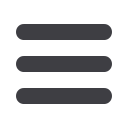

INFORMS Nashville – 2016
30
SA38
3 - Inventory Management In An Omnichannel Environment
Yong-Pin Zhou, Professor, Foster School of Business, University of
Washington, Seattle, Seattle, WA, 98195-3226, United States,
yongpin@uw.edu, Elnaz Jalilipour Alishah
We consider a single newsvendor-type product that is sold both online and
offline. We present two models where each channel is used as a backup for the
other channel, and derive structural and qualitative results on effective inventory
management policies. Specifically, we consider inventory positioning, inventory
level, and real-time inventory rationing decisions. When it is possible to shift
some customer demand using discounts, we also investigate the level of discount
and customer reaction.
4 - The Omni-channel Fulfillment Dilemma
Santiago Gallino, Dartmouth College, Dartmouth, NH,
United States,
santiago.gallino@tuck.dartmouth.edu,
Antonio Moreno-Garcia, Robert P. Rooderkerk
Using transaction level data from a multi-channel retailer we explore how
customer interact with the retailer over time. We study the underlying patters of
customer’s interactions and discuss the implications for retailers that have both an
online and a brick and mortar presence.
SA38
206A-MCC
Product Development and Competition
Invited: New Product Development
Invited Session
Chair: Morvarid Rahmani, Georgia Institute of Technology, Atlanta,
Atlanta, GA, 30308, United States,
morvarid.rahmani@scheller.gatech.eduCo-Chair: Karthik Ramachandran, Georgia Institute of Technology,
Atlanta, GA, 30308, United States,
karthik.ramachandran@scheller.gatech.edu1 - Knowledge Search In Mobile App Development
Nilam Kaushik, PhD Candidate, UCL School of Management,
London, United Kingdom,
uceikau@ucl.ac.uk,Bilal Gokpinar
The process of search, identification, and acquisition of new knowledge is
essential for the success of new products. We explore how firms search for ideas
in sequential product development through the highly competitive and dynamic
setting of mobile application development. Using novel text-mining techniques,
we derive measures of similarity between a focal app’s update and updates made
by competitor apps and study performance implications thereof. We also explore
the performance implications of the distance of an app’s update with respect to its
past updates.
2 - Decision Options At Project Gate Reviews:
Beyond The Go/ Kill Model
Alison Olechowski, Massachusetts Institute of Technology,
Cambridge, MA, United States,
alisono@mit.eduSteven D Eppinger, Nitin Joglekar
Most current academic models of project gate reviews represent the gate decision
as a simple choice between go and kill. In reality, product developers often reach
the gate with incomplete or unacceptable deliverables, and managers consider
more than just the kill option if a go is not appropriate. We have created a simple
model which adds options of: waiver, waiver with re-review, delay and switch to
back-up plan. This decision tree model compares the value of this more realistic
set of options based on costs, payoffs and confidences. We demonstrate the
application of this model to complex product development gate decisions via
multiple case examples from industry.
3 - Capacity Investment For Product Upgrades Under Competition
Ram Bala, Santa Clara University,
ram.bala@gmail.com,
Milind Sohoni, Sumit Kunnumkal
Firms often introduce a vertical line extension of an existing product to
consolidate their market position after loss of monopoly status. However,
introducing a line extension is fraught with uncertainty as it may fail to be
technically feasible as originally intended. We analyze a two stage competitive
game between an incumbent and an entrant where the firms make capacity
investment decisions before uncertainty resolution and then set production
quantities. We uncover conditions on innovation level which determine whether
the incumbent will continue to offer the existing product once the new product
succeeds. We also determine the innovation level beyond which competitive
entry is deterred.
4 - Institutional Design And The Creative Process:
An Experimental Study
Lakshminarayana Nittala, University of California San Diego,
La Jolla, CA, 92037, United States,
lnittala@ucsd.eduSanjiv Erat, Viswanathan Krishnan
The process of Innovation often takes the form of problem solving and requires
creative insights for achieving success. In an experimental setting we use tasks
that are representative of such problems and study the role of institutional design
on the creative output and the underlying search process.
SA39
207A-MCC
A/B Testing, Experiments, and Learning
Sponsored: Applied Probability
Sponsored Session
Chair: Ramesh Johari, Stanford University, Stanford, CA, United States,
ramesh.johari@stanford.eduCo-Chair: David Walsh, Stanford University, Department of Statistics,
Stanford, CA, 94305, United States,
dwalsh@stanford.edu1 - Using Simulation To Improve Statistical Power In Switchback
Experiments At Uber
Peter Frazier, Cornell University, Ithaca, NY, 14850, United States,
pf98@cornell.eduWe consider A/B testing of systemic changes with time-varying effects, such as
changes to the algorithm used to dispatch cars at Uber. Testing such changes is
made difficult by correlations in outcomes across dispatches, and by seasonal and
autocorrelated random variation in riders’ demand for trips. One standard A/B
testing method is a switchback experiment, which applies the treatment and
control on alternating days over two weeks. We show how to combine
simulation-based predictions of a change’s effects with data from a switchback
experiment to improve statistical power, and make analysis robust to missing
data.
2 - A/B Testing In A Changing World
David Walsh, Stanford University,
dwalsh@stanford.eduThe purpose of A/B testing is to let any technology company iterate on its
products quickly and stay ahead of a rapidly changing market. Given this dynamic
context, it is odd that the existing statistical approaches to A/B testing view the
environment as static. The outcome: inferences that do not generalize beyond the
life of the experiment, which then lead to actions that perform substantially
worse than expected. I present new methodology that anticipates temporal
variation, generating the right inferences to support dynamic product
optimization.
3 - Simple Bayesian Algorithms For Identifying The Best Arm In A
Multi-armed Bandit
Daniel Russo, Northwestern University, Evanston, IL, United
States,
Dan.Joseph.Russo@gmail.comThis talk considers the optimal adaptive allocation of measurement effort for
identifying the best among a finite set of options or designs. An experimenter
sequentially chooses designs to measure and observes noisy signals of their
quality with the goal of confidently identifying the best design after a small
number of measurements. I propose three simple Bayesian algorithms for
adaptively allocating measurement effort. Each is shown to have strong
performance in numerical experiments, and a unified analysis establishes each
satisfies a strong asymptotic optimality property.
4 - Sequential A-B Testing With Constraints
Vivek Farias, Massachusetts Institute of Technology, Cambridge,
MA, United States,
vivekf@mit.edu,Ciamac Cyrus Moallemi
We consider the problem of sequential A-B testing when the impact of a
treatment is marred by a large number of covariates. Our main contribution is a
tractable algorithm for the online allocation of test subjects to either treatment
with the goal of maximizing the efficiency of the estimates treatment effect under
a linear model, which due to a surprising state space collapse, reduces to solving a
low dimensional dynamic program. Our approach is robust and covers many
variations of the problem, including cases where there are budget constraints on
individual treatments, where the number of trials is to be endogenously decided,
and where the objective is to balance a tradeoff between efficiency and bias.