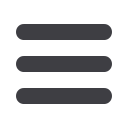

INFORMS Nashville – 2016
280
TB48
210-MCC
Optimization and Statistical Learning
Sponsored: Optimization, Optimization Under Uncertainty
Sponsored Session
Chair: Dimitri Papadimitriou, Alcatel-Lucent,
Bell-Telephonelaan, Brussels, BC, 00000, Belgium,
dimitri.papadimitriou@alcatel-lucent.comCo-Chair: Dimitri Papadimitriou, Bell Labs, Copernicuslaan 50,
Antwerp, 2018, Belgium,
dimitri.papadimitriou@nokia.com1 - Statistical Learning Approaches For Stochastic Optimization
(SLASO)
Suvrajeet Sen, USC,
s.sen@usc.edu,Yunxiao Deng
SLASO is a new distribution-free concept for Integrative Analytics, bringing
together both Predictive and Prescriptive Analytics. We will illustrate the power of
this concept by demonstrating how multiple activities, such as sales, marketing,
and production planning can all work from the same data sources, thus helping to
coordinate decisions from a variety of groups within an organization. The SLASO
framework helps cross-fertilize members of an analytics team so that tools such as
regression (Statistics), linear programming (Optimization), variance reduction
(Simulation) and others can be viewed from an integrative perspective, rather
than the current lens of disciplinary stove-pipes.
2 - Time Series Applications Of SLASO
Yunxiao Deng, USC,
yunxiaod@usc.eduWe will introduce how SLASO can be used for Time Series Applications by
studying a single echelon inventory model in which the demand data are time
dependent and stochastic. To minimize the expected cost of holding inventory
plus lost sales, we formulate a Stochastic Linear Programming model which
combines statistics as well as optimization by adopting SLASO framework. With
other two methods (Newsvendor and Demand Forecasting), this approach
performs better in both Back-Testing and Stress-Testing during validation analysis.
3 - Bayes-optimal Entropy Minimization For Active Learning In
Conjoint Analysis
Stephen N Pallone, Cornell University, 290 Rhodes Hall, Cornell
University, Ithaca, NY, 14853, United States,
snp32@cornell.edu,Peter Frazier, Shane Henderson
Choice-based conjoint analysis is a method for preference elicitation where the
user is offered a set of alternatives and chooses the preferred option. The rate at
which we learn depends on the alternatives offered. We model the user’s
preferences through a linear classifier. Under certain noise assumptions, we prove
a linear lower bound on the posterior entropy of this linear classifier, and show
entropy pursuit can attain the bound when alternatives can be fabricated.
Further, we explore an information theoretic variant of the knowledge gradient
policy that selects comparative questions to greedily minimize interaction
information, and numerically compare this policy with entropy pursuit.
4 - Learning Uncertainty Sets And Automation Of Robust Formulation
Dimitri Papadimitriou, Bell Labs,
dimitri.papadimitriou@nokia.comMachine learning shares deep connections with robust optimization as they both
perform by adding uncertainty in the model, formulating the optimization
problem, and exploiting mathematical programming. Machine learning applies to
i) model uncertainty by automating processing of noisy or aleatory data to
produce perturbation sets, ii) automatically derive robust formulation but also
adapt decision rules associated to adjustable variables, and iii) learn about the
behavior of resolution algorithm(s) and tune its execution to improve its
performance. We illustrate them on three network optimization problems, the
multi-commodity network flow, facility location and hub location problem.
TB49
211-MCC
Educational Research in OR/MS
Sponsored: Education (INFORMED)
Sponsored Session
Chair: Susan Wright Palocsay, James Madison University,
Harrisonburg, VA, United States,
palocssw@jmu.edu1 - Batching In Higher Education
Jan Riezebos, University of Groningen, Nettelbosje 2, P.O. Box
800, Groningen, 9700 AV, Netherlands,
j.riezebos@rug.nl,Iris F Vis
Models for optimal batch sizes in industrial context, such as economic lot sizing,
cannot directly be applied to the context of higher education. However, in higher
education, batching is even more important than in industrial contexts, as it is not
just the economical impact that has to be considered, but also various effects on
learning. We explore possible ways to extend OR models for batching in higher
education.
2 - Effective Teaching For Mastery Of Boolean Constraints
Scott Stevens, Professor, CIS & Business Analytics, James Madison
University, James Madison University, MSC 202, Harrisonburg, VA,
22807, United States,
stevensp@jmu.edu, Susan Wright Palocsay
Mixed integer optimization problems often include constraints involving Boolean
variables—variables that can equal only 0 or 1—representing the truth values of
some logical propositions. The pure compound propositions of either “all Ai are
true” or “at least one Ai is true” for some collection of Boolean variables Ai are
easy to represent as linear expressions, but students frequently find propositions
of the form (pure compound 1) implies (pure compound 2) to be baffling. We
introduce a technique that allows any such proposition to be written as one or
more linear constraints by applying three simple rules: decomposition,
translation, and compression, and then provide evidence of efficacy.
3 - A Review Of The Literature For Operations
Management Education
Susan Wright Palocsay, James Madison University, Harrisonburg,
VA, United States,
palocssw@jmu.edu, Michael Busing
Operations management (OM) has maintained a close association with OR/MS as
its scope has broadened from manufacturing to service processes. In response, OM
curricula have undergone significant revision with a corresponding increase in
OM pedagogical studies. We will present a summary of OM educational
publications and discuss OM teaching trends.
TB50
212-MCC
Panel: Topics for PhD Students
Sponsored: Minority Issues
Sponsored Session
Moderator: Maria Esther Mayorga, North Carolina State University, 111
Lampe Drive, Campus Box 7906, Raleigh, NC, 27695, United States,
memayorg@ncsu.edu1 - Topics For Phd Students
Maria Esther Mayorga, North Carolina State University,
memayorg@ncsu.eduThis session will serve as a panel discussion on topics of interest for PhD students
nearing graduation. Topics include: - deciding on industry versus academia - how
to prioritize objectives towards then end of the PhD Process - work/life balance
when pursuing tenure - networking to achieve a desired faculty position - how to
position yourself when pursuing the market - networking at conferences such as
INFORMS
2 - Panelist
Maria Esther Mayorga, North Carolina State University,
memayorg@ncsu.edu3 - Panelist
Karen T Hicklin, University of North Carolina at Chapel Hill,
Chapel Hill, NC, 27599, United States,
khicklin@email.unc.eduTB51
213-MCC
Matchings and Assignments with Societal Impact
Sponsored: Public Sector OR
Sponsored Session
Chair: Tina Rezvanian, Northeastern University, 360 Huntington
Avenue, Boston, MA, 02115, United States,
rezvanian.t@husky.neu.eduCo-Chair: Ozlem Ergun, Northeastern University, 453 Meserve,
360 Huntington Avenue, 360 Huntington Avenue, MA, 02115,
United States,
o.ergun@neu.edu1 - Two-sided Stable Matching In Markets With Multiple Periods
Tina Rezvanian, Northeastern University, Boston, MA,
United States,
rezvanian.t@husky.neu.edu,Ozlem Ergun
Many industries demand mechanisms for job assignments that are sustainable
over multiple periods of time. It is a well-known fact that the core stays the same
under any stable matching algorithm and therefore no such matching can
guarantee sustainability and perfectness as a dominant strategy for participants as
same participants stay unsatisfied. We introduce a popularity measure that
identifies a connection between stability of the match and fairness for those
unsatisfied. We use this trade off to employ negotiation schemes in our proposed
algorithm. By analyzing network structures of the large-scale markets we are able
to acquire near-optimal solutions.
TB48