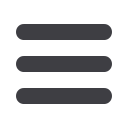

INFORMS Nashville – 2016
285
TB63
Cumberland 5- Omni
Deterministic Network Design
Sponsored: TSL, Freight Transportation & Logistics
Sponsored Session
Chair: Mike Hewitt, Loyola University Chicago, NA, Chicago, IL, 60611,
United States,
mhewitt3@luc.edu1 - Barge Scheduled Service Network Design With Resource And
Revenue Management
Teodor Gabriel Crainic, Universite du Quebec a Montreal,
TeodorGabriel.Crainic@cirrelt.net, Ioana Bilegan, Yunfei Wang
We study the incorporation of revenue management (RM) considerations, usually
tackled at the operational-planning level, into tactical planning models for
consolidation-based freight transportation carriers, and the impact of this
integration on the selection of customer to service and on the structure of the
service network, e.g., should the carrier increase the offer of service through more
departures or larger vessels in order to later be able to capture spot demand? We
present a service network design model with resource and RM considerations, a
meta-heuristic, and the experimental results and insights obtained in the context
of intermodal barge transportation.
2 - The Value Of Flexibility In Long-haul Transportation
Network Design
Mike Hewitt, Loyola University Chicago,
mhewitt3@luc.edu,
Natashia Boland, Martin W P Savelsbergh
Freight transportation carriers are facing increased demands from customers for
shorter service standards. At the same time, some cusotmers are flexible in terms
of when they want their shipments delivered, and will accept longer delivery
times if given a discount. In this talk we present a model that will not only design
a long-haul transportation network, but will do so while also determining which
customers to offer a discount to in order to have more time for delivery. We
present a solution approach for the model and the results of an extensive
computational study.
3 - Decomposition Methods For Multi-period Network
Design Problems
Ioannis Fragkos, Rotterdam School of Management,
fragkos@rsm.nl,Jean-Francois Cordeau, Raf Jans
We devise decomposition methods to solve large-scale instances of multi-period
network expansion problems. For capacitated networks, we devise a custom
heuristic procedure combined with arc-based Lagrange relaxation. For
uncapacitated networks, we employ Bender’s decomposition, where the
subproblems are decomposable per period and per commodity. We formulate the
problem of generating Pareto Optimal cuts, and based on structural properties of
optimal solutions we devise a heuristic approach to solve it, thereby improving
the original Benders cuts. Computational results demonstrate the efficiency of this
approach.
4 - New Lagrangian Relaxation For Multicommodity Capacitated
Network Design
Mohammad Rahim Akhavan, Universite de Montreal (DIRO),
Monrteal, QC, Canada,
Akhavanm@iro.umontreal.ca,Teodor Gabriel Crainic, Bernard Gendron
The usual Lagrangian relaxations for multicommodity capacitated network design
are the so-called shortest path and knapsack relaxations, which are obtained,
respectively, by relaxing linking constraints and flow conservation equations. We
present a new reformulation and Lagrangian relaxation for the problem. We
show that the Lagrangian dual bound improves upon the so-called strong LP
bound (known to be equal to the Lagrangian dual bounds of the shortest path
and knapsack relaxations).
TB64
Cumberland 6- Omni
Multi-objective Optimization: Algorithms
and Applications
Sponsored: Multiple Criteria Decision Making
Sponsored Session
Chair: Lakmali Weerasena, University of Tennessee, College Dr,
Chattanooga, TN, 31705, United States,
lweeras@g.clemson.edu1 - New Multicriteria Models For Robust Data Classification In
Supervised Learning
Alexander Engau, University of Colorado Denver,
alexander.engau@ucdenver.eduData classification is a key task for predictive analytics, data mining and
supervised machine or statistical learning. In extension of its current state-of-the-
art optimization approaches this presentation highlights several new multicriteria
mixed-integer goal programming models that can further improve their
performance for classification and prediction by combining a variety of different
objectives including solution accuracy as well as total and minimum or maximum
internal or external deviation. Computational experiments on financial and
medical data sets are reported and demonstrate promising results with highly
improved robustness and better classification accuracy overall.
2 - Utility Indifference Pricing Under Incomplete Preferences Via
Vector Optimization
Firdevs Ulus, Bilkent University,
firdevs@bilkent.edu.trUnder some assumptions on an incomplete preference relation, utility
maximization problem is a convex vector optimization problem. Accordingly, the
utility buy and sell prices are defined as set valued functions of the claim. It has
been shown that the buy and the sell prices recover the complete preference case
where the utility function is univariate. Moreover, buy and sell prices satisfy some
monotonicity and convexity properties as expected. It is possible to compute these
set valued prices by solving convex vector optimization problems.
3 - Local Branching Algorithm For Approximating The Pareto Set Of
The Multiobjective Set Covering Problem
Lakmali Weerasena, University of Tennessee at Chattanooga,
Chattanooga, TN, United States,
lweeras@g.clemson.eduThe multiobjective set covering problem (MOSCP), a challenging combinatorial
optimization problem, has received limited attention in the literature. We present
an algorithm to approximate the Pareto set of the MOSCP. The proposed
algorithm applies a local branching approach on a tree structure and is enhanced
with a node exploration strategy specially developed for the MOSCP. The key idea
is to partition the search region into subregions based on the neighbors of a
reference solution. Numerical experiments confirmed that the proposed algorithm
performs well on the MOSCP. Results on a performance comparison with
benchmark algorithms from the literature show that the new algorithm is
competitive.
4 - Interactive Weight Region-based Approach For Multiobjective
Optimization Problems
Mehmet Basdere, Northwestern University, Evanston, IL,
United States,
mehmetbasdere2016@u.northwestern.edu,
Sanjay Mehrotra, Karen Smilowitz
We introduce an interactive weight region-based approach that can iteratively
find the most preferred solution of a decision maker (DM) after exploring a small
fraction of all nondominated solutions. To obtain preference information, the DM
is given a series of questions to compare and these comparisons define constraints
restricting the weight region. New solutions are obtained by using diverse weight
vectors generated from the remaining weight region via a mixed integer
programming formulation. We develop two finitely converging algorithms for
multi-objective linear and integer programs respectively. The results show that
the algorithms terminate after a reasonable number of iterations.
TB65
Mockingbird 1- Omni
Digital Business Models and Strategies in the
Era of Analytics
Sponsored: Information Systems
Sponsored Session
Chair: Ling Xue, Georgia State University, Georgia State University,
Atlanta, GA, 30302, United States,
lxue5@gsu.edu1 - Relationships Between Online Daily Deal Promotions And Local
Retailers’ Online Reputation
Gang Wang, University of Delaware,
gangw@udel.eduOnline daily deal sites such as Groupon have recently provided an innovative
marketing tool for local retailers. On the one hand, a local retailer’s online
reputation is an important fact that may impact its decision whether to run a
promotion. On the other hand, an online daily deal promotion may also impact
the local retailer’s online reputation. In this study, we study the causal
relationships between a local retailer’s promotion decision and its online
reputation using data collected from Groupon and Yelp. Our current results
enhance understanding of local retailers’ Groupon promotion decisions and yield
important implications related to daily deal sites.
TB65