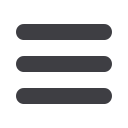

INFORMS Philadelphia – 2015
333
TC43
43-Room 103A, CC
Joint Session RMP/MSOM: Choice Models:
Estimation and Optimization
Sponsor: Revenue Management and Pricing
Sponsored Session
Chair: Sumit Kunnumkal, Indian School of Business, Gachibowli,
Hyderabad, India,
Sumit_Kunnumkal@isb.edu1 - Formulation, Motivation, and Estimation for the D-Level Nested
Logit Model
Guang Li, University of Southern California, Bridge Hall 401,
None, Los Angeles, CA, 90089-0809, United States of America,
guangli@usc.edu,Huseyin Topaloglu, Paat Rusmevichientong
Using a tree of depth d, we provide a novel formulation for the d-level nested
logit model. Our model is consistent with the random utility maximization
principle and equivalent to the elimination by aspects model. Using new
concavity results on the log-likelihood function, we develop an effective
parameter estimation algorithm. Numerical results show that the prediction
accuracy of the d-level nested logit model can be substantially improved by
increasing the number of levels d in the tree.
2 - Assortment Optimization Over Time
James Davis, Cornell University, 290 Rhodes Hall, Ithaca, NY,
United States of America,
jamesmariodavis@gmail.com,
Huseyin Topaloglu, David Williamson
Inspired by online retail we introduce a new type type of assortment optimization
problem: assortment optimization over time. In this problem the retailer must
choose which products to display but must also choose an ordering for the
products. This is a relevant problem when items are displayed as a list; this is
common when returning results from a search query, for example. We provide a
framework to analyze this problem, provide an approximation algorithm, and
some hardness results.
3 - Tractable Bounds for Assortment Planning with Product Costs
Sumit Kunnumkal, Indian School of Business, Gachibowli,
Hyderabad, India,
Sumit_Kunnumkal@isb.edu,
Victor Martínez-de-Albéniz
Assortment planning under a logit demand model is a difficult problem when
there are product specific costs associated with including products into the
assortment. In this paper, we describe a tractable method to obtain an upper
bound on the optimal expected profit. We provide performance guarantees on the
upper bound obtained. We describe how the method can be extended to
incorporate additional constraints on the assortment or multiple customer
segments.
4 - Clustering Consumers Based on Their Preferences
Ashwin Venkataraman, New York University,
715 Broadway, New York, NY, United States of America,
ashwin.venkataraman@gmail.com, Srikanth Jagabathula,
Lakshminarayana Subramanian
Preference-based clustering is an important and challenging problem. We propose
a non-parametric method to cluster consumers based on their preferences for a
set of items. Our method combines the versatility of model-free clustering (such
as k-means) with the flexibility and rigor of model-based clustering (based on EM
algorithm). Our approach is fast, can handle missing data, identify general
correlation patterns in consumer preferences, and has provable guarantees under
reasonable assumptions.
TC44
44-Room 103B, CC
Pricing in Online Markets
Sponsor: Revenue Management and Pricing
Sponsored Session
Chair: Kostas Bimpikis, Stanford GSB, 655 Knight Way, Stanford, CA,
94305, United States of America,
kostasb@stanford.edu1 - Modeling Growth for Services: Evidence from the App Economy
Ken Moon, PhD Candidate, Stanford GSB, 655 Knight Way,
Stanford, CA, 94305, United States of America,
kenmoon@stanford.edu,Haim Mendelson
We present a model of service operations to grow and sustain customers by the
operational design and performance of services, rather than marketing alone.
Applying our framework to data from services in the app economy, we show (i)
that customers’ engagement contributes as powerfully to growth as virality; and
(ii) evidence of an experience curve (from customer interactions) for service
operations. We present a model of incentive-compatible pricing for this setting.
2 - Mobile Technology in Retail: The Value of
Location-based Information
Marcel Goic, Assistant Professor Or Marketing, University of
Chile, Republica #701, Santiago, 8370438, Chile,
mgoic@dii.uchile.cl, Jose Guajardo
We analyze the value of location-based information in mobile retailing and the
conditions under which incorporating geolocation information increase
effectiveness metrics for retailers.
3 - Dynamic Pricing in Ride-Sharing Platforms
Siddhartha Banerjee, Postdoc, Stanford University, 475 Via
Ortega, Stanford, CA, 94305, United States of America,
sidb@stanford.edu, Carlos Riquelme, Ramesh Johari
We develop a model for ride-share platforms, which combines a queueing model
for the platform dynamics with strategic models for passenger and driver
behavior. Using this, we study various aspects of this system - the value of
dynamic pricing versus static pricing; the robustness of these policies; the effect of
heterogenous ride-request rates and traffic between different locations. Joint
work with Ramesh Johari, Carlos Riquelme and the Data Science team at Lyft.
4 - Pricing with Limited Knowledge of Demand
Maxime Cohen, MIT, 70 Pacific Street, Apt. 737B, Cambridge,
MA, 02139, United States of America,
maxcohen@mit.edu,
Georgia Perakis, Robert Pindyck
How should a firm price a new product with limited information on demand? We
propose a simple pricing rule that can be used if the firm’s marginal cost is
constant: the firm estimates the maximum price it can charge and then sets price
as if demand were linear. We develop bounds that show that if the true demand is
one of many commonly used demand functions, the firm will do “very well” - its
profit will be close to what it would earn if it knew the true demand.
TC45
45-Room 103C, CC
Behavioral Issues in RM
Sponsor: Revenue Management and Pricing
Sponsored Session
Chair: Anton Ovchinnikov, Queen’s University, 143 Union Str West,
Kingston, Canada,
anton.ovchinnikov@queensu.ca1 - Should Consumers be Strategic?
Arian Aflaki, Doctoral Student, Duke University, 100 Fuqua
Drive, Box 90120, Durham, NC, 27708, United States of America,
arian.aflaki@duke.edu, Robert Swinney, Pnina Feldman
We consider whether strategic consumer behavior benefits consumers when they
purchase from a rational, revenue-maximizing firm that sets prices over multiple
periods. We show that strategic behavior does not benefit all consumers. Then, by
studying a wide range of pricing and inventory strategies in a unified setting, we
find that different strategies may induce different levels of interest in strategic
behavior.
2 - Intertemporal Pricing under Minimax Regret
Ying Liu, Stern School of Business, New York University, 44 West
4th Street, KMC 8-154, New York, NY, 10012, United States of
America,
yliu2@stern.nyu.edu, Rene Caldentey, Ilan Lobel
We consider a monopolist selling a product to a population of consumers who are
heterogeneous in valuations and arrival times. We study the policies that attain
minimum regret when selling to either myopic or strategic customers. We
characterize the set of optimal policies and demonstrate their structural
properties.
3 - Behavioral Anomalies in Consumer Wait-or-Buy Decisions and the
Implications for Markdown Management
Nikolay Osadchiy, Emory University,
1300 Clifton Rd NE, Atlanta, GA, 30322, United States of
America,
nikolay.osadchiy@emory.edu,Anton Ovchinnikov,
Manel Baucells
A decision to buy at a tag price or wait for a possible markdown involves a trade-
off between the value, delay, risk and markdown magnitude. We build an
axiomatic framework that accounts for three well-known behavioral anomalies
along these dimensions and produces a parsimonious generalization of discounted
expected utility. We consider a pricing/purchasing game and show that
accounting for the behavioral anomalies results in substantially larger markdowns
and leads to noticeable revenue gains.
TC45