Pavithra Harsha
We present an effective mixed-integer programming (MIP) based optimization
approach to calibrating attraction demand models for pricing and revenue
management applications using censored historical sales data. This single-step
approach helps overcome some of the limitations present in prior approaches
(e.g., the EM method). We discuss its practical viability by reviewing a recent
commercial implementation for a large retail chain. We also comment on
interesting extensions.
2 - Learning Valuation Distributions From Bundle Sales
Will Ma, ORC, MIT,
willma353@gmail.com,David Simchi-Levi
Bundling has been widely studied in the literature as a form of price
discrimination. We show that it can also be used as a form of price
experimentation - a mixed bundling scheme allows the firm to quickly learn the
customer valuation distributions without having to change any prices. We present
an iterative algorithm to reverse-engineer the valuations based on bundle sales,
with theoretical convergence guarantees for Uniform distributions. For other two-
parameter families of distributions, our extensive numerical experiments
demonstrate that optimizing over the learned parameters still extracts close to
100% of the optimal profit obtainable had we known the exact parameters.
3 - Less Can Be More In Price Experimentation
Georgia Perakis, MIT,
georgiap@mit.edu, Divya Singhvi
We consider a dynamic pricing problem where a monopolist is selling a single
product but has no knowledge of the demand curve. Further, there is a cost on
price experimentation, as for every price the monopolist incurs a fixed
operational cost. The monopolist seeks to efficiently learn the demand curve and
keep the cost of price experimentation low. We propose an approach for price
experimenting and learning the demand for the problem and provide bounds on
the number of price experimentations needed to achieve a threshold revenue
level for both parametric and non-parametric demand functions. We show that
with few price experimentations (aka 4) we can be within 18% of the optimal.
4 - A Nonparametric Self-adjusting Control For Multi-product Pricing
With Limited Resources
Qi (George) Chen, Ross School of Business, University of
Michigan, Ann Arbor, MI, United States,
georgeqc@umich.edu,
Stefanus Jasin, Izak Duenyas
We study a multi-period network revenue management problem where the
underlying demand function is unknown (in the nonparametric sense) to the
seller who uses dynamic pricing to minimize expected revenue loss. It is known
that the asymptotic revenue loss of any feasible pricing policy is O(k^{1/2}) (k
indicates the size of the problem), but there is a considerable gap between this
theoretical lower bound and the performance bound of all existing heuristics. We
propose a Nonparametric Self-adjusting Control and show that it guarantees a
revenue loss of O(k^{1/2+epsilon} log k) for any arbitrarily small epsilon>0,
provided that the underlying demand function is sufficiently smooth.
TB43
208A-MCC
Panel: New Frontiers in Decision Analysis Practice
and Theory
Sponsored: Decision Analysis
Sponsored Session
Moderator: Franklyn Koch, Koch Decision Consulting, Eugene, OR,
United States,
kochfg@gmail.comModerator: Melissa A. Kenney, University of Maryland, College Park,
MD, United States,
kenney@umd.edu1 - New Frontiers In Decision Analysis Practice And Theory
Franklyn Koch, Koch Decision Consulting,
kochfg@gmail.comThis panel of Decision Analysis practitioners and academicians will discuss some
of the problems in Decision Analysis that they are struggling to solve. These
would include decisions where the existing techniques & tools fall short, areas
where practitioners are looking for new approaches & insights, and innovative
ideas and techniques that may provide new insights into difficult or complex
decisions. Panelists include: Greg Hamm, Berkeley Research Group; Babak
Jafarizadeh, Statoil; Bill Klimack, Chevron.
TB44
208B-MCC
Graphical Methods
Sponsored: Decision Analysis
Sponsored Session
Chair: Jeffrey M Keisler, University of Massachusetts - Boston,
100 Morrissey Boulevard, Boston, MA, 02125, United States,
jeff.keisler@umb.edu1 - Decision Circuits For Decision Analysis
Debarun Bhattacharjya, IBM T. J. Watson Research Center,
Yorktown Heights, NY, United States,
debarunb@us.ibm.com,
Ross D Shachter
A decision circuit is a graphical representation that is syntactic, i.e. depicts
summation, multiplication and maximization operations required to solve a
decision problem. Decision circuits can be viewed as a representation of decision
analysis computations and therefore generalize decision trees as well as other
well-known graphical forms. In this talk, I will present advances in our research
on the formulation and analysis of decision analysis problems using decision
circuits.
2 - On Computing Probabilities Of Dismissal Of 10b-5 Security
Class-action Cases
Sumanta Singha, PhD Student, University of Kansas, 1654
Naismith Dr, Lawrence, KS, 66045, United States,
sumanta.singha@ku.edu,Steve Hillmer, Prakash P Shenoy
The main goal of this paper is to propose a probability model for computing
probabilities of dismissal of 10b-5 securities class-action cases filed in U.S. Federal
district courts. By dismissal, we mean dismissal with prejudice in response to the
motion to dismiss filed by the defendants, and not eventual dismissal after the
discovery process. The proposed probability model is a hybrid of two widely-used
methods: logistic regression (LR), and naïve Bayes (NB). Using a dataset of 925
10b-5 securities class-action cases, we show that the proposed hybrid model has
the potential of computing better probabilities than either LR or NB models. By
better, we mean lower RMSE of probabilities of dismissal.
3 - Observing Reporting And Deciding In Networks
Jeffrey Keisler, University of Massachusetts Boston,
jeff.keisler@umb.edu,H Jerome Keisler
In observation networks, agents make observations, make new inferences, and
report to neighbors to ultimately identify correct alternatives. Report plans ensure
that this happens reliably. Junction tree algorithms applied to Bayes networks
constitute report plans. General conditions for existence of report plans suggest
other modeling possibilities.
TB45
209A-MCC
Parallel Simulation Optimization
Sponsored: Simulation
Sponsored Session
Chair: Jie Xu, George Mason University, 4400 University Dr., MSN 4A6,
Fairfax, VA, 22030, United States,
jxu13@gmu.edu1 - Implementing A Ranking And Selection Procedure In The Cloud
Sijia Ma, Cornell University, Ithaca, NY, United States,
sm2462@cornell.edu,Shane Henderson
The goal of ranking and selection (R&S) procedures is to identify the stochastic
system with largest mean from among a finite set of competing alternatives. We
are implementing a R&S algorithm within a commercial simulation software
product that runs in the cloud. A cloud-computing implementation requires
estimating the wall-clock running time as a function of the number of cores used.
To estimate the running time we develop a sampling and estimation method to
learn about the ordered means. This methodology allows us to predict the
residual running time more and more accurately as the R&S algorithm proceeds,
and may prove useful when estimating the running times of other R&S
procedures.
2 - Speeding Up Sequential Selection-of-the-best Procedures For
Large-scale Problems
Jeff Hong, City University of Hong Kong,
jeffhong@cityu.edu.hk,
Jun Luo, Ying Zhong
Classical sequential ranking-and-selection (R&S) procedures require all pairwise
comparisons after collecting one additional observation from each surviving
system, which is typically an O(k^2) operation where k is the number of systems.
When k is large (e.g., millions), these comparisons can be very costly and may
significantly slow down the R&S procedures. In this paper we revise KN
procedure slightly and show that one may reduce the computational complexity
of all pairwise comparisons to an O(k) operation, thus significantly reducing the
computational burden. Numerical experiments show that the computational time
reduces by orders of magnitude.
TB42